DISAL offers a range of student projects its three main areas of expertise: Distributed Robotic Systems, Sensor and Actuator Networks, and Intelligent Vehicles. For more information about supervision guidelines and different types of student projects please refer to this page.
Fall Semester 2024-2025
Odometry Frames Alignment with Fiducial Markers
![]() |
![]() |
Community-critical steel infrastructure, such as energy transmission and telecommunication lines, steel bridges, offshore rigs, and so on, requires regular inspection to guarantee the integrity of the structure and sound operation. Current inspection approaches usually require the use of heavy equipment, as well as visual inspection performed by technicians, which exposes them to potentially dangerous situations. Furthermore, inspections usually imply downtime for the structure under inspection, which is costly and inefficient. The main interest is, therefore, to identify fatigue signs within a structure without requiring it to stop its usage. Micro Aerial Vehicles (MAVs) endowed with visual and depth-sensing capabilities are good candidates for this goal.
When considering inspection scenarios with multiple robots, these robots need to localize relative to a common reference frame. In case of GNSS-denied navigation and relying solely on onboard sensors, this can prove challenging. Solutions, such as distributed SLAM, exist but are computationally expensive. This project aims at implementing a reference frame alignment pipeline using fiducial markers (namely AprilTags [1]) to provide a shared static frame of reference to all robots when they observe the reference tag. To this end, a self-contained ROS node shall be implemented that leverages computer vision libraries (e.g., OpenCV [2]) to undistort the acquired camera images (supporting both pinhole and fish eye camera models), run the fiducial marker detection continuously (using the AprilTag library [1]) and produce an accurate localization of the reference tag to enable the alignment the individual robots’ odometry frames. The consecutive tag detections should be filtered appropriately (using weights proportional to the quality of the detection and using an appropriate mathematical formulation to fuse measurements under uncertainty). The implemented approach will be developed and tested on simulated MAVs in Webots [3], and later validated on real hardware if time permits.
Recommended type of project: semester project
Work breakdown: 20% theory, 50% coding, 30% simulation
Prerequisites: Broad interest in robotics; excellent programming skills (C/C++); good knowledge of ROS; basic knowledge of Webots and git.
Keywords: MAV, AprilTag, sensor fusion, Webots, simulation
Contact: Lucas Wälti
References:
[1] Wang John, and Edwin Olson. “AprilTag 2: Efficient and Robust Fiducial Detection.” In 2016 IEEE/RSJ International Conference on Intelligent Robots and Systems, 4193–98, 2016. https://doi.org/10.1109/IROS.2016.7759617.
[2] “OpenCV: OpenCV Modules.” Accessed June 6, 2024. https://docs.opencv.org/4.10.0/.
[3] “Webots: Robot Simulator.” Accessed October 6, 2022. https://cyberbotics.com/.
Geometry-Informed Path Planning for Steel Structure Inspection
Assigned to: Zhu Zhengming
![]() |
![]() |
Community-critical steel infrastructure, such as energy transmission and telecommunication lines, steel bridges, offshore rigs, etc… require regular inspection, in order to guarantee the integrity of the structure and good operations. Current inspection approaches usually require the use of heavy equipment, as well as visual inspection performed by technicians, which exposes them to potentially dangerous situations. Furthermore, inspections usually imply down-time for the structure under inspection, which is costly and inefficient. The main interest is therefore to identify fatigue signs within a structure, without requiring to stop its usage. Micro Aerial Vehicles (MAVs) endowed with visual and depth sensing capabilities are potentially good candidates to achieve this goal.
This project aims at developing a navigation strategy suitable for the inspection of steel structures with an MAV equipped with a forward-facing depth camera. Previous projects have studied the reconstruction of the structure leveraging two methods, namely: 3D Hough transform [1] and Iterative Closest Point (ICP) [2] to map the structure, and also compensate the drift accumulated over time in the MAV’s state estimate by aligning consecutive measurements. In this work, you will propose a navigation solution leveraging the geometric understanding of the structure offered by the Hough transform and assess how it allows the MAV to explore and cover the structure, as well as how well the previously-implemented relocalization methods can adjust the MAV’s pose estimate, given the implemented navigation approach. The proposed solution can take inspiration from informative path planning solutions, cf. [3-5] for some examples, but will likely differ from them due to the specific scenario considered here. The project can be broken down into two parts, namely a coverage and exploration part. This work will be carried on a simulated MAV in Webots [6].
Recommended type of project: semester/master project
Work breakdown: 60% theory, 40% simulation
Prerequisites: Broad interest in robotics; excellent programming skills (C/C++); good knowledge in ROS, path planning algorithms; basic knowledge in Webots, git.
Keywords: Webots, simulation, MAV, point cloud, 3D, segmentation
Contact: Lucas Wälti
References:
[1] Dalitz Christoph, Schramke Tilman, and Jeltsch Manuel. “Iterative Hough Transform for Line Detection in 3D Point Clouds.” Image Processing On Line 7 (July 19, 2017): 184–96. https://doi.org/10.5201/ipol.2017.208.
[2] “Point Cloud Library (PCL): Pcl::IterativeClosestPoint< PointSource, PointTarget, Scalar > Class Template Reference.” Accessed June 6, 2024. http://pointclouds.org/documentation/classpcl_1_1_iterative_closest_point.html.
[3] Papachristos Christos, Kostas Alexis, Garcia Carrillo Luis Rodolfo, and Tzes Anthony. “Distributed Infrastructure Inspection Path Planning for Aerial Robotics Subject to Time Constraints.” In International Conference on Unmanned Aircraft Systems , 406–412, 2016. https://doi.org/10.1109/ICUAS.2016.7502523.
[4] Song Soohwan, and Sungho Jo. “Surface-Based Exploration for Autonomous 3D Modeling.” In IEEE International Conference on Robotics and Automation, 4319–4326. Brisbane, Australia, 2018. https://doi.org/10.1109/ICRA.2018.8460862.
[5] Meng Zehui, Hailong Qin, Chen Ziyue, Chen Xudong, Hao Sun, Feng Lin, and Ang Marcelo H. “A Two-Stage Optimized Next-View Planning Framework for 3-D Unknown Environment Exploration, and Structural Reconstruction.” IEEE Robotics and Automation Letters 2, no. 3 (July 2017): 1680–87. https://doi.org/10.1109/LRA.2017.2655144.
[6] “Webots: Robot Simulator.” Accessed October 6, 2022. https://cyberbotics.com/.
Gas Source Localization with Gaussian Markov Random Field
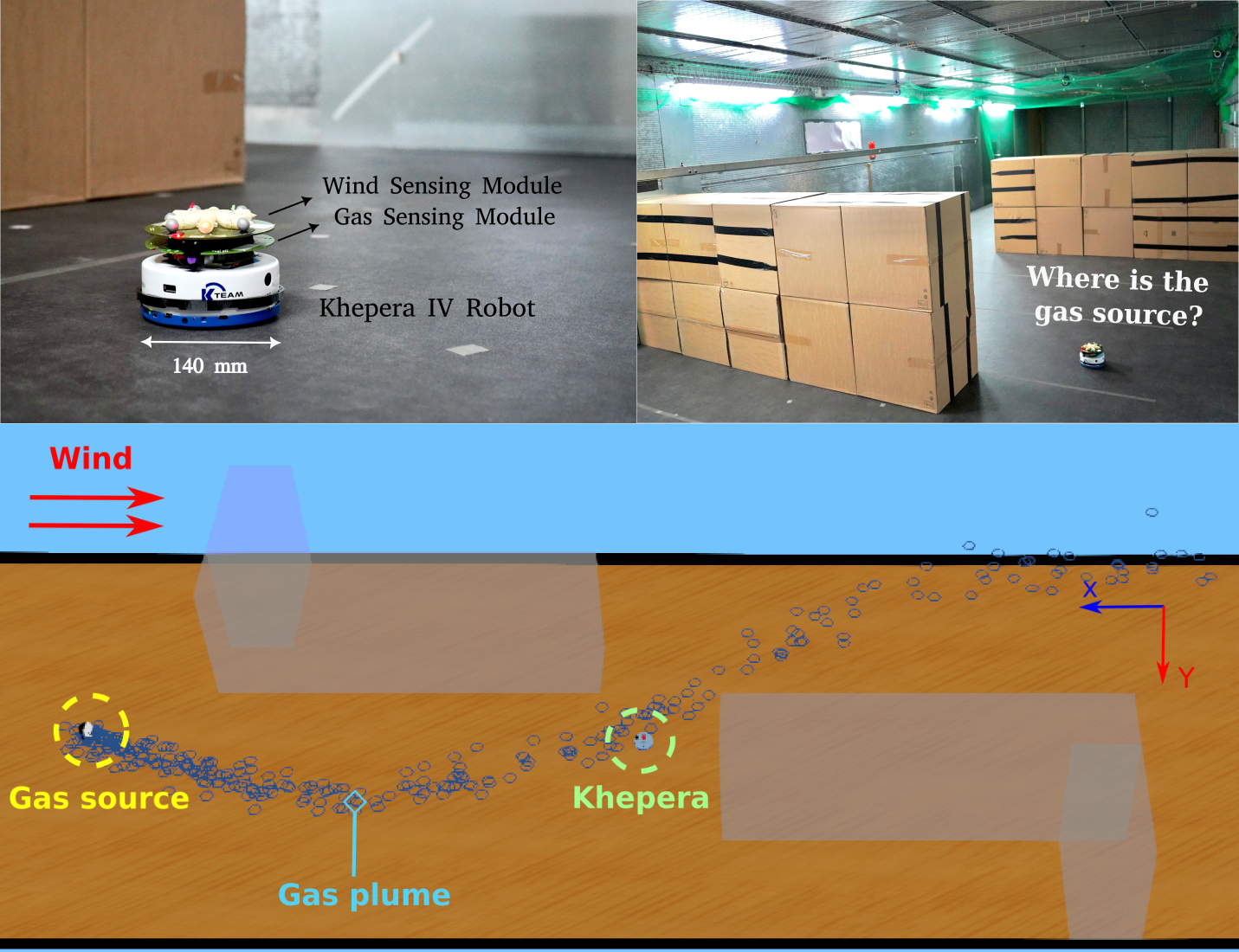
The deployment of robots for Gas Source Localization (GSL) tasks in hazardous scenarios significantly reduces the risk to humans and animals, given their autonomy and sensing capability (gas and wind). GSL is a challenging task in the real world due to the stochastic nature of the gas dispersion phenomenon, primarily influenced by the advection caused by airflow, which directs the movement of molecules. The environmental structure and obstacles shape the airflow, and therefore impact the gas dispersion process [1]. Estimating the overall wind flow offer valuable information about the gas trace, substantially enhancing GSL task efficiency.
This project aims to explore the integration of an optimization-based field estimation algorithm – Gaussian Markov Random Field to GSL tasks [2][3]. The student will start by understanding its working principles, investigating its solutions and evaluating their performance in the high-fidelity robotics simulator Webots [4]. If the results are promising and if time allows, physical experiments with a real mobile robot, equipped with gas and wind sensing modules, will be conducted in a wind tunnel.
Recommended type of project: semester project, master project
Work breakdown: 40% theory, 60% simulation
Prerequisites: passion on solving issues, broad interest in robotics, good programming skill (C/C++), experience with Webots and real robot experiments will be an asset
Keywords: gas source localization, wind information, optimization
Contact: Wanting Jin
References:
[1] W. Jin, F. Rahbar, C. Ercolani, and A. Martinoli, “Towards Efficient Gas Leak Detection in Built Environments: Data-Driven Plume Modeling for Gas Sensing Robots,” in 2023 IEEE International Conference on Robotics and Automation (ICRA). London, United Kingdom: IEEE, May 2023, pp. 7749–7755.
[2] J. G. Monroy, J.-L. Blanco, and J. Gonzalez-Jimenez, “Time-variant gas distribution mapping with obstacle information,” Auton. Robots, vol. 40, no. 1, pp. 1–16, Jan. 2016.
[3] C. Rhodes, C. Liu, W. Chen. Structurally aware 3D gas distribution mapping using belief propagation: a real-time algorithm for robotic deployment. IEEE Transactions on Automation Science and Engineering, vol. 21, no. 2, pp. 1623-1637, April 2024.
[4] Webots: https://cyberbotics.com/doc/reference/index.
Gas Source Localization with Physics-Informed Neural Networks (PINN)
![]() |
In case of gas leaks, to estimate the source position with the gas concentration measured at different locations in the environment becomes an ill-posed inverse estimation problem. This complexity arises from the stochastic nature of the gas dispersion phenomenon. The gas dispersion process is mainly governed by the wind flow, influenced by the environment inlet and outlet condition, as well as obstacle configuration. Given the impracticality of extensively exploring and monitoring the entire environment in terms of time and resources, integrating physical knowledge about the gas dispersion process and the environmental information is crucial to ensure the success and efficiency of the localizing source position from scattered measurements [1].
The Physics-Informed Neural Networks (PINNs) [2] can incorporate the knowledge of general physical laws, described by partial differential equations, that govern a given dataset in the learning process. Consequently, PINNs hold significant potential in addressing Gas Source Localization (GSL) problems. In the previous semester project, the integration of PINNs for GSL tasks has already been explored and achieved some satisfying preliminary results. In this project, the extension of PINNs to incorporate environmental information to enhance its generalization capabilities under different boundary conditions will be investigated [3]. The algorithm performance will be tested with a simulated sensor network in the high-fidelity robotics simulator Webots [4].
Recommended type of project: semester project, master project
Work breakdown: 40% theory, 60% programming
Prerequisites: passion on solving issues, broad interest in robotics and deep learning, good programming skill (C/C++), experience with PINNs and fluid dynamics will be an asset
Keywords: gas source localization, neural networks, sensor networks
Contact: Wanting Jin
References:
[1] W. Jin, F. Rahbar, C. Ercolani, and A. Martinoli, “Towards Efficient Gas Leak Detection in Built Environments: Data-Driven Plume Modeling for Gas Sensing Robots,” in 2023 IEEE International Conference on Robotics and Automation (ICRA). London, United Kingdom: IEEE, May 2023, pp. 7749–7755.
[2] Raissi, M., Perdikaris, P. and Karniadakis, G.E., 2019. Physics-informed neural networks: A deep learning framework for solving forward and inverse problems involving nonlinear partial differential equations. Journal of Computational physics, 378, pp.686-707.
[3] V. Schäfer, M. Bracke, R. Pinnau, et al. “Generalization of PINNs for various boundary and initial conditions”, 2022, Master Thesis, University of Kaiserslautern.
[4] Webots: https://cyberbotics.com/doc/reference/index
Autonomous Visual Line-of-sight Inspections for Micro Aerial Vehicles
![]() |
![]() |
Visual Line-Of-Sight (VLOS) flight of a Micro Aerial Vehicle (MAV) requires a remote pilot/observer to clearly see the vehicle and the surrounding airspace at all times while it is airborne [1]. In Switzerland, for the “Open” category, VLOS should always be maintained with the vehicle [2]. Although it is possible to apply for a Beyond VLOS (BVLOS) certification in the “Specific” category for fully autonomous flight, this procedure is rather complex and takes a long time.
This project aims to develop a control and estimation strategy to perform autonomous inspections of MAVs while considering VLOS constraints. In particular, the MAV should detect the observer by using its sensors, estimate its position while performing inspection tasks, and adjust its trajectories to help the remote pilot maintain VLOS conditions. It is planned to leverage optimization-based methodologies [3,4] for motion planning such as Model Predictive Control (MPC). The algorithms will be developed and tested in a high-fidelity robotic simulator (Webots) using the Robot Operating System (ROS) in environments where the complexity of inspection regions changes. Various metrics will be defined to evaluate the performance of inspection and quality of VLOS flights.
Recommended type of project: semester project/master project
Work breakdown: 30% theory, 40% coding, 30% simulation
Prerequisites: Broad interest in robotics, excellent programming skills (C/C++), moderate knowledge of ROS and MPC, basic knowledge in Webots/Gazebo, git.
Keywords: VLOS, MAV, inspection, object detection, MPC, constrained satisfaction
Contact: İzzet Kağan Erünsal
References:
[1] https://ruas.co.uk/vlos-evlos-bvlos-whats-the-difference/
[2] https://www.bazl.admin.ch/bazl/en/home/drohnen.html
[3] I. K. Erunsal, J. Zheng, R. Ventura and A. Martinoli, “Linear and Nonlinear Model Predictive Control Strategies for Trajectory Tracking Micro Aerial Vehicles: A Comparative Study,” IEEE/RSJ International Conference on Intelligent Robots and Systems, Kyoto, Japan, 2022, pp. 12106-12113, doi: 10.1109/IROS47612.2022.9981880.
[4] M. E. ELzaiady and A. Elnagar, “Next-best-view planning for environment exploration and 3D model construction,” 2017 International Conference on Infocom Technologies and Unmanned Systems (Trends and Future Directions), Dubai, United Arab Emirates, 2017, pp. 745-750, doi: 10.1109/ICTUS.2017.8286106.
CFD Analysis and Design Modifications of a Micro Drone for Gas Sensing Applications
![]() |
![]() |
Detecting the source of a dangerous gas is very useful for environmental monitoring and for taking fast and effective countermeasures in case of an emergency. Multi-rotor drones are becoming increasingly popular for performing this task. However, the propeller rotation inside the gas plume can compromise gas sensor readings. Therefore, an appropriate mechanical design and gas sensor configuration are essential to minimize the adverse effects of airflow on the gas sensor [1].
In a previous semester project [2], a Computational Fluid Dynamics (CFD) simulation of the gas flow in the vicinity of Crazyflie [3] nano drone was conducted using OpenFOAM [4] and Solidworks [5] to reveal the flow’s properties. Such effort needed to be complemented by experimental work to decide on the gas sensor placement [6].
In this project, taking the previous work [2], [6] as a baseline, we aim to experimentally validate the result of the CFD simulation for the Crazyflie in wind tunnel experiments further and leverage this expertise to iteratively refine the mechanical design and sensor configuration, primarily leveraging CFD tools, for the larger micro drone Starling [7], which shows significantly better autonomy and payload capacity than the Crazyflie nano drone.
Recommended type of project: semester project/master project
Work breakdown: 20% theory, 30% coding, 50% simulation and physical experiments
Prerequisites: Broad interest in robotics, good programming skills (C/C++ and/or Matlab), an introductory knowledge or interest in learning fluid dynamics, and a background in CFD will be an asset
Keywords: CFD simulations, quadrotors, gas sensing, mechanical design
Contact: İzzet Kağan Erünsal
References:
[1] S.R. Weerasinghe and M. Monasor, “Simulation and Experimental Analysis of Hovering and Flight of a Quadrotor”, 13th International Conference on Heat Transfer, Fluid Mechanics, and Thermodynamics, 2017
[2] A. Moufidi, “Validation of a High-Fidelity Simulator for Odor Sensing with a Quadrotor”, Semester project, DISAL, 2020[3] https://www.bitcraze.io/products/old-products/crazyflie-2-0/, accessed in June 2024
[4] https://www.openfoam.com/, OpenFOAM, accessed in June 2024
[5] https://www.solidworks.com/, Solidworks, accessed in June 2024
[6] Ercolani C. and Martinoli A., “3D Odor Source Localization using a Micro Aerial Vehicle: System Design and Performance Evaluation”, Proc. of the IEEE/RSJ Int. Conf. on Intelligent Robots and Systems, October 2020, Las Vegas, NV, USA, online organization, pp. 6194-6200.
[7] https://www.modalai.com/products/starling-v1, ModalAI, accessed in June 2024
Spring Semester 2024-2025
Projects for the spring semester will be announced around November 2024.