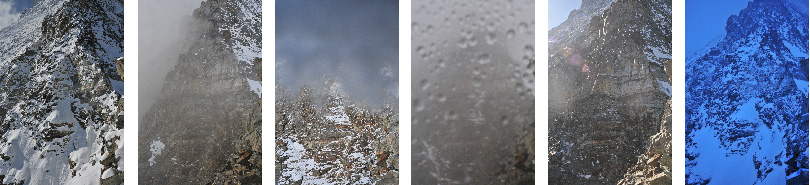
Authors
Dominic Rüfenacht, Matthew Brown, Jan Beutel, and Sabine Süsstrunk
Click here to get access to the full paper and the presentation slides.
Abstract
We propose a method for accurate and temporally consistent surface classification in the presence of sparse, noisy and irregularly sampled measurements, and apply it to the estimation of snow coverage over time. The input imagery is extremely challenging, with large variations in lighting and weather distorting the measurements. Initial snow cover estimations are obtained using a Gaussian Mixture Model of color. To achieve a temporally consistent snow-cover estimation, we use a Markov Random Field that penalizes rapid fluctuations in the snow state, and show that the penalty term needs to be quite large, resulting in slow reactivity to changes. We thus propose a classifier to separate good from uninformative images, which allows to use a smaller penalty term. We show that the incorporation of domain knowledge to discard uninformative images leads to better reactivity to changes in snow coverage as well as more accurate snow cover estimations.
Time-Lapse Videos
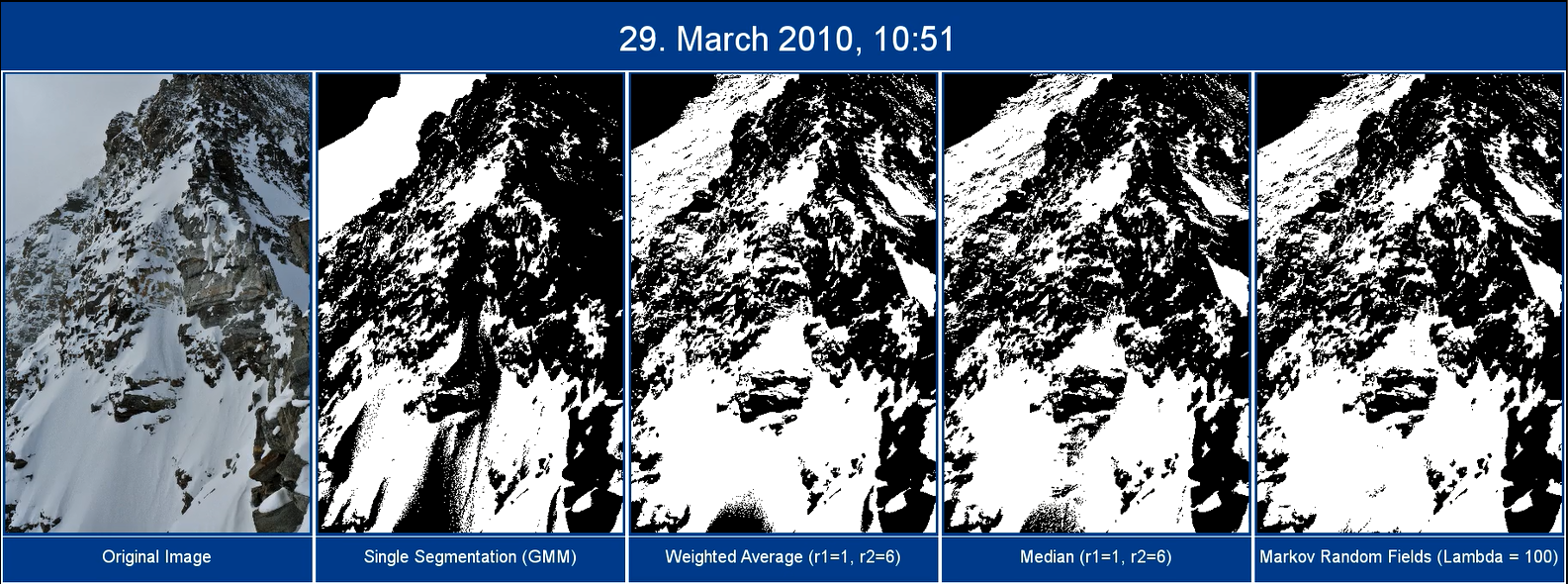
In order to evaluate temporal consistency and to compare the snow cover estimations of different methods, we created time-lapse videos for the two image sets.
- daytime (1763 images) [155 MB]
- good (659 images) [82 MB]
Some interesting events happened on the following dates (time stamps of the videos are in parentheses, for the daytime and the good images, respectively):
– 27.11.2009 (1:06, 0:34): | Major snowfall, many uninformative measurements. |
– 06.04.2010 (5:54, 2:19): | Before a major power outage. The next image was taken 14 days later. Note how the MRF is able to faster and more accurately adapt to the change in snow. |
– 29.04.2010 (6:46, 2:41): | There are too many uninformative images in the daytime set so that all methods give wrong estimates. Note that the estimates are improved for the good image set, since many of the uninformative images have been discarded. |
PermaSense Database
Link to the PermaSense project database: data.permasense.ch
Reference
Temporally Consistent Snow Cover Estimation from Noisy, Irregularly Sampled Measurements. 9th International Conference on Computer Vision Theory and Applications (VISAPP), Lisbon, Portugal, January 5-8, 2014.
, , and .Contact
Questions and remarks about this project are welcome and can be sent to [email protected].