Single View Camera Pose Estimation Dataset
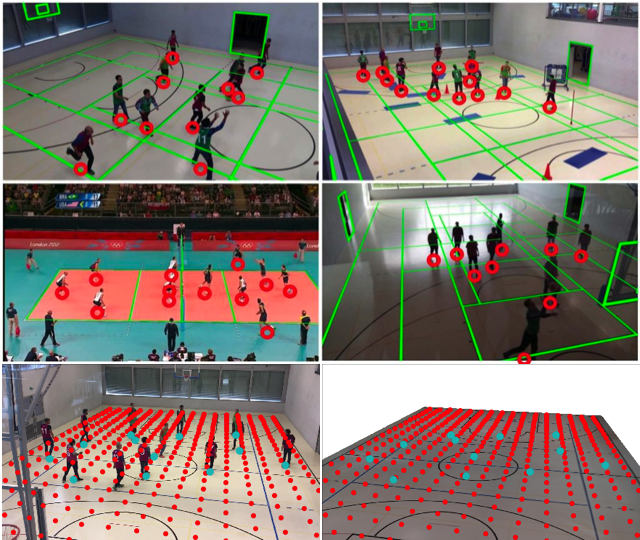
The dataset is composed of image sequences acquired with two different, and moving, smartphones (SamsungA5 and Iphone6) of an amateur basketball match. Each image is associated with a camera pose and a homography w.r.t the world coordinate system placed at the center of the court. The intrinsic matrices and the template of the court are provided. In addition, player positions on the ground are also provided for a subset of the images.
Note: For privacy reasons, the faces of the participants have been blurred. The results reported in [Citraro20] are however computed on the original images.
The set is composed of 50,127 images forming 28 distinct sequences, some of which feature difficult light conditions and foreign objects such as gymnastic mats and other pieces of equipment occluding parts of the field.
In addition to the basketball dataset, we provide estimated homography matrices and player positions for four sequences of the Volleyball dataset. [Ibrahim16]
This data is free of use for research purposes. We invite to cite our work if you find this data useful.
The data was acquired at the Centre Sport et Santé (CSS) of the University of Lausanne (UNIL) and École Polytechnique Fédérale de Lausanne (EPFL).
Download
Single view basketball dataset
Volleyball poses, homographies and player positions
contact: [email protected]
Real-time camera pose estimation for sports fields
Machine Vision and Applications (MVA). 2020-03-25. Vol. 31, num. 3, p. 16. DOI : 10.1007/s00138-020-01064-7.Multi-View Human Pose Estimation Dataset
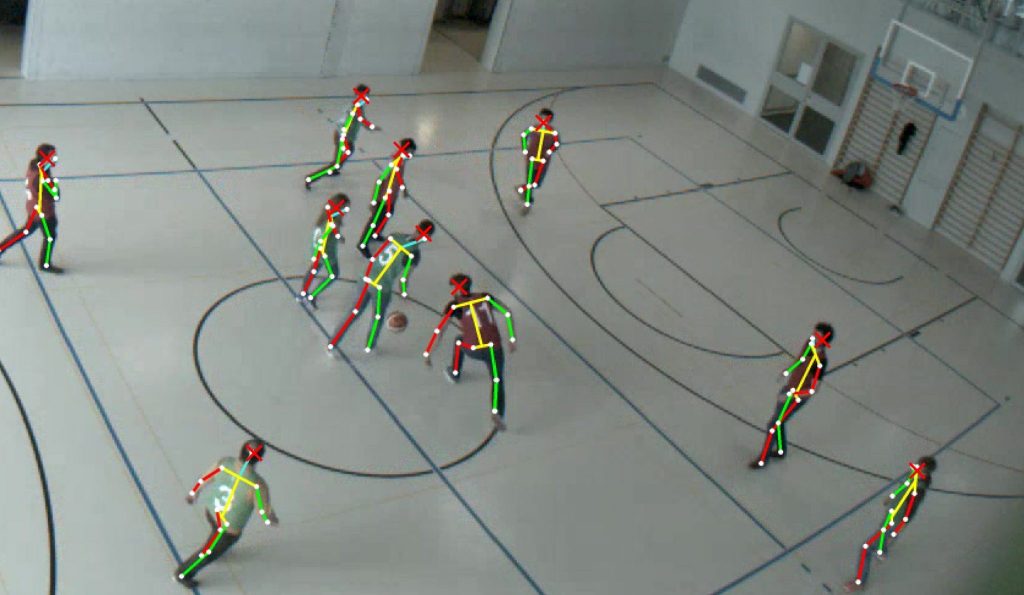
The dataset is composed of image sequences acquired from 8 fixed, synchronized and calibrated cameras depicting an amateur basketball match. The images feature a variable number of subjects ranging from 10 to 13 that are either running, walking, or standing still. The players might be occluded either by others or by various objects, such as the metal frames of the nets. There are also substantial light variations that makes it even more challenging. We computed the players’ trajectories for the whole sequence and manually annotated a subset with 2D human poses. Thereafter, we obtained the 3D poses by triangulating the manually annotated 2D detections. The dataset comprises ~370,000 images for which 560 are provided with 2D and 3D human poses. In addition to these manually annotated poses, we provide poses for 1000 temporal frames that we obtained from a pose model trained using active learning. More precisely, we alternated between manual annotation and training of a pose model till convergence.
Download
contact: [email protected]