Mean-Field Inference for Conditional Random Fields
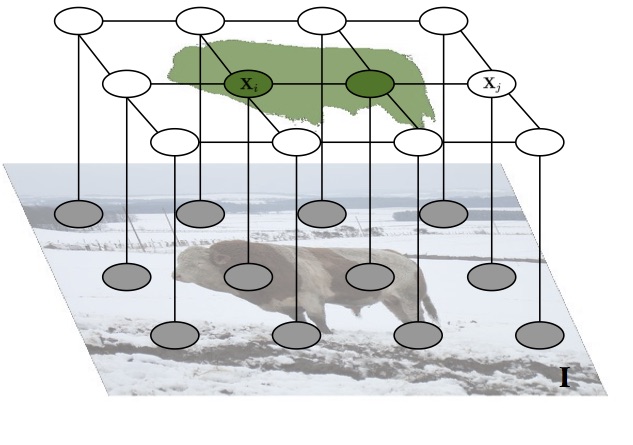
Mean-field variational inference is one of the most popular approaches to inference in discrete random fields. Standard mean-field optimization is based on coordinate descent and in many situations can be impractical. Thus, in practice, various parallel techniques are used, which either rely on ad hoc smoothing with heuristically set parameters, or put strong constraints on the type of models.
To remove these limitations, we have proposed a novel proximal gradient-based approach to optimizing the variational objective. It is naturally parallelizable and easy to implement. We have proved its convergence, and demonstrated that, in practice, it yields faster convergence and often finds better optima than more traditional mean-field optimization techniques. Moreover, our method is less sensitive to the choice of parameters.