We develop new theory and methods that can be used to answer real-life questions. In practice, researchers and decision makers are often interested in causal effects: they want to learn what works. For example, will a new medication lead to better survival? Can a new teaching strategy lead to better exam scores? Should we implement a new policy to improve the economy?
However, making valid (causal) inference from data is rarely trivial. Indeed, we strongly believe that a formal framework is crucial to avoid sloppy reasoning and pitfalls. Thus, one of our main goals is to create new theory and methods for causal inference. Crucially, we strive to develop methods that rely on assumptions that are (i) transparent, (ii) scientifically testable and (iii) as weak as possible.
More specifically, several of our projects concern non-parametric identification of causal parameters in interventionist frameworks for causal inference. We are particularly interested in longitudinal data structures, where exposures, effects and outcomes are functions of time. For example, we derive results on time-to-event outcomes, such as survival, which are ubiquitous in practice. We are also developing generic estimators, where we use theory for ordinary and stochastic differential equations to obtain asymptotic guarantees. These estimators can be used to study a wide-range of causal and non-causal parameters.
Besides our methodological work, we are interested in applying new methods to available data. While most of our current applications concern clinical medicine and epidemiology, we are also interested in problems arising in biology, psychology, economics and engineering.
Pål Christie Ryalen – post-doctoral Researcher
Causal inference in recurrent events settings
My research interest is in developing causal inference methodology for survival and event history analysis. Main problems of interest include identification of causal parameters, developing software, and asymptotics of estimators; consistency and limiting distributions. Currently I am working on causal inference problems in recurrent events. In this project I will work on formulating and identifying parameters that quantify treatment effects in this setting.
Matias Janvin – Doctoral Assistant
I am interested in causal inference in settings where the outcome of interest is an event history. Currently, I am working on causal parameters for recurrent outcomes. A central aim of our work is to develop new methods to target questions of clinical interest.
Aaron Sarvet – Postdoctoral Researcher
My research focuses on extending methods for causal inference to support policy, decision-making, and mechanistic research in settings with complex social features. Such features including resource limitations and more generalised forms of connections between units. I am also interested in understanding social histories of causal inference thinking in statistics and clinical medicine.
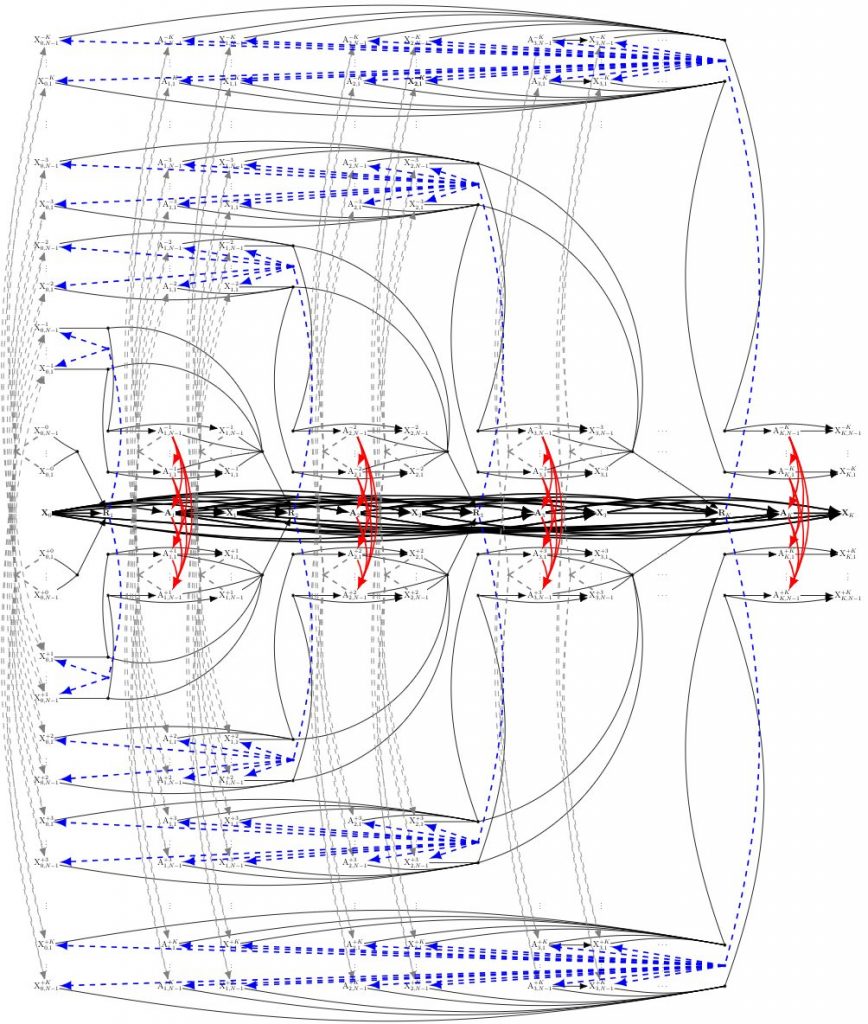