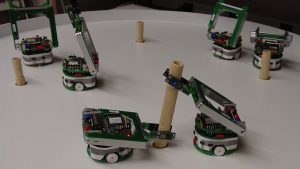
Several case studies concerned with distributed manipulation of objects have been performed in order to develop and verify probabilistic modeling techniques, bio-inspired distributed control algorithms, and control optimization methods based on machine-learning. We classify these efforts based on the target experiment although the ultimate goal of this research has been (and still is) to develop general modeling, control, and optimization methodologies for self-organized, swarm-intelligent, real-time, embedded systems.
Modeling distributed manipulation experiments
We developed a macroscopic modeling methodology for swarm-based, distributed robotic manipulation. The methodology is well-suited for nonspatial metrics as it does not take into account robots’ trajectories or the spatial distribution of objects in the environment. The strength of the proposed models is that they have been built up incrementally, with matching between models and embodied simulations (and sometimes, real robot experiments) verified at each step as new complexity was added. Precise heuristic criteria based on geometrical considerations and systematic tests with one or two embodied agents prevent the introduction of free parameters into the model. Two concrete case-studies were considered. The first case-study, referred to as the aggregation experiment, is a non-collaborative manipulation concerned with gathering and clustering small objects initially scattered in an enclosed arena. The other case-study is involves strictly collaborative manipulation and is referred to as the stick-pulling experiment, as the robots’ task is to collaborate to pull sticks out of holes in the arena floor. Results show that the proposed approach delivers quantitatively accurate predictions, in particular for nonspatial metrics related to both the aggregation and stick-pulling processes, and constitutes a computationally efficient tool. The simplicity of the modeling methodology suggests that it is easily applicable to other experiments characterized by different agent capabilities and individual control algorithms.
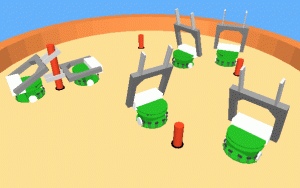
Team and Collaborators
- Kjerstin Easton
- William Agassounon
In collaboration with:
- Joel Burdick
- Kristina Lerman
- Wulfram Gerstner
Sponsors and Research Period
NSF via CNSE under Grant EEC-9402726 and Graduate Research Fellowship assigned to Kjerstin Easton
Videos
- Stick pulling with real robots (basic configuration)
- Stick pulling in simulation (Webots 2.0)
- Stick pulling with real robots equipped with a vision module
Publications
Please note that the publication lists from Infoscience integrated into the EPFL website, lab or people pages are frozen following the launch of the new version of platform. The owners of these pages are invited to recreate their publication list from Infoscience. For any assistance, please consult the Infoscience help or contact support.