Epilepsy is affecting over 60 million individuals worldwide (70.000 in Switzerland), one third of whom suffer unpredictable recurrent seizures despite treatment, translating into a major medical, social and economic burden. However, part of this burden would be relieved if seizures could be reliably detected and forecasted by non-invasive monitoring of patients in their daily routine. This would allow triggering alarms and taking immediate actions to protect patients from the many severe consequences of seizures (including death), help physicians optimising treatment based on more accurate information about patients’ seizure frequency, and help patients planned their activities with knowledge about their risk of suffering a seizure in a given time period.
Current available methods for very long-term non-invasive ambulatory tracking of seizures only detect generalized tonic-clonic seizures (GTCS), which account for less than 15% of all seizures. While a large number of algorithms aiming at detecting focal seizures were developed and mostly tested off-line, no solution is yet available to perform long-term non-invasive ambulatory detection of such seizures with an appropriate sensitivity and specificity.
The development of multi-parametric wearable devices and breakthrough progress in the fields of seizure forecasting and Internet of Health (IoH) offer novel opportunities to tackle this issue. To achieve more general seizure detection and forecasting, we advocate that multi-biosignals shall be monitored. Indeed, currently detectable biosignals known to be sensitive to epileptic seizures, including 3D-accelerometry (3D-ACC), heart rate (HR), pulse oximetry (SpO2), electrodermal activity (EDA) and electroencephalography (EEG), all have advantages and drawbacks which make them poorly effective if used in isolation. Novel technologies, including those developed by our consortium, offer new possibilities to perform multimodal recordings using both wrist-worn multisensors combined with wearable EEG adapted to very long term recordings.
On top of this multi-biosignals, seizure detection and forecasting algorithms should be personalized according to the large inter-individual variability and strong intra-individual stereotypy of epileptic seizures and patterns of recurrence, with a capacity to learn from the false detections and missed seizures recorded in each individual. Coupling seizure detection and forecasting creates a virtuous circle through which improved seizure detection helps optimising forecasting and vice versa. Finally, running such complex multi-biosensor wearable device with embedded machine learning engine comes with very challenging energy requirements that need to be tackled.
To achieve breakthrough seizure detection and forecasting, we thus propose an ambitious multi-disciplinary project that will deliver an ultra-low-energy embedded system connected to multi-parametric sensing wearables, and a central computing platform to tune the machine learning technology and lead to optimal personalized seizure detection and forecasting algorithms. The project will use an agile and adaptive organisation to optimize interaction between the data science and engineering groups developing these innovative IoH solutions and the clinical studies providing patients’ data and testing the performance of these solutions in hospital and ambulatory environments. Retrospective and prospectively collected datasets of more than 1200 patients will be used during this project, including EEG, ECG, PPG, SPO2, EDA, 3D-ACC and arm surface EMG. These data will be used to develop novel interpretable machine learning algorithms and specify the requirements for an optimal IoH solution. In parallel, several prototypes of wearables EEG, including behind the ear EEG for sleep-friendly night time recording and e-Glasses EEG for non-stigmatising day time recording, will be developed and integrated with non-EEG biosensors and low-energy embedded machine learning processor to achieve personalized and coupled seizure detection and forecasting.
We have developed smart wearable systems based on four electroencephalogram (EEG) electrodes for the detection of epileptic seizures. These systems aim to address multiple challenges that can be encountered due to this condition. For instance, they could allow to notify caregivers for rescue to avoid epilepsy-related death due to the underlying neurological disorders, sudden unexpected death in epilepsy, or accidents during seizures.
Two main systems have been developed so far: one based on the design of conventional glasses (called E-glass system) and one based on the design of bone conduction headphone (called EpiPhone system). One of the main challenges for the development of wearable for long term monitoring is to provide a solution which is both comfortable and inconspicuous to avoid any stigmata associated with the condition.
Videos
Documentation
Personalized Detection of Epileptic Seizure in the Internet of Things (IoT) Era |
Systematic Assessment of Hyperdimensional Computingfor Epileptic Seizure Detection |
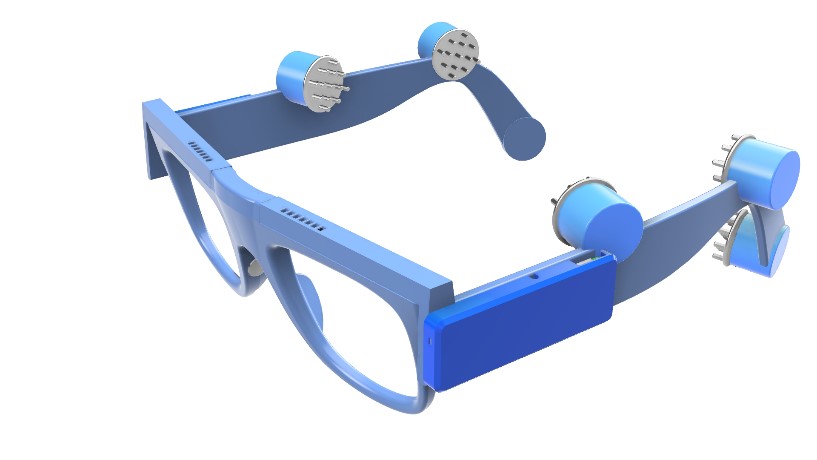
E-Glass
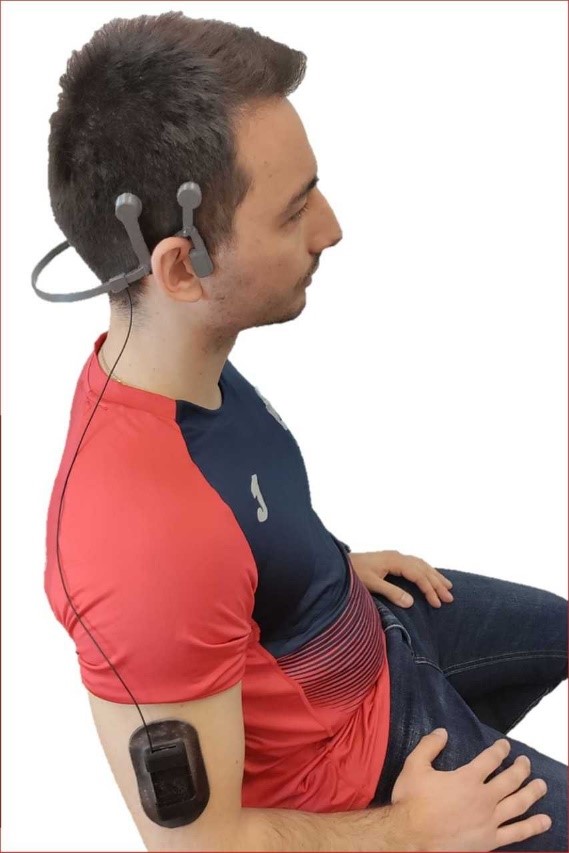
EpiPhone
News
Best Paper Award at DS4S |
Related Publications
Validation of artificial intelligence for epileptic seizure detection: Moving from research into the clinic | |||||||
Dan, Jonathan | |||||||
2024-06-19 | Developmental Medicine & Child Neurology | ![]() | |||||
M2SKD: Multi-to-Single Knowledge Distillation of Real-Time Epileptic Seizure Detection for Low-Power Wearable Systems | |||||||
Baghersalimi, Saleh; Amirshahi, Alireza; Forooghifar, Farnaz; Teijeiro, Tomas; AMIR AMINIFAR; Atienza Alonso, David | |||||||
2024-05-29 | ACM Transactions on Intelligent Systems and Technology | ![]() | ![]() | ||||
Resource-Efficient Continual Learning for Personalized Online Seizure Detection | |||||||
Shahbazinia, Amirhossein; Ponzina, Flavio; Miranda Calero, José Angel; Dan, Jonathan; Ansaloni, Giovanni; Atienza Alonso, David | |||||||
2024-04-15 | Conference Paper | ![]() | ![]() | ![]() | |||
FETCH: A Fast and Efficient Technique for Channel Selection in EEG Wearable Systems | |||||||
Amirshahi, Alireza; Dan, Jonathan; Miranda Calero, José Angel; Aminifar, Amir; Atienza Alonso, David | |||||||
2024-04-04 | Conference Paper | ![]() | ![]() | ![]() | |||
SzCORE: A Seizure Community Open-source Research Evaluation framework for the validation of EEG-based automated seizure detection algorithms | |||||||
Dan, Jonathan; Pale, Una; Amirshahi, Alireza; Cappelletti, William; Ingolfsson, Thorir Mar; Wang, Xiaying; Cossettini, Andrea; Bernini, Adriano; Benini, Luca; Beniczky, Sándor; Atienza, David; Ryvlin, Philippe | |||||||
2024 | Conference Paper | ![]() | |||||
Layer-Wise Learning Framework for Efficient DNN Deployment in Biomedical Wearable Systems | |||||||
Baghersalimi, Saleh; Amirshahi, Alireza; Teijeiro, Tomas; Aminifar, Amir; Atienza Alonso, David | |||||||
2023-10-09 | [Proceedings of IEEE BSN 2023] | ![]() | ![]() | ||||
Decentralized Federated Learning for Epileptic Seizures Detection in Low-Power Wearable Systems | |||||||
Baghersalimi, Saleh; Teijeiro, Tomas; Aminifar, Amir; Atienza Alonso, David | |||||||
2023 | IEEE Transactions on Mobile Computing | ![]() | ![]() | ||||
EpilepsyNet: Interpretable Self-Supervised Seizure Detection for Low-Power Wearable Systems | |||||||
Huang, Baichuan; Zanetti, Renato; Abtahi, Arza; Atienza, David; Aminifar, Amir | |||||||
2023 | Proceedings of the 5th IEEE International Conference on Artificial Intelligence Circuits and Systems (AICAS 2023) | ![]() | |||||
Approximate Zero-Crossing: A new interpretable, highly discriminative and low-complexity feature for EEG and iEEG seizure detection | |||||||
Zanetti, R; Pale, U; Teijeiro, T; Atienza Alonso, David | |||||||
2022-11-10 | Journal of Neural Engineering | ![]() | ![]() | ||||
M2D2: Maximum-Mean-Discrepancy Decoder for Temporal Localization of Epileptic Brain Activities | |||||||
Amirshahi, Alireza; Thomas, Anthony; Aminifar, Amir; Rosing, Tajana; Atienza Alonso, David | |||||||
2022-09-22 | IEEE Journal of Biomedical and Health Informatics (JBHI) | ![]() | ![]() | ![]() | |||
INCLASS: Incremental Classification Strategy for Self-Aware Epileptic Seizure Detection | |||||||
Ferretti, Lorenzo; Ansaloni, Giovanni; Marquis, Renaud; Teijeiro, Tomas; Ryvlin, Philippe; Atienza, David; Pozzi, Laura | |||||||
2022-03-23 | Conference Paper | ![]() | ![]() | ![]() | |||
Personalized seizure signature: An interpretable approach to false alarm reduction for longâ€term epileptic seizure detection | |||||||
Sopic, Dionisije; Teijeiro, Tomas; Atienza, David; Aminifar, Amir; Ryvlin, Philippe | |||||||
2022-02-03 | Epilepsia | ![]() | ![]() | ![]() | ![]() | ![]() | |
Personalized Real-Time Federated Learning for Epileptic Seizure Detection | |||||||
Baghersalimi, Saleh; Teijeiro, Tomas; Atienza, David; Aminifar, Amir | |||||||
2021-07-09 | IEEE Journal of Biomedical and Health Informatics | ![]() | ![]() | ![]() | ![]() | ![]() | |
Interpreting deep learning models for epileptic seizure detection on EEG signals | |||||||
Gabeff, Valentin; Teijeiro, Tomas; Zapater, Marina; Cammoun, Leila; Rheims, Sylvain; Ryvlin, Philippe; Atienza, David | |||||||
2021-05-01 | Artificial Intelligence in Medicine | ![]() | |||||
Self-Aware Anomaly-Detection for Epilepsy Monitoring on Low-Power Wearable Electrocardiographic Devices | |||||||
Forooghifar, Farnaz; Aminifar, Amin; Teijeiro, Tomas; Aminifar, Amir; Jeppesen, Jesper; Beniczky, Sandor; Atienza Alonso, David | |||||||
2021-04-12 | Conference Paper | ![]() | ![]() | ![]() | |||
Systematic Assessment of Hyperdimensional Computing for Epileptic Seizure Detection | |||||||
Pale, Una; Teijeiro, Tomas; Atienza Alonso, David | |||||||
2021 | Conference Paper | ![]() | ![]() | ||||
EpilepsyGAN: Synthetic Epileptic Brain Activities with Privacy Preservation | |||||||
Pascual Ortiz, Damián; Amirshahi, Alireza; Aminifar, Amir; Atienza, David; Ryvlin, Philippe; Wattenhofer, Roger | |||||||
2020-11-30 | IEEE Transactions on Biomedical Engineering | ![]() | ![]() | ![]() | |||
Noninvasive detection of focal seizures in ambulatory patients | |||||||
Ryvlin, Philippe; Cammoun, Leila; Hubbard, Ilona; Ravey, France; Beniczky, Sandor; Atienza, David | |||||||
2020-06-02 | Epilepsia | ||||||
Noise-Resilient and Interpretable Epileptic Seizure Detection | |||||||
Hitchcock Thomas, Anthony; Aminifar, Amir; Atienza Alonso, David | |||||||
2020-05-17 | [2020 IEEE International Symposium on Circuits and Systems (ISCAS). Proceedings] | ![]() | ![]() | ![]() | |||
Robust Epileptic Seizure Detection on Wearable Systems with Reduced False-Alarm Rate | |||||||
Zanetti, Renato; Aminifar, Amir; Atienza Alonso, David | |||||||
2020 | ![]() |