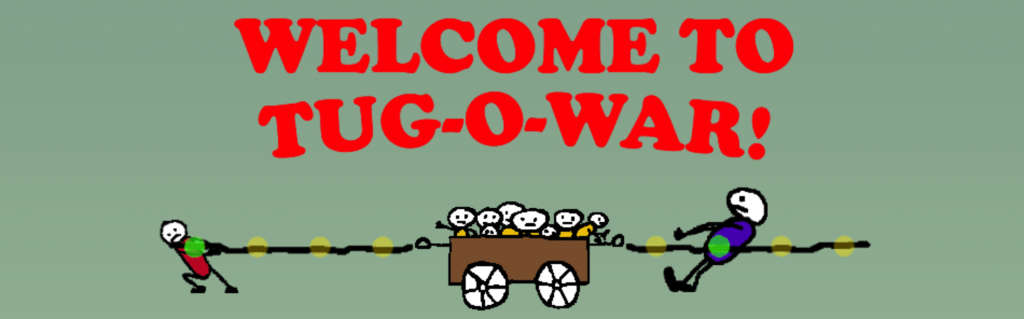
Modeling Exploratory Strategies in Interactive Educational Games via Reinforcement Learning
In recent years, open-ended learning environments, such as games, have received more and more attention. Within these environments, students can play with the interface in order to grasp notions and concepts pertaining to the educational domain by themselves. Providing students with learning scenarios where they are required to explore a gamified environment has a potential to shape better student’s exploratory pathways and improve their decision-making skills.
When students play within an open-ended learning environment, it becomes crucial to choose what content/problem to give them so that they learn as much as possible. To this end, reinforcement learning, in which automated agents learn how to perform tasks by interacting with environments, represents a key machine-learning strategy. Reinforcement learning agents can be optimized to simulate human behavior, and then behavioral strategies can be induced into them to analyze how students might behave/perform under a certain task. However, while simulating human behavior via agents has been widely explored in many contexts, its use in education is still under-explored.
This project aims at modeling exploratory strategies of students in interactive educational games. In collaboration with the AAALab at the Stanford Graduate School of Education, we are conducting studies on TugLet, an interactive computer-based environment they developed to assess student’s exploration choices by means of a tug-of-war game, modeled with respect to forces and motions. Students can simulate tug-of-war setups or guessing the winning side of a certain tug-of-war setup and receive the corresponding right-wrong answer. The final student’s goal is to understand how a team’s size is associated with the strength of its pull, so that they can decide the winning team for an unseen scenario. This is the context where we are optimizing reinforcement learning agents on student behavioral data to induce pedagogical policies and analyze educational outcomes.
The outputs of this project aim to advance learning by characterizing students’ interactions and uncovering exploratory behaviors worth of teaching, linking exploratory patterns to their learning outcomes, and supporting the integration of new pedagogical policies into the teaching practices.