In both life and material sciences, electron microscopy is at the heart of much recent progress. They range from a better understanding of the brain connectivity that underpins neural function to modeling and tuning 3D structures and material properties at the nanometer scale. The figure below illustrates a few use cases.
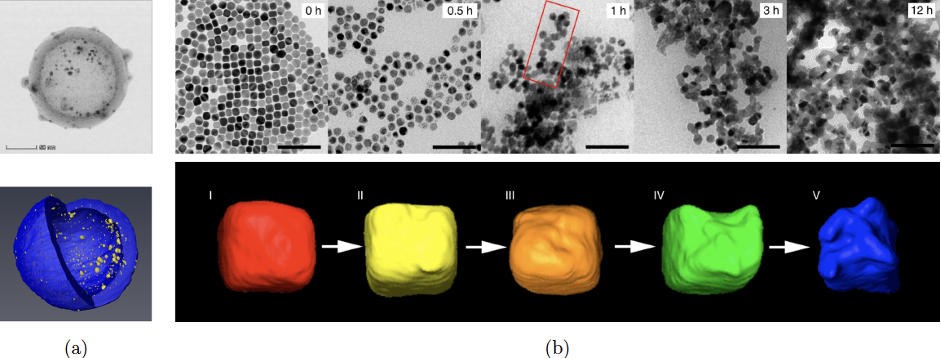
Electron tomography is a key technique to recover 3D structures by tilting the sample at various angles with respect to the electron beam. Unfortunately, existi.ng techniques require acquiring many images, which makes them slow and not well suited to modeling dynamic processes.
The goal of this project will be to explore deep learning techniques inspired by stable diffusion approaches to overcoming these limitations and then to continue developing them in the context of a PhD thesis. It will be conducted in collaboration with the Electron Spectrometry and Microscopy Laboratory.
Prerequisites:
- Masters in Computer Science or related field.
- An interest in combining physics and computer science is a must.
- Familiarity with the Fourier Transform and its applications would be a plus.
Contact:
If you are interested, please write to [email protected] and [email protected]
References:
[1] O. Altingovde and A. Mishchuk and G. Ganeeva and E. Oveisi and C. Hebert and P. Fua, 3D Reconstruction of Curvilinear Structures with Stereo Matching Deep Convolutional Neural Networks. Ultramicroscopy, 234:113460, 2022.
[2] E. Oveisi, A. Letouzey, S. De Zanet, G. Lucas., M. Antoni, P. Fua, and C. Hebert. Stereo-Vision Three-Dimensional Reconstruction of Curvilinear Structures Imaged with a TEM. Ultra-Microscopy, 184(A), 2018.