Our research is dedicated to the modeling and optimization of urban transportation systems with a special focus on emerging mobility services and technologies (e.g., ridesharing, autonomous vehicles).
- Modeling: We build models to describe strategic behaviors of stakeholders in the transportation systems (e.g., travelers, drivers, operators) and their interactions, drawing methodologies from economics and game theory.
- Optimization: We tackle the design and operational problems in transportation systems at different levels using both classic optimization tools and data-driven methods.
We also hold the mission to develop personalized mobility solutions meanwhile addressing the issues of equity and privacy.
Shared and on-demand mobility (MoD) covers a wide range of urban transport services, including taxis/robotaxis, e-hailing, ride-pooling, bike-sharing, etc. These services share the same principle, i.e., using a fleet of vehicles to serve passenger trips in a demand-responsive manner.
Our research on this topic covers the following aspects:
- physical matching and scale economies in MoD
- market equilibrium and operations management of MoD services
- network traffic equilibrium with MoD vehicles
Due to the similarities in physical demand-supply interactions and operational characteristics, the methodologies developed for mobility services are also implemented in our research of on-demand urban logistics services (e.g., meal delivery).
Transportation network modeling is a classic transportation research topic and has been studied extensively in the context of road traffic and public transit. Yet, it still serves as a useful tool to examine the overall impacts of emerging mobility services and technologies on the urban transportation system.
Our particular interests on this topic include:
- multi-modal transport system and mobility-as-a-service (MaaS)
- ride-sharing and ride-pooling
- mixed traffic with autonomous vehicles (AVs) and electric vehicles (EVs)
- nonconventional demand management instruments (e.g., mobility credit)
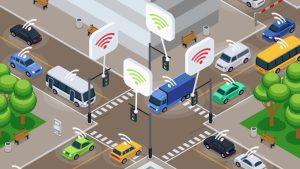
Classic network-level traffic control requests a sophisticated traffic model, which, however, often fails to predict true traffic dynamics due to strong assumptions. Besides, the centralized control problem is usually computationally expensive to solve and thus hardly scalable. The recent advances in machine learning and control theory bring new opportunities to tackle network-level traffic control in real-time without explicitly characterizing the traffic model.
Here are our previous and ongoing projects on this topic:
- data-enabled predictive control for dynamic traffic routing
- distributed optimization for networked intersection control
- learning-based ride-hailing vehicle rebalancing