In the context of seismic analysis, equivalent frame models are frequently employed to predict the behavior of masonry structures due to their computational efficiency. Nevertheless, a significant impediment within the modeling workflow is the manual and time-consuming generation of model geometry via computer-aided design software. This research aims to streamline this process by harnessing recent breakthroughs in computer vision and machine learning to automate model creation. We introduce a comprehensive, image-driven framework that autonomously constructs finite element meshes for both solid-element and equivalent-frame models, specifically targeting the external walls of standalone historic masonry buildings. As the input, our framework requires RGB images of the buildings processed using structure-from-motion algorithms, which create 3D geometries, and convolutional neural networks, which segment the openings and their corners. These layers are then combined to generate level-of-detail models. Our approach was subjected to rigorous testing on buildings characterized by non-uniform surface textures and complex opening arrangements. Although the derivation of solid element meshes from detailed models is relatively straightforward, the creation of equivalent frame models requires specialized algorithms for façade segmentation and mesh generation. These finite element geometries are anticipated to be of significant utility in enhancing the precision of seismic response forecasts for both damaged and undamaged buildings.
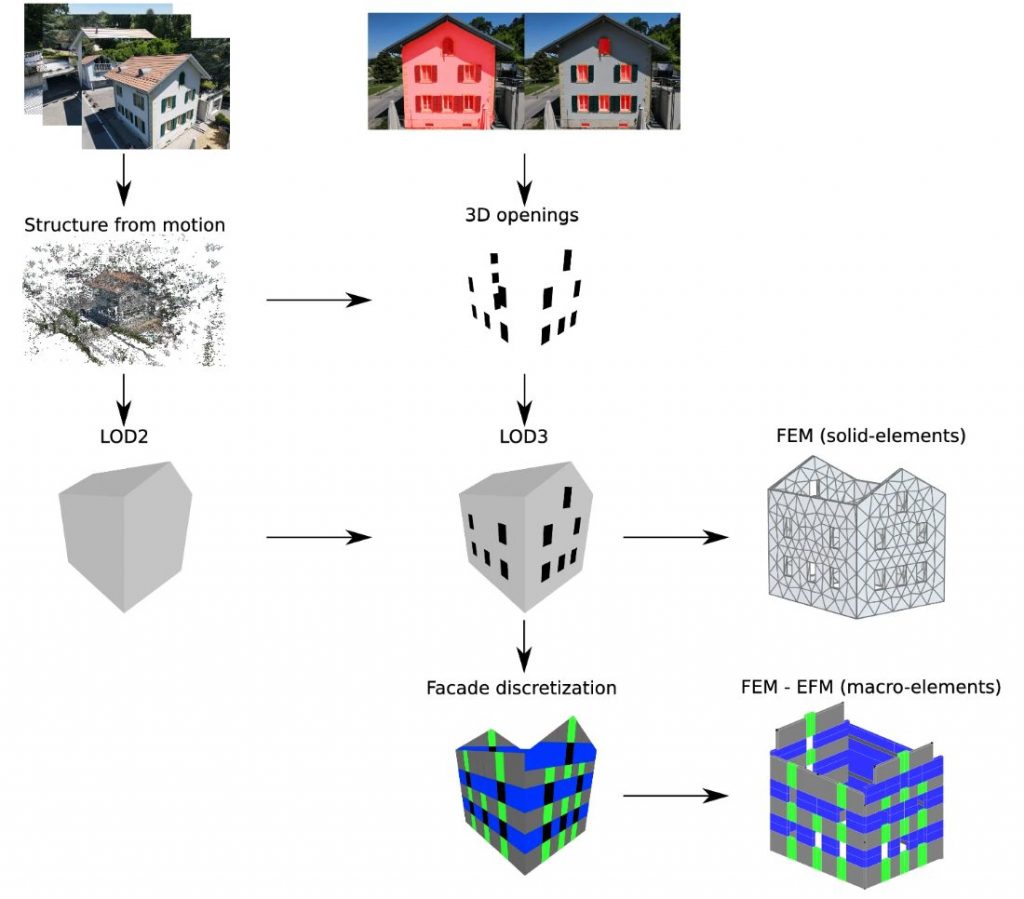
- Article:
- Automated image-based generation of finite element models for masonry buildings [journal link]
- Source code: [source code link]
- Dataset: [dataset link]
- Funding: This work was partially funded by the Swiss Data Science Center (SDSC).