The goal of Deep Domain Adaptation is to make it possible to use Deep Nets trained in one domain where there is enough annotated training data in another where there is little or none. Most current approaches have focused on learning feature representations that are invariant to the changes that occur when going from one domain to the other, which means using the same network parameters in both domains. While some recent algorithms explicitly model the changes by adapting the network parameters, they either severely restrict the possible domain changes, or significantly increase the number of model parameters.
By contrast, we introduce a network architecture that includes auxiliary residual networks, which we train to predict the parameters in the domain with little annotated data from those in the other one. This architecture enables us to flexibly preserve the similarities between domains where they exist and model the differences when necessary.
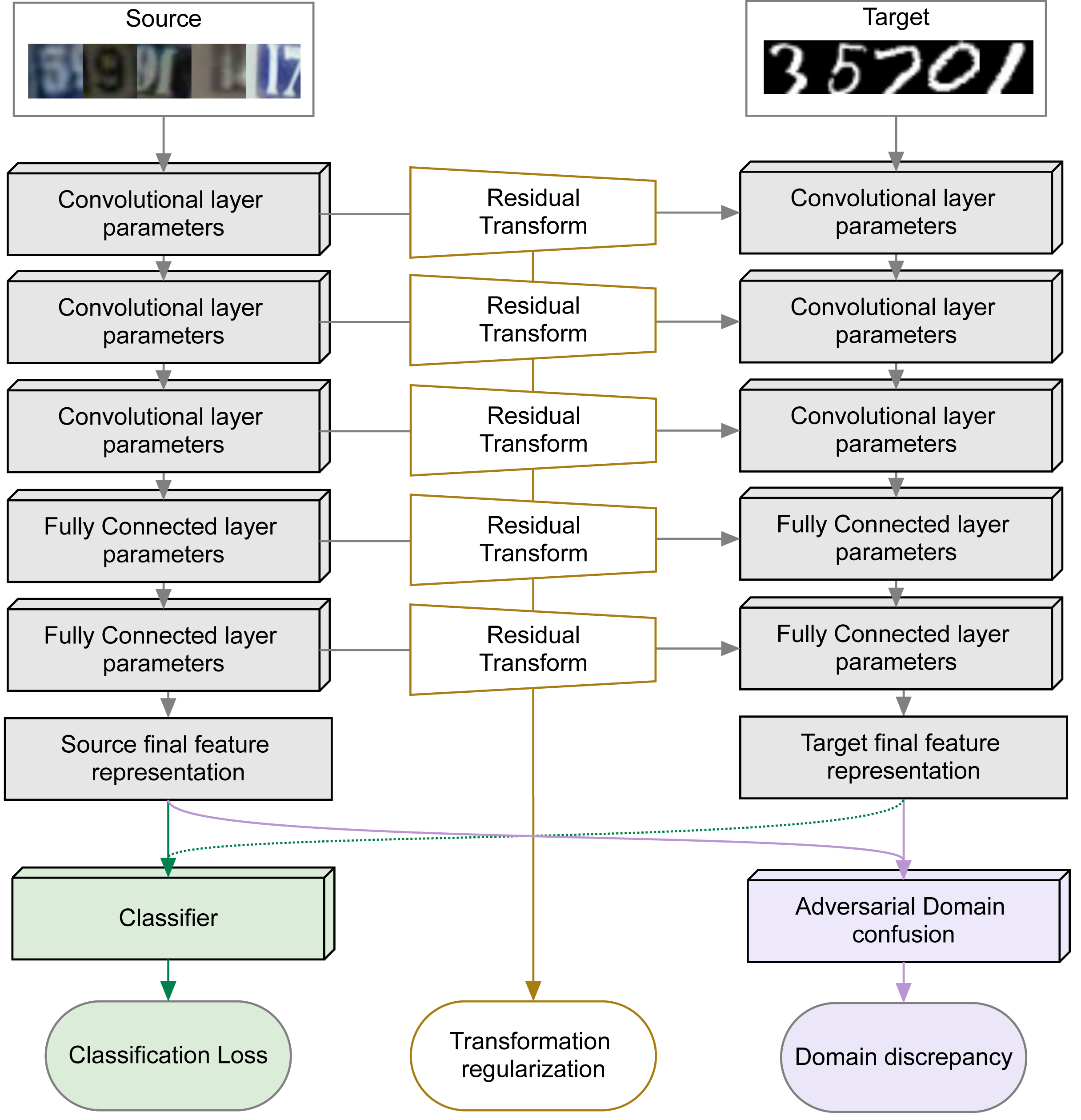
Residual transformation can be represented as follows:
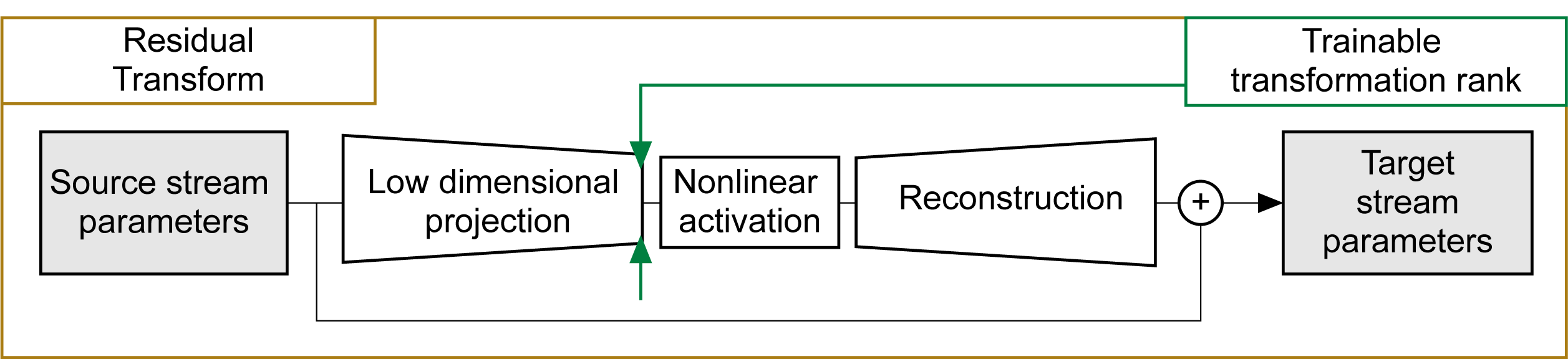
For more information please check the Residual Parameter Transfer for Deep Domain Adaptation paper, as depicted below.
Framework overview
We start by pretraining the source stream on the source data. We then continue training both the source stream and the residual transformation parameters by minimizing the objective function that consists of four components:
- Classification loss term (cross-entropy);
- Domain discrepancy loss term (any loss that maximizes domain confusion);
- Residual Transformation Regularization loss term;
- Automatic architecture selection loss term.
The overall training and testing procedure is described in the following figure.
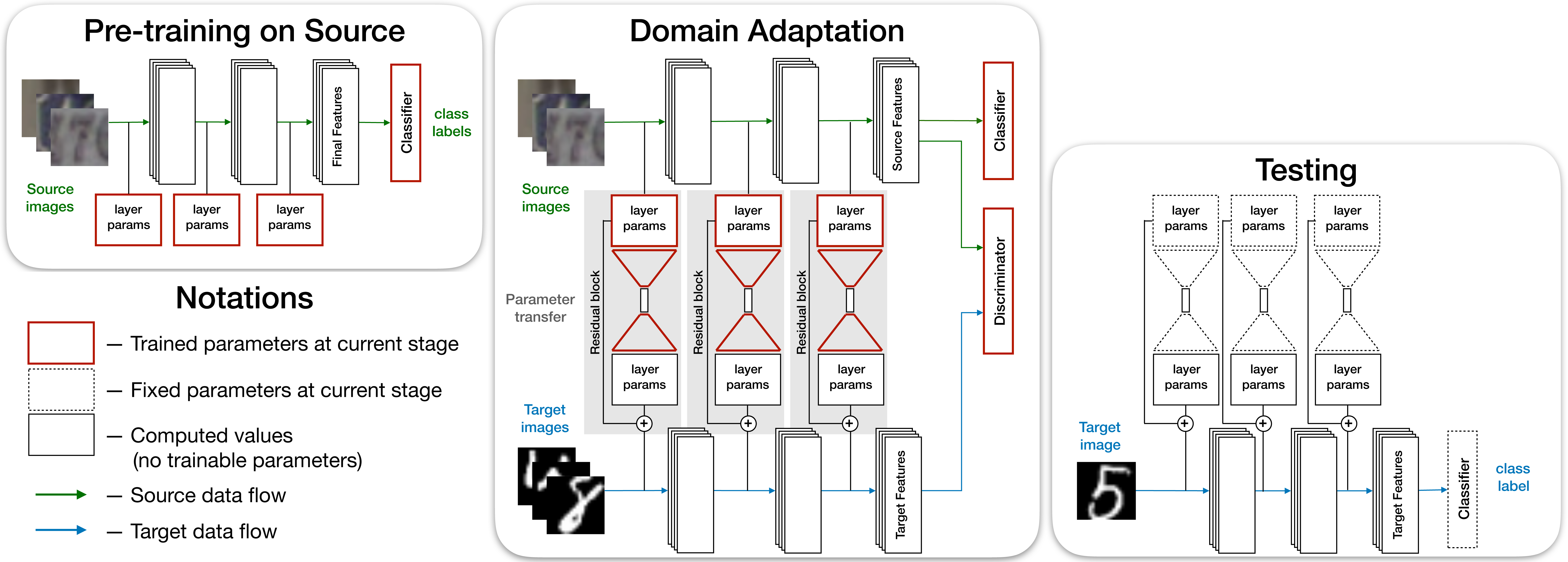
For more information please check the Residual Parameter Transfer for Deep Domain Adaptation paper, as depicted below.
Code
The code for the project is available under the following links: drive.switch; c4science.
References
Please note that the publication lists from Infoscience integrated into the EPFL website, lab or people pages are frozen following the launch of the new version of platform. The owners of these pages are invited to recreate their publication list from Infoscience. For any assistance, please consult the Infoscience help or contact support.