Below you will find some examples where we have annotated the canvas to perform a benefit-risk analysis for some digital solutions.
While these examples can serve as inspiration, keep in mind that the process is more important than the result when using the canvas. Not only is there no single “solution” to ethical risk analysis, but it’s also preferable to be pessimistic in your assessment, as it’s safer to anticipate risks that turn out not to exist than to ignore risks that do.
A synchronous chatroom for education – the canvas at design time
In this example, we consider creating an online web application that would allow students to ask and answer questions synchronously in class. We use the canvas at a very early stage of design to be able to take into account the ethical risks when thinking about the features of our solution.
Here is the color code we have used:
- Green: benefits
- Yellow: ethical risks
- Blue: mitigation options that developers can implement
- Purple: mitigation options that users can implement
- Grey: factual information
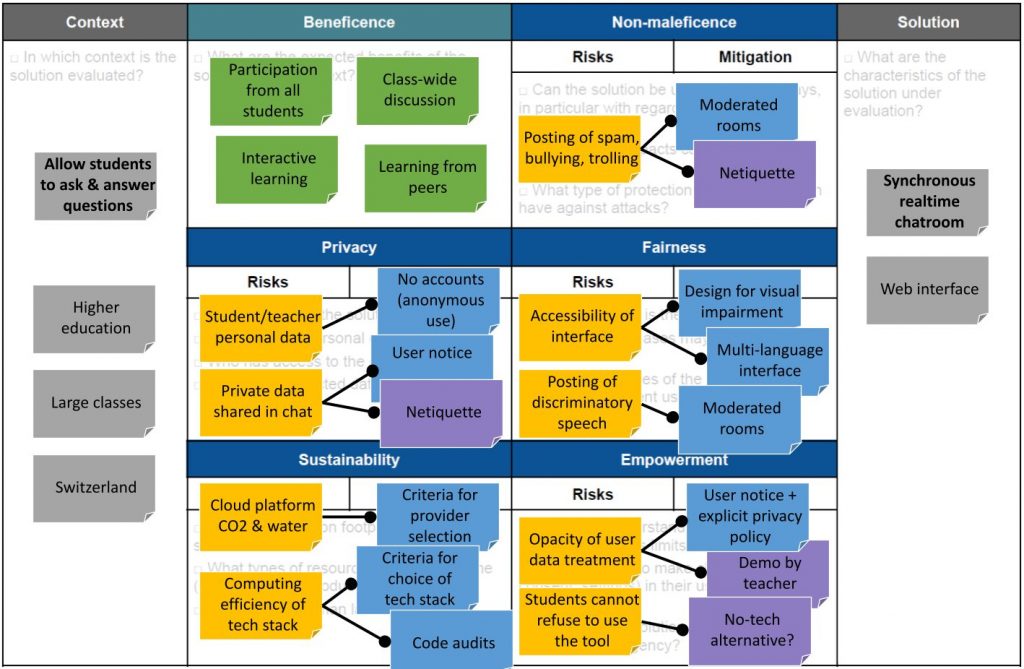
This type of analysis at an early stage can really help identify how some features or some implementation choices can significantly reduce the potential for negative impact of the solution.
Predicting user emotions – the canvas in Machine Learning
In this example we consider developing a machine learning (ML) model to predict user emotions from smartphone touch data and want to evaluate the potential ethical risks beforehand.
One difficulty when evaluating risks for ML models is that you have to imagine the many different ways in which they could be used first. So let’s imagine this emotion prediction model could be embedded into a social media platform and used in three different ways: for internal functionalities (e.g. content recommendation and moderation), to enrich the user interface for end-users and as a service to third parties-users (e.g. ad providers).
Here is the color code we have used:
- Green: benefits
- Yellow to red: medium to severe risks
- Grey: factual information
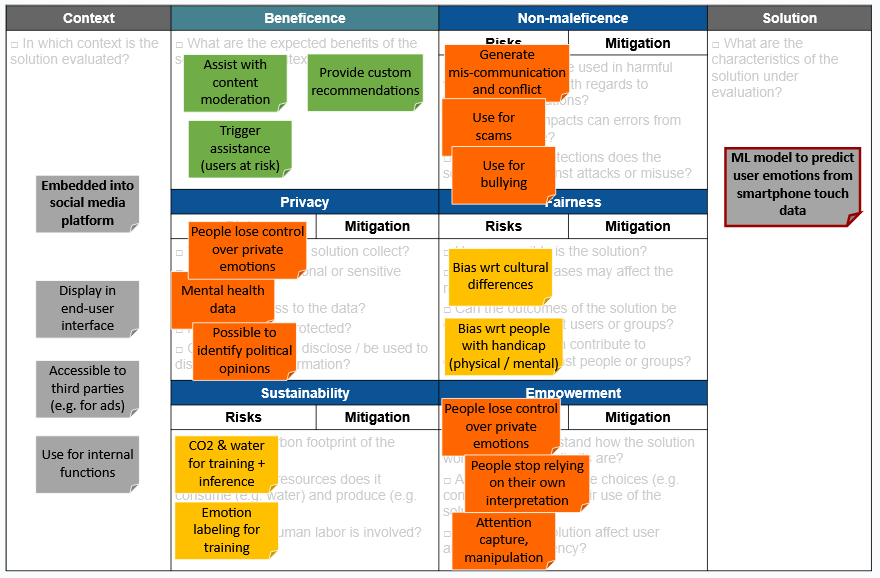
The evaluation above highlights a noteworthy amount of severe risks. Beyond the different types of use we have imagined, this would tend to indicate that developing such a model would not be a great idea.
Actually, research shows that the application of data analysis and machine learning to human emotions is a highly controversial domain generating a number of ethical and legal challenges. While it is unclear whether emotion data are protected under GDPR, other risks currently identified in emotion recognition/prediction systems are their intrusiveness, their lack of scientific grounding and the threats they generate towards human rights. This is why a ban on emotion recognition/prediction systems is under consideration in the EU AI Act.
For a complement of information, here are some additional resources:
- Hauselmann, Andreas, Alan M. Sears, Lex Zard, and Eduard Fosch-Villaronga. 2023. “EU Law and Emotion Data.” http://arxiv.org/abs/2309.10776.
- Gremsl, Thomas, and Elisabeth Hödl. 2022. “Emotional AI: Legal and Ethical Challenges.” Information Polity 27(2): 163–74. https://content.iospress.com/articles/information-polity/ip211529.
- Davis, Nicola. April 2021. “Scientists Create Online Games to Show Risks of AI Emotion Recognition.” The Guardian. https://www.theguardian.com/technology/2021/apr/04/online-games-ai-emotion-recognition-emojify.
ChatGPT for information search – the canvas at use time
The image below shows the result of the ethical risk analysis of using ChatGPT for information search (e.g. instead of a classic web search).
Here is the color code we have used:
- Green: benefits or mitigation options
- Yellow to red: medium to severe risks
- Grey: factual information
One specificity of this example is that it focuses on the risks at use time. As a result, very few mitigation options are available. When evaluating the risks at design time, there are generally many more possibilities to reduce the risks!
Another point to keep in mind is the importance of the context: using ChatGPT for other use cases (e.g. as help for brainstorming) may raise different types of risks.
If you want to have more details about this analysis and the research on which it is based, you can have a look at the slides of our “Ethics of Generative AI for Education” workshop.
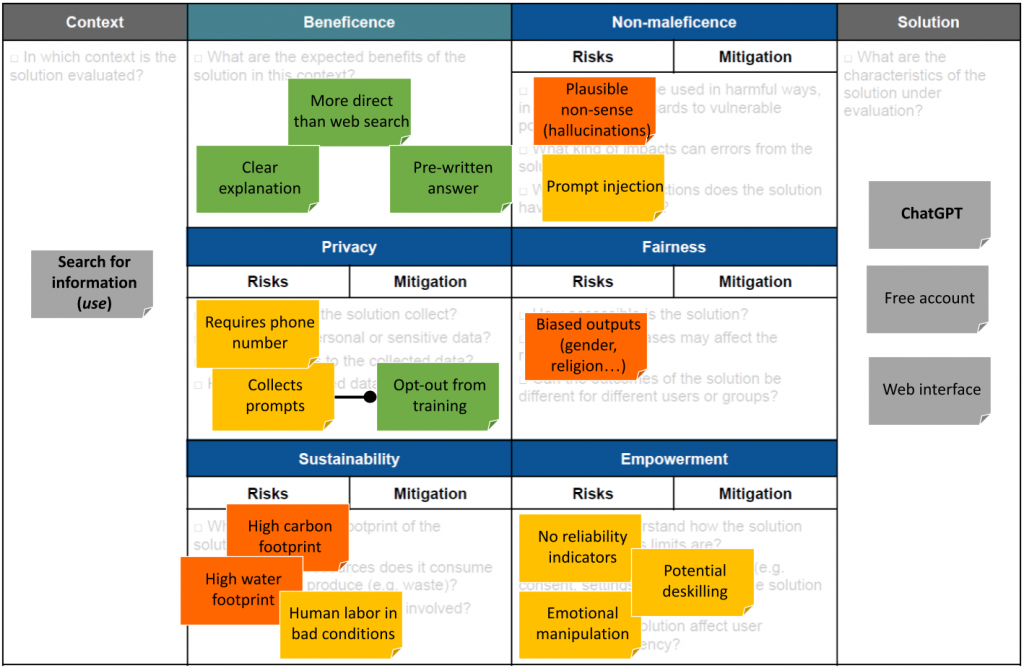