Learning Analytics
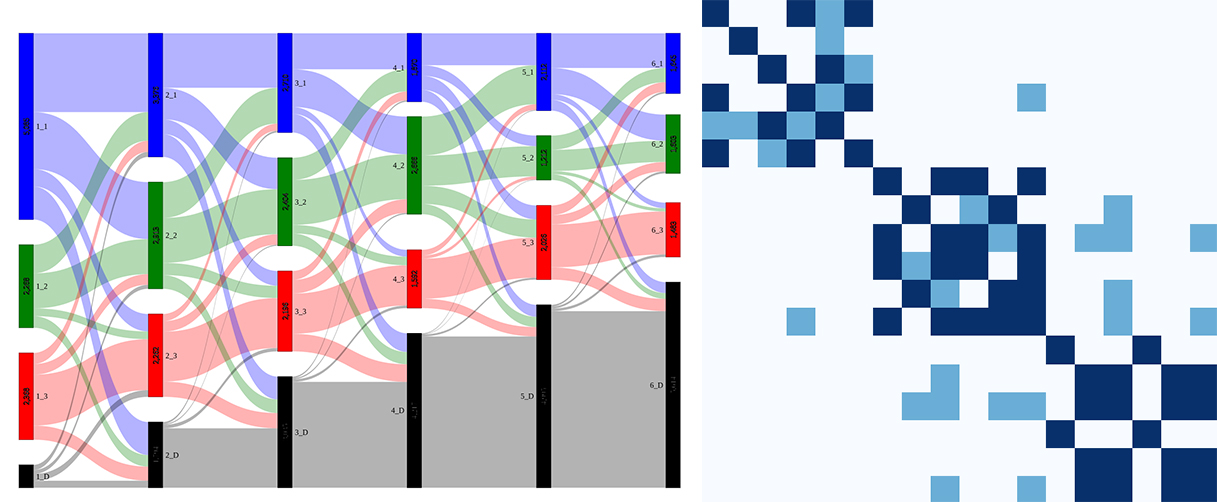
As learning technologies develop and are using more and more digital technology, new opportunities emerge. In particular, Learning Analytics, which is concerned with analyzing the processes of learning benefits greatly from this transformation. The first benefit is obviously the quantity of data that learning environment are now able to collect. From MOOCs, EPFL courses alone have generated data about more than a million of students. The technology is also inviting itself in the classrooms and universities which collects amounts of learning data un seen few decades ago. Such information can be analysed instantaneously and reported to the teacher who can then better evaluate and adapt to their students.
A second benefit is the quality of analysis tools. With the development of machine learning techniques, the modeling of student behavior and learning process has seen a lot of progress. This allows for building personalized learning environments or to intervene early for students predicted to need more attention.
Current Projects
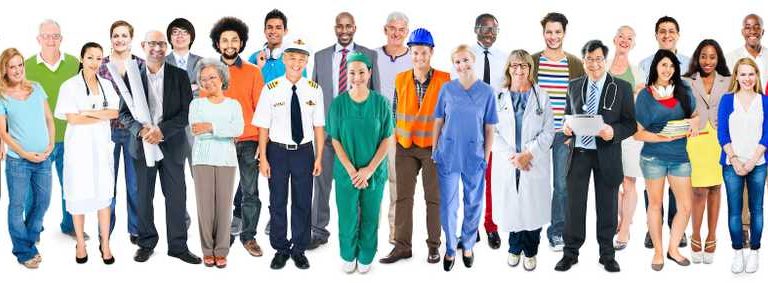
Detecting latent training needs from digital traces
It is a well-known fact that many professions, especially nowadays, undergo rapid changes to the skill sets required for them.
Previous Projects
We consider the problem where a teacher has to deliver the same sequence of examples to a diverse group of students. Their diversity stems from their prior knowledge and learning ability. We solve this using machine teaching, an inverse problem of machine learning, where the target is known. Using such stylized models allows us to consider how to best partition the class, explore the trade-offs between the teacher’s orchestration cost and students’ workload. We apply our model to the task of teaching to distinguish between certain species of butterflies and moths and teaching children to write with the CoWriter project. The sequence chosen exhibits an intuitive curriculum, going from easy to hard examples, even though we did not enforce this constraint.
Student simulations is the use of probabilistic models built from assumptions about students learning processes and trained on student data. In our research we used such simulations to understand the behavior of students in MOOCs, to analyses the inductive reasoning strategies of children and to predict the rate of progress of students for activities in classrooms.
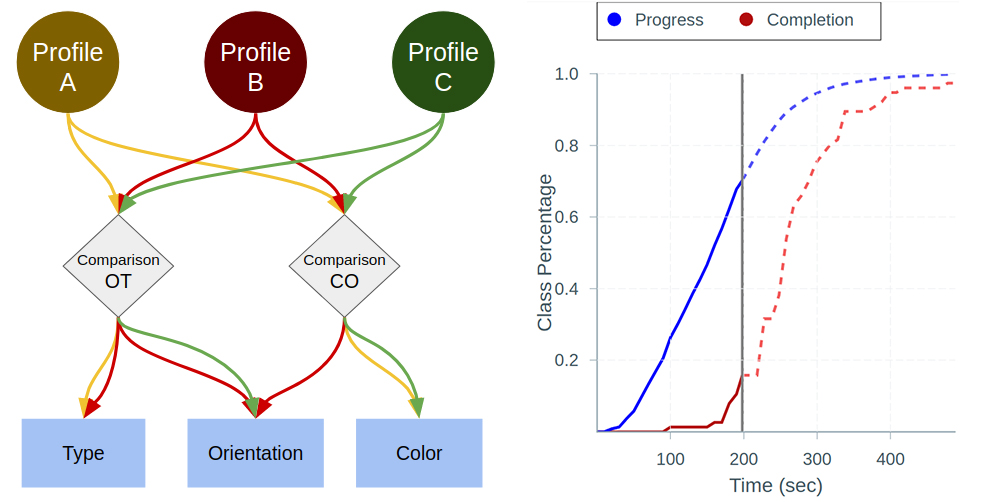
Related Publications
Please note that the publication lists from Infoscience integrated into the EPFL website, lab or people pages are frozen following the launch of the new version of platform. The owners of these pages are invited to recreate their publication list from Infoscience. For any assistance, please consult the Infoscience help or contact support.