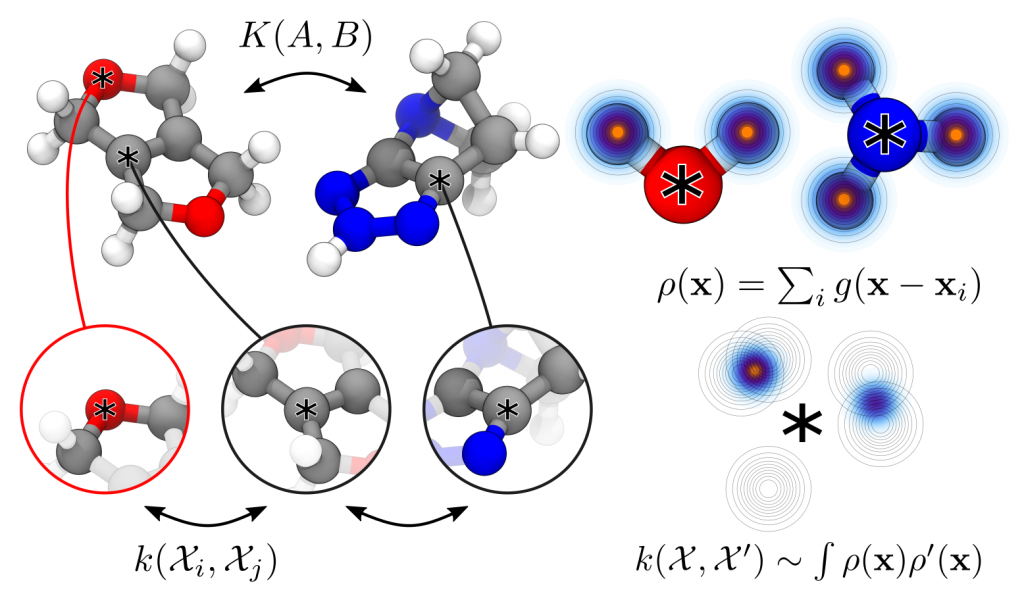
Machine-learning shows great promise to complement accurate electronic-structure methods in predicting atomic-scale properties from first principles. We work on developing and applying representations of atomic-scale structure that capture the essential physical symmetries and can be used seamlessly across many classes of systems – from small molecules, to materials and biological systems.
These mathematically robust and physically motivated structural descriptors can also be used to generate simplified representation of complex datasets and to predict the non-linear optical response of solutions and interfaces.
Entropy-regularized Kernels to Capture the Similarity Between Structures
Kernel function are basic ingredients of many machine-learning schemes, that should represent the similarity between structures, and provide a link between structure and properties. In order to generate kernels that can be applied to arbitrary structures, from the gas to the condensed phase, we build kernels by combining similarities between local environments, using e.g. the Smooth Overlap of Atomic Positions (SOAP) kernel, extended to represent the similarity between different elements in the periodic table. An entropy-regularized matching schemes makes it possible to interpolate between a comparison that weighs equally all the possible pairings (leading to properties that are an additive combination of environments) and one in which only the best-matching environments are compared. This scheme demonstrated excellent performance in applications to molecules, materials and drug design.