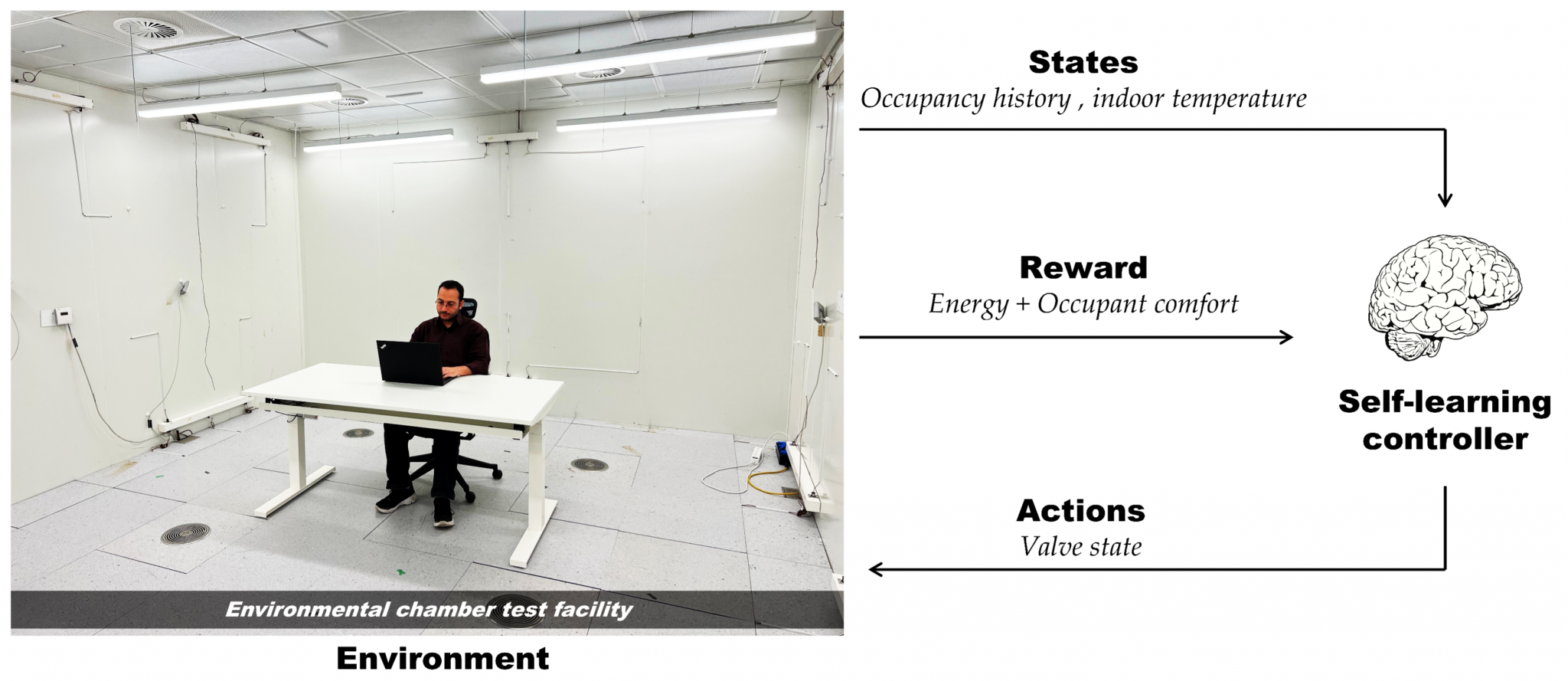
Occupant behavior is known as a key driver of building energy use. It is a highly stochastic and complicated phenomenon, driven by a wide variety of factors, and is unique in each building. Therefore, it cannot be addressed using analytical approaches traditionally used to describe physics-based aspects of buildings. Consequently, current controls which rely on hard-coded expert knowledge have a limited potential for integrating occupant behavior. An alternative approach is to program a human-like learning mechanism and develop a controller that is capable of learning the control policy by itself through interacting with the environment and learning from experience. Reinforcement Learning (RL), a machine learning algorithm inspired by neuroscience, can be used to develop such a self-learning controller. Given the learning ability, these controllers are able to learn optimal control policy without prior knowledge or a detailed system model, and can continuously adapt to the stochastic variations in the environment to ensure an optimal operation.
The main question that the study “BehaveLearn: Reinforcement Learning for occupant-centric operation of building energy systems” lead by the Ph.D. student Amirreza Heidari deals with is how to develop a controller that can perceive and adapt to the occupant behavior to minimize energy use without compromising user needs?
In this context, three occupant-centric control frameworks are developed in this study:
- DeepHot: focused on hot water production in residential buildings
- DeepSolar: focused on solar-assisted space heating and hot water production in
residential buildings; - DeepValve: focused on zone-level space heating in offices
DeepHot and DeepSolar frameworks are evaluated using real-world hot water use behavior of occupants, and DeepValve is experimentally implemented in the environmental chamber of the ICE lab. Results show promising energy saving potentials of each control framework.
Background
- Occupant behavior is highly stochastic, and is unique in each building
- Current controls rely on expert knowledge and cannot easily integrate occupant behavior
- Learning controls can autonomously learn and adapt to occupant behavior to reduce energy use in buildings
Methodology
- Collecting data on hot water use behavior in 3 Swiss houses
- Develop and evaluate DeepHot and DeepSolar frameworks in simulation using real-world data
- Develop and evaluate DeepValve framework in simulation using real-world data from other studies
- Experimentally implement DeepValve in the ICE environmental chamber
Goals
- Develop self-learning control frameworks to integrate occupant behavior in building controls
- Test the frameworks in simulation using real-world data of occupant behavior
- Test the frameworks experimentally in the environmental chamber of the ICE lab
Results
- A significant energy reduction can be achieved by integrating occupant behavior
- Energy reduction is achieved without compromising health and comfort of occupants
- Self-learning control can also coordinate stochastic renewable energy use and occupant behavior
Journal Publications
- RL for proactive operation of residential energy systems by learning stochastic occupant behavior and fluctuating solar energy: Balancing comfort, hygiene and energy use. A Heidari, F Maréchal, D Khovalyg. Applied Energy, 2022, V. 318, 119206
- An occupant-centric control framework for balancing comfort, energy use and hygiene in hot water systems: A model-free reinforcement learning approach. A Heidari, F Maréchal, D Khovalyg. Applied Energy, 2022, V. 312, 118833
- Adaptive hot water production based on Supervised Learning, A. Heidari, N. Olsen, P. Mermod, A. Alahi, D. Khovalyg, Sustainable Cities and Society, V. 66, 2021, 102625
- Short-term energy use prediction of solar-assisted water heating system: Application case of combined attention-based LSTM and time-series decomposition, A. Heidari, D. Khovalyg, Solar Energy, 2020, V. 207, pp. 626-639
Conference Publications
- Reinforcement learning for occupant-centric operation of residential energy system: Evaluating the adaptation potential to the unusual occupants´ behavior during COVID-19 pandemic. A Heidari, F Maréchal, D Khovalyg. CLIMA 2022
- An adaptive control framework based on Reinforcement learning to balance energy, comfort and hygiene in heat pump water heating systems. A Heidari, F Marechal, D Khovalyg. Journal of physics: Conference series 2042 (1), 012006