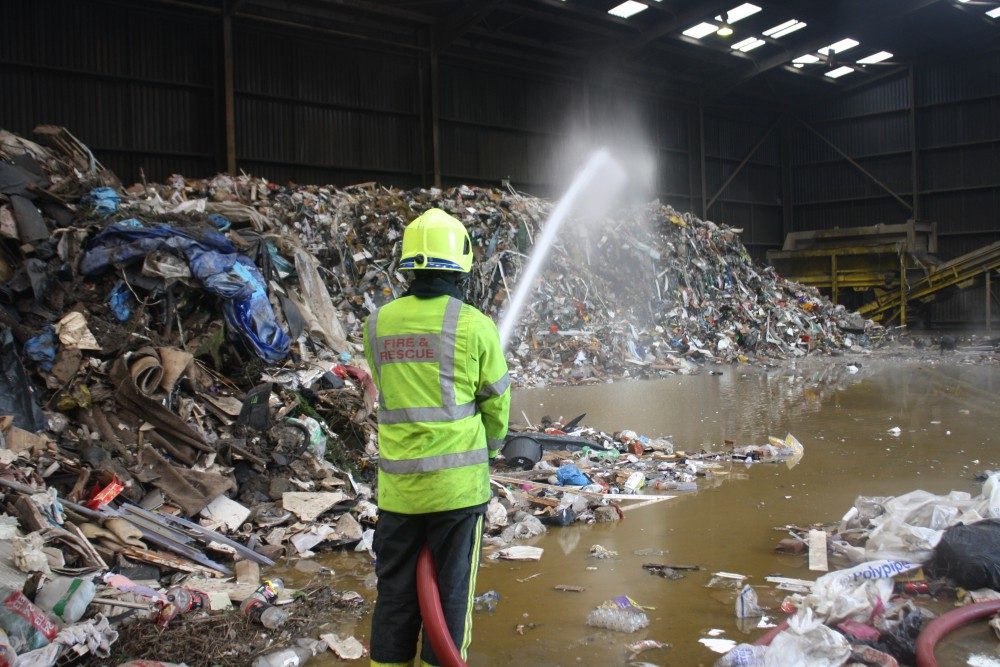
Every year we generate globally 2 billion tonnes of waste and WorldBank forecast that this number could rise up to 3.4 billion tonnes in 2050. To transform this matter into valuable we need to tackle the problem of efficient recycling. The recycling process is composed of multiple steps from waste collection to material transformation, however the most difficult is sorting or recognizing value in a waste mix. At WasteFlow we are developing a Saas for recycling facilities to help them optimize their recycling process by understanding waste mix at different steps of the recycling process.
Waste sorting facilities process an important number of different waste types that can become dangerous when manipulated such as batteries, gaz tanks etc.. These dangerous wastes cause incidents weekly at recycling facilities and create an unsafe working environment for workers. This project has the objective to explore the use of infrared, thermal, depth, and RGB data for the problem of detecting and classifying an object or a group of objects as dangerous in the case of partially or fully hidden objects. The developed system has the objective to recognize as much dangerous waste from the first layer of a pile of waste. Through this project field experimentation will be possible to gather more data and test the system.
The work will be done in collaboration with a member of WasteFlow team and a research supervisor at CVLAB.
Key Domains
- Deep Learning
- Sensor-fusion
- Recycling
Prerequisite
- Proficiency in Python
- Basics of Computer Vision and Machine Learning with experience in Pytorch or similar libraries
Contact
- Valentin Ibars @ WasteFlow – valentin.ibars@wasteflow.ai
- Mathieu Salzmann @ CVLab – mathieu.salzmann@epfl.ch