Projects are extracted from ISA database, some delays may occur.
For additional information and project status, please send an email directly to the project contact person/assistant.
Please note that the online project status (available/taken/etc) may not be accurate.
For Internship + PDM in indusry, please contact Dr. Alireza Karimi
Directives (2014) for projects at LA
Information to add/manage projects on ISA can be found here (not official).
LA projects on ISA (Jones, Ferrari Trecate, Kamgarpour, Karimi, Salzmann)
Adaptive Model Predictive Control for Racing Cars
- ID: 13933
- Type(s): Projet de semestre MA EL
- Section(s): EL
- Status: Validé
- Professor: Giancarlo Ferrari Trecate, Daniele Martinelli
The project involves leveraging state-of-the-art optimization techniques to control a mini race-car. The focus is on navigating through unpredictable and challenging environments while ensuring that safety conditions are met. These uncertainties may come from the extern (environment) or be present due to unmodeled disturbances in the physical model.
In order to handle these uncertainties, we rely on state-of-the-art Regret Minimization controllers [1]. The control phase will be carried out using a Model Predictive Controller (MPC), allowing the system to adapt to disturbances and uncertainties in real-time.
Students will also get to work with an Optitrack system, which is important in robotics and automation applications.
Students interested in this project should have:
*Coding proficiency in Python & C++ (!!!)
*Control Theory & Optimization Techniques Knowledge(!!)
*Coding proficiency in ROS (!)
*Mechanical Knowledge to model simple physical systems (!)
—————————————————————————————————————
[1]: Martin, Andrea, et al. “Safe control with minimal regret.” Learning for Dynamics and Control Conference. PMLR, 2022.
- Comment
- FOR ANY INFO, CONTACT: [email protected]
- Professor(s)
- Giancarlo Ferrari Trecate, Daniele Martinelli
- Administration
- Barbara Marie-Louise Frédérique Schenkel
Agent-based energy management strategy
- ID: 13386
- Type(s): Projet de semestre MA EL, Projet de Master (PDM) EL
- Section(s): EL
- Status: Complet
- Professor: Maryam Kamgarpour, Maryam Kamgarpour
Modern districts are commonly equipped with PV panels, electric batteries, variable power heat pump and electric vehicles. This means local energy systems are becoming complex energy hubs which require an efficient energy management system (EMS) that can be configured in a simple way while satisfying complex needs such as improving self-consumption and reducing cost.
The energy systems group at CSEM has developed the software NRGMaestro(TM) a next-generation energy management system (EMS) that is based on Model Predictive Control (MPC) and which can be easily reconfigured through an automated controller synthesis process. This energy manager has been embedded in the commercial product Soleco Optimizer [https://soleco-optimizer.ch/] and is currently in operation in several buildings in Switzerland.
In order to scale-up to districts and eventually beyond that, centralizing all the information in one location to calculate an optimal energy dispatch is problematic for multiple reasons (privacy, computational issues, reliability). Therefore, a distributed management approach is largely preferable. The goal of this project is to design a mechanism to network local optimizing EMS in multiple buildings in order to achieve a collectively satisfactory outcome in terms of energy costs for an energy community. To this end, the intern is expected to
– Review academic literature on multi-agent systems, distributed optimization and game theory, including distributed Nash equilibrium seeking to identify promising directions,
– Implement prototypes of strategies
– Evaluate in simulation on synthetic scenarios using real-world data from different buildings equipped with modern energy devices such as electric vehicles, heat pumps, photovoltaic production, etc
While the objective of the work is clearly industrial, there will be scope to present the results in scientific journals or at conferences. The student will be part of a team of twelve experienced engineers and researchers and will face theoretical and practical challenges.
- Comment
- Requirements:
– Interest in energy systems and energy management problematics.
– Solid understanding of algorithms, in particular optimization methods
– Familiarity with some of the following topics is a plus:
o Game-theory
o Distributed algorithms
o Model predictive control
– Interest and experience in programming ( Python )
Salary: 2000 CHF/month
Type of project: Master in industry or internship, minimum 5 months. Location: Neuchatel
- Professor(s)
- Maryam Kamgarpour (Recherche en systèmes), Maryam Kamgarpour
- Administration
- Sandra de Best
- External
- Tomasz Gorecki, [email protected]
Babyfoot
- ID: 10134
- Type(s): Projet de semestre MA EL, Projet de Master (PDM) EL, Projet de semestre BA EL
- Section(s): EL
- Status: Validé
- Professor: Christophe Salzmann
The EPFL babyfoot is under continuous improvement.
While the babyfoot can easily intercept the ball and kick toward the opponent goal with success, it can also juggle and shoot if the ball is still.
The difficulty is to capture the ball, make a pass and chain precise actions. This project aims at improving the ball handling while it is moving to permit capture, pass and juggling, and then shoot toward opponents goal.
Students suggested improvements are also welcome.
The babyfoot is programmed using LabVIEW, knowledge of this language is prerequisite for strategy related projects.
- Professor(s)
- Christophe Salzmann (Laboratoire d’automatique 3)
- Site
- https://www.epfl.ch/labs/la/pi/babyfoot/
Benchmark Problem of Dual-Stage Actuator in HDD
- ID: 13748
- Type(s): Projet de semestre MA MT
- Section(s): MT
- Status: Complet
- Professor: Alireza Karimi
Background
The data capacity of the hard disk drive (HDD) must increase to meet the demands for data storage. As a result, we must improve the positioning accuracy of the magnetic head in the HDD for a better future.
Objective
To encourage research about magnetic-head positioning control, a benchmark problem which works with MATLAB has been released for the IFAC World Congress 2023 (see https://www.ifac2023.org/media-download/79/8d46c77a8e65ee38/). A model is given to simulate the magnetic-head positioning system used in the latest HDDs. The objective for students is to apply control synthesis techniques seen in the Advanced Control system course to beat the benchmark performance.
Requirements: Advanced Control Systems
- Comment
- Contact: [email protected]
- Professor(s)
- Alireza Karimi
- Administration
- Philippe Louis Schuchert
Continuous online controller improvement for a mini racecar
- ID: 13861
- Type(s): Projet de semestre MA EL
- Section(s): EL
- Status: Validé
- Professor: Colin Neil Jones, Johannes Christian Karl Waibel
Motivation:
The Automatic Control Lab develops Model Predictive Control schemes for a miniature race car system. Innovative methods will have a wide scope of applications in driving safety and/or autonomous driving.
Modeling the tire properties (longitudinal and side slip forces) turns out to be challenging for a 1/27-scale. This is due to difficulties collecting informative and consistent experimental data but also uncertainty about the physical effects that should be covered by the model structure.
Description:
The goal of this project is to continuously learn and improve the racing control performance of the mini race car while it is driving. Intuitively, we would like to imitate the situation where a driver gets to conduct a new unknown car on a new unknown race track. The driver will carefully explore the safe driving envelope of the car on the track while improving the lap time.
We want to explore different methods at the intersection between system identification, controller tuning, and machine learning. The exact approach will be discussed with the student depending on the state of the project and the student’s skills.
Skills needed
– Mechanical understanding, ideally knowledge about car dynamics
– Proficiency in C++ (for controlling the car and sensor interface)
– Proficiency in Matlab (for data analysis)
– Familiarity with Python/PyTorch (for Machine Learning)
– Significant experience in coding projects
– Familiarity with ROS/ROS2 (Robot Operating System) is a plus
– Familiarity with Eigen, Casadi is a plus (for modeling/parameter optimization)
– Optimal Control (e.g., MPC) is a plus
- Comment
- Please contact Johannes Waibel ([email protected]) if interested.
- Professor(s)
- Colin Neil Jones (Laboratoire d’automatique 3), Johannes Christian Karl Waibel
- Administration
- Nicole Anne Bouendin
Data-Driven Building Control: Simulations and Experiments
- ID: 13285
- Type(s): Projet de semestre MA EL
- Section(s): EL
- Status: Validé
- Professor: Colin Neil Jones, Christophe Salzmann
Commercial buildings consume around 40% of the world’s energy. Many research papers have explored different advanced methods to save energy. For example, model predictive control (MPC) does the job and guarantees occupancy comfort. However, there is still a large gap between the promising research results and real-world deployment. Thus, this project explores a more implement-friendly building control framework – data-driven control methods.
Current industrial practice is typically simple controllers such as bang-bang and PI controllers. To update these simple controllers to more advanced optimization/ learning-based control, such as predictive model control and reinforcement learning, additional investment in computation infrastructure is needed. One low-cost alternative solution is directly tuning those parameters of existing simple controllers to optimize the building performance. For example, Bayesian optimization has been demonstrated to be effective in tuning building controllers. This project could explore more experimental work on Bayesian optimization-based building controller tuning. A building testbed in the EPFL campus, Polydome (shown in the image below), is ready for actual experiments.
Requirements:
1. Proficient in Matlab or Python
2. Knowledge in optimal control or machine learning is preferred
3. Knowledge in Bayesian optimization or derivative-free optimization is a plus
- Comment
- Assistants: Jicheng Shi ([email protected]), Wenjie Xu ([email protected])
- Professor(s)
- Colin Neil Jones (Laboratoire d’automatique 3), Christophe Salzmann
- Administration
- Nicole Anne Bouendin
Data-driven output-feedback control of microgrids using bahavioral approach
- ID: 13801
- Type(s): Projet de Master (PDM) GM, Projet de semestre MA GM
- Section(s): GM
- Status: Validé
- Professor: Alireza Karimi
The large-scale integration of distributed power-electronic devices has rendered modern power systems difficult to be explicitly and accurately modeled through first principle or system identification. Meanwhile, the ubiquitous smart meters and smart sensors in power systems give us the access to a substantial amount of data. The behavioral approach, representing the system dynamics with trajectory data, lend itself to the data-driven analysis and control of complex power systems owing to non-parametric representation.
This project aims to design output-feedback controller for complex microgrids using behavioral approach. On top of some reasonable assumptions about noise, robust controllers and (or) optimal controllers are supposed to be designed. The performance of controllers will be validated and compared by simulation.
This project can either be a semester project or a master project.
- Professor(s)
- Alireza Karimi
- Administration
- Zhaoming Qin
Design of Energy Based Classifier for General Classification Tasks
- ID: 11545
- Type(s): Projet de semestre MA GM
- Section(s): GM
- Status: Validé
- Professor: Christophe Salzmann, Giancarlo Ferrari Trecate
Deep networks have become invaluable tools for supervised machine learning, e.g., they have been successfully applied in many computer vision and classification tasks. While often offering superior results over traditional techniques, deep architectures are known to be challenging to design and train such that they generalize well to new data. Important issues with deep architectures are numerical instabilities in learning algorithms commonly called exploding or vanishing gradients.
This project aims to develop a novel energy based classifier for classification purposes by employing Hamiltonian based deep neural networks (H-DNNs). These neural networks stem from the discretization of ordinary differential equations and guarantee non-vanishing gradients by design. Hamiltonian systems are attractive due to their energy-preservation properties which can result in more stable deep networks. Recently, H-DNNs have been successfully implemented for several data-sets. Figure 1 demonstrates the efficacy of standard H-DNNs and nonlinear boundaries for classification.
The project has following milestones:
1. Understanding the basic architecture of H-DNNs and literature survey (2 – 3 weeks).
2. Understanding the implementation of energy classifier for binary classification data-sets (as per Figure 1) as a head-start (4 – 5 weeks).
3. Implementation of energy classifiers on Python (pytorch) for standard data sets, i.e. multi-class peaks, and MNIST (3 – 4 weeks).
4. Simulation comparisons with baselines on the basis of robustness against adversarial attacks and validation accuracy (1 – 2 weeks).
5. Report writing (1 – 2 weeks).
6. (Bonus) Testing the classifier for more challenging data-sets, for instance, CIFAR-10.
Requirements: System and Control Theory, Machine Learning, experience with Python.
- Comment
- Assistants: Clara Galimberti ([email protected]) and Muhammad Zakwan ([email protected])
- Professor(s)
- Christophe Salzmann (Laboratoire d’automatique 3), Giancarlo Ferrari Trecate
- Administration
- Nicole Anne Bouendin
- Site
- https://www.epfl.ch/labs/la/pi/
Distributed Control of Microgrids
- ID: 13800
- Type(s): Projet de semestre MA MT
- Section(s): MT
- Status: Complet
- Professor: Alireza Karimi, Vaibhav Gupta
The proposed project aims to analyse the dynamics of a microgrid with multiple sources, including renewables (such as solar and wind) and backup sources (such as batteries or generators). The primary objective is to develop a comprehensive understanding of the microgrid’s behaviour under varying conditions and propose an efficient control algorithm to optimise its operation. This will be achieved through real-time simulations using Simulink and MATLAB.
- Comment
- Assistant: Vaibhav Gupta
- Professor(s)
- Alireza Karimi, Vaibhav Gupta
- Administration
- Sandra de Best
- Site
- ddmac.epfl.ch, la.epfl.ch
Distributed Neural Control of Robotarium
- ID: 12762
- Type(s): Projet de semestre MA GM
- Section(s): GM
- Status: Validé
- Professor: Giancarlo Ferrari Trecate, Christophe Salzmann
Deep neural networks have proved successful in many application domains, such as image recognition, language comprehension, and sequential decision making. However, recently, neural networks are being employed for the control of non-linear dynamical systems.
In this project, our goal is to employ distributed neural networks for the control of interconnected systems while guaranteeing the closed-loop stability. Particularly, we will consider Hamiltonian deep neural networks for distributed control problems, for instance, formation, consensus, and coverage control with collision avoidance. Moreover, we will test our neural networks on the state-of-the-art platform called Robotarium that provides a remotely access to swarm robotics.
https://www.robotarium.gatech.edu
- Comment
- Assistant: Muhammad Zakwan ([email protected])
- Professor(s)
- Giancarlo Ferrari Trecate, Christophe Salzmann
- Administration
- Barbara Marie-Louise Frédérique Schenkel
- External
- [email protected]
- Site
- https://www.epfl.ch/labs/la/pi/
Hamiltonian deep neural network controllers: A free parametrization approach
- ID: 13927
- Type(s): Projet de semestre MA GM
- Section(s): GM
- Status: Validé
- Professor: Giancarlo Ferrari Trecate, Christophe Salzmann
Deep neural networks have proved successful in many application domains, such as image recognition, language comprehension, and sequential decision-making. However, recently, neural networks have been successfully employed for the control of non-linear dynamical systems.
In this project, our goal is to employ a class of distributed neural networks called Hamiltonian deep neural network controllers for the control of autonomous systems while guaranteeing closed-loop stability. For instance, one possible application is learning to navigate a fleet of robots through a cluttered environment while avoiding obstacles. We will test our neural network controllers on the state-of-the-art platform called BRAX. It is a general-purpose physics engine that aims to facilitate research and development in robotics, biomechanics, graphics and animation, machine learning, and other areas that demand fast and accurate simulation of articulated structures interacting with their environment.
- Comment
- For more information please contact.
TA: Muhammad Zakwan
email: [email protected]
- Professor(s)
- Giancarlo Ferrari Trecate, Christophe Salzmann
- Administration
- Nicole Anne Bouendin
- Site
- https://www.epfl.ch/labs/la/pi/
Identification and Control system Design and Implementation on a 3 Degree of Freedom Hover
- ID: 10839
- Type(s): Projet de semestre MA MT
- Section(s): MT
- Status: Validé
- Professor: Alireza Karimi, Vaibhav Gupta
In this project, the objective is first identify a model for a 3-Degree-Of-Freedom (3DOF) hover based on conventional techniques in system identification. To do so, first, a code to run the system using LABVIEW should be written and deployed on MyRIO.
Moreover, all the electrical connections should also become compatible for running the system.
The code for system identification should be flexible to select different excitation such as PRBS, white noise, single tone sinusoidal and sin-sweep. The second part of the project includes designing a controller using the methods of advanced control such as H-infinity and data-driven method and then applying on the device using an appropriate labview code and validate the performance.
- Comment
- Assistant: Vaibhav Gupta
- Professor(s)
- Alireza Karimi, Vaibhav Gupta
- Administration
- Isabelle Stoudmann Schmutz
- Site
- la.epfl.ch
Implementation and deployment of sensor fusion and control algorithms on embedded hardware for a miniature hovercraft
- ID: 13806
- Type(s): Projet de semestre MA EL
- Section(s): EL
- Status: Validé
- Professor: Colin Neil Jones, Roland Schwan
Motivation:
In the previous semesters, we build a lightweight hovercraft platform capable of hovering on an air-hockey table. The long-term goal of the project would be the autonomous play against humans, or having multiple hovercrafts playing air hockey autonomously against each other.
Description:
In this project, we want to develop and deploy sensor fusion (Kalman filter) and control algorithms onboard the microprocessor of the hovercraft (ESP32). For this, you will build upon our existing micro-ROS implementation running on FreeRTOS. We have data from the onboard sensors (accelerometer, velocity sensor, and gyroscope) which have to be fused with delayed external position measurements (Optitrack) to obtain a high-frequency state estimate. Using this state estimate, the aim is to develop an onboard low-level controller tracking trajectories given from an offboard computer.
Skills needed:
– Excellent knowledge of C/C++
– Knowledge about Control Systems is a plus
– Familiarity with ROS/ROS2 is a plus
If interested, please contact the responsible assistant directly ([email protected]) and attach your transcripts and CV.
- Professor(s)
- Colin Neil Jones (Laboratoire d’automatique 3), Roland Schwan
- Administration
- Nicole Anne Bouendin
Koopman Operator based robust control of nonlinear systems
- ID: 13937
- Type(s): Projet de semestre MA GM
- Section(s): GM
- Status: Validé
- Professor: Alireza Karimi
The goal of the project is to study an application of the Koopman operator based control approach. For autonomous systems, Koopman operator theory shows that a nonlinear system can be represented as a linear system in a higher dimensional (possibly infinite dimensional) space. Thus, by lifting a nonlinear system to a higher dimensional space this approach enables the use of tools from linear systems theory for studying nonlinear systems. By using the Koopman operator approach, our goal is to obtain a linear system representation in a high dimensional space and employ linear control design techniques to control the actually nonlinear system.
The lifted system representation will be obtained in a data-driven fashion. As a result of this as well as further structural restrictions, the lifted representation never exactly captures the original system dynamics. Thus, the project will also aim for bounding these errors such that guarantees for the true closed-loop system can be provided by using robust control tools.
For now a pendulum system is considered as the application.
Skills needed:
-Advanced control systems (familiarity with frequency domain approach and robust control)
-Matlab/Simulink, LabVIEW
- Comment
- Contact: [email protected]
- Professor(s)
- Alireza Karimi
- Administration
- Mert Eyuboglu
- Site
- ddmac.epfl.ch, la.epfl.ch
Learning to maximize the social welfare from human preferences
- ID: 13928
- Type(s): Projet de Master (PDM) EL
- Section(s): EL
- Status: Validé
- Professor: Maryam Kamgarpour, Anna Maria Maddux
Motivation: Shared decision-making is at the heart of any multi-agent system. Each agent independently aims to maximize its reward which depends on the decisions of all agents. The outcome of
such independent decision-making, however, is often inefficient in terms of social welfare and may violate other central objectives such as fairness. In traffic routing, for example, each driver aims to minimize its travel time independently which can result in traffic jams. Traffic routing under a central authority, however, can lead to a reduction in the aggregate travel time of all drivers. The field of mechanism design is concerned with how a central authority can incentivize independent decision-making towards an outcome that is aligned with the social welfare, possibly by incurring a cost on each agent. A key challenge is that the social welfare function can be complex and taking into account several possibly conflicting objectives, and may thus be a priori unknown. To address this, this master project aims to incorporate feedback from the central authority to learn a social welfare function that is aligned with the central authority’s preferences.
- Comment
- Requirements: We seek for motivated students with a strong mathematical, or computer science background. We do have some concrete ideas on how to tackle the above challenges, but we are always open for different suggestions. If you are interested, please send an email containing 1. one paragraph on your background and fit for the project, 2. your BS and MS transcripts to [email protected] and [email protected].
- Professor(s)
- Maryam Kamgarpour (Recherche en systèmes), Anna Maria Maddux
- Administration
- Sandra de Best
- Site
- https://www.epfl.ch/labs/sycamore/student-projects/
Meta-Planning and Optimal Control to Play a Game of Air Hockey Autonomously
- ID: 13862
- Type(s): Projet de Master (PDM) EL
- Section(s): EL
- Status: Validé
- Professor: Colin Neil Jones, Roland Schwan
Motivation:
In previous semesters, we build a lightweight hovercraft platform capable of hovering on an air-hockey table, developed a software/hardware stack based on ROS2, identified the system dynamics using machine learning techniques, and implemented some rudimentary control algorithms to position the hovercraft on the table freely. This project aims at tieing everything together to achieve optimal play against a human or another robot/hovercraft.
Description:
In this project, we want to achieve full autonomous play. For this, we need to develop a meta-planning algorithm which calculates a strategy to where, when, and how to hit the puck, playing it back optimally hitting the enemy goal. This will involve the interplay between optimal decision-making and optimal control, giving a good mix of mathematical modeling, algorithm development, software engineering work, and making things work in simulation and a real hardware platform. Additionally, there is the possibility to incorporate machine learning tools like Gaussian processes to learn and correct for potential model mismatch in real-time, and/or using Bayesian optimization for iterative performance tuning of hyperparameters.
Skills needed:
– Excellent knowledge of C/C++
– Knowledge in Optimal Control (MPC)
– Autonomy and creativity to come up with solutions on your own
– Familiarity with ROS/ROS2 is a plus
If interested, please contact the responsible assistant directly ([email protected]) and attach your transcripts and CV.
- Professor(s)
- Colin Neil Jones (Laboratoire d’automatique 3), Roland Schwan
- Administration
- Nicole Anne Bouendin
Model-Free Safe Exploration and Optimization
- ID: 11559
- Type(s): Projet de Master (PDM) GM, Projet de semestre MA GM
- Section(s): GM
- Status: Validé
- Professor: Christophe Salzmann, Giancarlo Ferrari Trecate
Traditionally, people accomplish control design through modelling of the system along with the environment and optimizing based on the models. However, this procedure is costing more and more efforts due to the increasing complexity of realistic tasks. In some applications like surveillance, emergency rescue and autonomous driving [1], we have no chance to obtain accurate models of both the robot and the environment it is interacting with. Consequently, it is always necessary to explore, safely and efficiently, to collect data for long-term optimality.
In this project, we look into the optimal operation of a distribution power grid [2] with unknown parameters and utilises Bayesian Optimization (BO), based on Gaussian Process Regression, to safely converge to the optimum [3]. You might have the following tasks:
1. In BO and Safe Exploration, usually certain regularity conditions are assumed for the system and the environment. It is important to consider how to tailor these conditions specifically for our task.
2. Compare BO with other model-free methods, like Extremum Seeking and Safe RL, over several aspects like safety, suboptimality, efficiency and robustness.
3. There could be some random process involved, like process noise of the actuators. Evaluate how the uncertainty affects the performance of the algorithm. Hopefully, your algorithm can keep the system safe even when there are unpredictable components.
You are welcome to discuss the project by sending an email to [email protected]
Reference:
[1] Lu, Yimeng, and Maryam Kamgarpour. “Safe mission planning under dynamical uncertainties.” 2020 IEEE International Conference on Robotics and Automation (ICRA). IEEE, 2020.
[2] Karagiannopoulos, Stavros, et al. “Operational planning of active distribution grids under uncertainty.” IREP 2017, X Bulk Power Systems Dynamics and Control Symposium. 2017.
[3] Turchetta, Matteo, Felix Berkenkamp, and Andreas Krause. “Safe exploration for interactive machine learning.” arXiv preprint arXiv:1910.13726 (2019).
- Professor(s)
- Christophe Salzmann (Laboratoire d’automatique 3), Giancarlo Ferrari Trecate
- Administration
- Nicole Anne Bouendin
- Site
- la.epfl.ch
Multi-robot Trajectory Planning Under Traffic Interactions
- ID: 13931
- Type(s): Projet de Master (PDM) GM, Projet de semestre BA GM
- Section(s): GM
- Status: Validé
- Professor: Maryam Kamgarpour, Kai Ren
1. Overview
This project focuses on developing trajectory planning solutions for autonomous systems in interactive environments. The goal is to create algorithms enabling each robot to safely reach its destination while navigating complex scenarios, such as multiple autonomous cars at non-signalized intersections or multi-robot systems requiring formation control and coordination. Key challenges include collision avoidance with other agents and obstacles. The methodologies will be implemented and tested on an Nvidia Jetbot multi-robot testbed.
2. Responsibilities
– Literature review of existing trajectory planning methods within the frameworks of stochastic optimal control and game theory.
– Implementing the chosen control algorithms on the robotic testbed.
– Evaluating the algorithms for optimality, safety, and computational efficiency.
– Developing new algorithmic approaches for multi-robot trajectory planning based on the needs of the problem.
3. Requirements
– Familiarity with ROS/ROS2 and independence in trouble-shooting.
– Proficiency in Python for algorithm development and system integration.
– The project requires strong academic performance in control courses including model predictive control (MPC).
- Comment
- Note: To apply, send an email to [email protected] including 1. one paragraph on your background and fit for the project, 2. your BS and MS transcripts. This project will be supervised by Prof. Maryam Kamgarpour and Kai Ren ([email protected]).
- Professor(s)
- Maryam Kamgarpour (Recherche en systèmes), Kai Ren
- Administration
- Sandra de Best
NN Control for Mini Race Cars
- ID: 13819
- Type(s): Projet de semestre MA GM
- Section(s): GM
- Status: Validé
- Professor: Giancarlo Ferrari Trecate, Daniele Martinelli
Motivation
Mini race car systems provide a compact yet realistic platform for testing and refining autonomous driving algorithms. In this project, we aim to control these vehicles using neural networks (NN).
The student will be able to improve the following skills:
* Neural Network Integration (Incorporating neural networks into control real systems).
* Sensor Fusion (Integrating various sensors for data processing).
* Safety Implementation (Implementing safety measures in autonomous systems).
* Project Management (Planning, executing, and managing progress).
* Innovation (Exploring creative solutions for advanced technology).
Description
This project aims to use NN for mini race car control, focusing on autonomy and safety. Through advanced algorithms and model training, we aspire to create an adaptive system that not only optimizes speed and agility but also ensures safety guarantees.
Skills needed (in decreasing order of importance)
* Mechanical understanding, ideally knowledge about car dynamics
* Proficiency in ROS/ROS2
* Familiarity with Python/PyTorch (for Machine Learning)
* Familiarity with Matlab (for data analysis)
* Significant experience in coding projects
* Eigen, Casadi knowledge is a plus (for modeling/parameter optimization)
* Optimal Control (e.g., MPC) is a plus
- Professor(s)
- Giancarlo Ferrari Trecate, Daniele Martinelli
- Administration
- Barbara Marie-Louise Frédérique Schenkel
Neural Network-powered safe control of Autonomous Systems in MuJoCo
- ID: 13828
- Type(s): Projet de semestre MA GM
- Section(s): GM
- Status: Validé
- Professor: Giancarlo Ferrari Trecate, Christophe Salzmann
Deep neural networks have proved successful in many application domains, such as image recognition, language comprehension, and sequential decision-making. However, recently, neural networks have been successfully employed for the control of non-linear dynamical systems.
In this project, our goal is to employ a class of distributed neural networks called recurrent equilibrium networks (RENs) for the control of autonomous systems while guaranteeing closed-loop stability. For instance, one possible application is learning to navigate a fleet of robots through a cluttered environment while avoiding obstacles. We will test our neural network controllers on the state-of-the-art platform called MuJoCo. MuJoCo stands for Multi-Joint dynamics with Contact. It is a general-purpose physics engine that aims to facilitate research and development in robotics, biomechanics, graphics and animation, machine learning, and other areas that demand fast and accurate simulation of articulated structures interacting with their environment.
- Comment
- TA : Muhammad Zakwan ( [email protected])
- Professor(s)
- Giancarlo Ferrari Trecate, Christophe Salzmann
- Administration
- Nicole Anne Bouendin
- Site
- https://www.epfl.ch/labs/la/pi/
No-regret learning in games with coupling constraints
- ID: 13930
- Type(s): Projet de semestre MA EL, Projet de Master (PDM) EL
- Section(s): EL
- Status: Validé
- Professor: Maryam Kamgarpour, Anna Maria Maddux
Shared decision-making is at the heart of any multi-agent system. Each agent aims to maximize its reward which depends on the decisions of all agents by repeatedly playing an action and observing the corresponding reward. A common performance measure in such repeated games is regret and several learning algorithms exist which attain no-regret. The global decision of all agents, however, may be subject to constraints and thus such independent decision-making may result in an infeasible outcome. Coupling constraints arise in several real-world problems such as electricity markets, traffic routing, and multi-robot applications. In electricity markets, for example, there are capacity constraints on the load of each grid line. In multi-robot applications in unexplored environments, the robots have to accomplish a task while avoiding collision with obstacles and other robots. For games with coupling constraints it is crucial to develop learning algorithms that not only aim at minimizing agents regret but also ensure that agents learn to play a feasible outcome. To address this, this project aims to develop a no-regret learning algorithm that simultaneously ensures constraint satisfaction.
- Comment
- In a first step, you will start by familiarizing yourself with the relevant literature on no-regret learning (see e.g. [1]) and on learning in games with constraints (see e.g. [2,3]). Next, the goal is to design a no-regret algorithm that ensures constraint satisfactions and provide theoretical guarantees for the developed algorithm. Depending on your own interest and background you can then address coupling constraints in Markov games, a class of repeated games with transition dynamics. Alternatively you focus on practical implementations of your developed algorithm for applications such as electricity markets, shared mobility systems (see e.g. [4]) and traffic routing.
Requirements: We seek for motivated students with a strong mathematical, or computer science background. We do have some concrete ideas on how to tackle the above challenges, but we are always open for different suggestions. If you are interested, please send an email containing 1. one paragraph on your background and fit for the project, 2. your BS and MS transcripts to [email protected].
- Professor(s)
- Maryam Kamgarpour (Recherche en systèmes), Anna Maria Maddux
- Administration
- Sandra de Best
- Site
- https://www.epfl.ch/labs/sycamore/student-projects/
Nonlinear Control for Spaceborne Hybrid Micro-disturbance Isolation Platform
- ID: 13966
- Type(s): Projet de semestre MA MT
- Section(s): MT
- Status: Validé
- Professor: Alireza Karimi, Elias Sebastian Klauser
Novel-type high-precision optical instruments used for Earth observation missions require a very high pointing accuracy. Line-of-sight stability requirements constrain the admissible level of mechanical vibration on board spacecraft. Micro-disturbances are a phenomenon caused by substantial satellite systems such as reaction wheels, thrusters, cryocoolers or solar array drive mechanisms. The term micro-disturbance refers to mechanical vibration or disturbance with low amplitude, typically occurring at frequencies from 1 Hz up to 1 kHz. These disturbances can substantially degrade the performance of sensitive payloads. A hybrid active-passive micro-disturbance isolation platform has been developed at CSEM Neuchâtel in the scope of an ESA-funded PhD thesis. The modular demonstration platform consists of an adjustable number of passive dampers, a set of proof mass actuators (PMA) creating a 6 DoF force tensor and an interface allowing to carry different types of payloads. A rapid prototyping platform is used for testing and optimization of different control algorithms. Each PMA contains a moving mass attached to a membrane behaving in a nonlinear manner below its resonance frequency. This behaviour makes it difficult to use the PMA below its resonance frequency.
The goal of the semester project is to provide a controller for disturbance rejection using the PMA at low frequencies. A feedforward strategy was implemented in the scope of a Master project to linearise the dynamics of the PMA. An extended Kalman filter (EKF) is applied for state estimation. The goal is now to combine this feedforward controller with a feedback disturbance rejection loop. Afterwards, testing campaign shall be performed to assess and validate the performance of the demonstration platform operating below the resonance frequency of the PMA.
During the semester project, the student will be partly working in the Sensing & Control laboratory at CSEM Neuchâtel.
- Professor(s)
- Alireza Karimi, Elias Sebastian Klauser
- External
- CSEM Neuchâtel
- Site
- https://www.csem.ch/ScientificInstrumentation
Online controller adaptation for a thrust-vector-controlled rocket
- ID: 13830
- Type(s): Projet de semestre MA EL
- Section(s): EL
- Status: Validé
- Professor: Colin Neil Jones, Johannes Christian Karl Waibel
Motivation:
The EPFL Rocket Team aims for building thrust-vector-controlled rockets that can be reused by controlling them to land upright at a specific location. We develop algorithms that guide and control the rocket during the descent phase, approach, and touchdown.
Description:
In this project, we would like to develop algorithms for safely adapting drone and rocket controllers online, i.e., during the flight.
First, we will establish a baseline method, i.e., an adaptation approach based on conventional model identification/controller design. One approach could be to recursively fit a linear model and update controller gains based on it.
Second, we will develop a Machine Learning method that tunes the existing controller, and/or models a physical submodule, and/or directly approximates a feedforward control law. Eventually, we will compare the performance of the two developed methods.
Skills needed:
– Understanding of flight mechanics, modeling, and identification
– Classical and/or Optimal Control (LQR/MPC)
– Machine Learning methods (Neural Networks/Gaussian Processes)
– Familiarity with Python/PyTorch (for Machine Learning)
– Experience in C++ (for operating the drone)
– Significant experience in coding projects
– Familiarity with ROS (Robot Operating System) is a plus
- Comment
- Please contact Johannes Waibel ([email protected]) if interested.
- Professor(s)
- Colin Neil Jones (Laboratoire d’automatique 3), Johannes Christian Karl Waibel
- Administration
- Nicole Anne Bouendin
Project Title: Renewable Risk Management in Power Grids
- ID: 13942
- Type(s): Projet de semestre MA EL, Projet de Master (PDM) EL
- Section(s): EL
- Status: Validé
- Professor: Giancarlo Ferrari Trecate, Jean-Sébastien Hubert Brouillon
The surge in global renewable electricity generation, aimed at combating climate change, poses significant physical and financial risks to the power grid. This project aims to assess potential losses resulting from uncertainties in power generation from renewable sources. Power producers currently grapple with the challenge of accurately predicting the capacity of all their generators in advance to meet established targets. The unpredictability and volatility in renewable power production can be addressed by incorporating excess capacity in renewable production, energy storage, and transmission infrastructure, albeit at a considerable expense. Although a spot market provides some flexibility every 15 minutes, it also often involves higher costs. This tradeoff must be explored in order to find optimal operational strategies.
The student will work on a simplified academic example, incorporating a battery, a cost-effective but limited generator, and an expensive peak generator. These flexible assets will be connected to variable loads and solar panels and controlled using Model Predictive Control (MPC) to match supply and demand over the following day(s).
The project is expected to provide valuable insights into the effectiveness of different optimization strategies in managing the uncertainties associated with renewable energy production. The findings may contribute to the development of more robust and cost-efficient renewable energy integration strategies within power grids. The candidate for this project should possess good knowledge of power systems and optimization, have experience with Python programming, and ideally be familiar with the “cvxpy” package for optimization tasks would be appreciated.
- Professor(s)
- Giancarlo Ferrari Trecate, Jean-Sébastien Hubert Brouillon
- Administration
- Barbara Marie-Louise Frédérique Schenkel
- Site
- https://la.epfl.ch/pi
Reward learning from human feedback
- ID: 13929
- Type(s): Projet de Master (PDM) EL
- Section(s): EL
- Status: Validé
- Professor: Maryam Kamgarpour, Andreas Schlaginhaufen
Reinforcement Learning (RL) is a promising approach to many complex decision making problems. However, one of the key challenges in applying RL to real-world scenarios is determining an appropriate reward function for the problem at hand. To alleviate this, RL from human preferences [1] aims to use human feedback to learn a reward function that is aligned with human preferences. To this end, the algorithm is iteratively asking human experts for their preferences between pairs of optimal demonstrations corresponding to different reward functions and then uses this new information to update its reward function. RL from human preferences has proven to be successful in simulation, and has recently also been applied to fine tuning of large language models [2].
In a previous master project, RL was used to teach robots to build a self-supporting structure connecting two points, for example a bridge. Determining a reward function for this task turns out to be difficult. However, human feedback may be valuable for telling whether a given demonstration is close to achieving the goal of building a stable bridge or not.
- Comment
- Requirements: We seek for motivated students with a strong mathematical, or computer science background. We do have some concrete ideas on how to tackle the above challenges, but we are always open for different suggestions. If you are interested, please send an email containing 1. one paragraph on your background and fit for the project, 2. your BS and MS transcripts to [email protected].
This project will be supervised by Prof. Maryam Kamgarpour ([email protected]), Anna Maddux ([email protected]), and Andreas Schlaginhaufen ([email protected]).
- Professor(s)
- Maryam Kamgarpour (Recherche en systèmes), Andreas Schlaginhaufen
- Administration
- Sandra de Best
- Site
- https://www.epfl.ch/labs/sycamore/student-projects/
Robust Data-Driven Control of Identical Dynamically Coupled Systems
- ID: 11546
- Type(s): Projet de semestre MA GM
- Section(s): GM
- Status: Validé
- Professor: Christophe Salzmann, Giancarlo Ferrari Trecate
Optimal control using data is increasing interest of both academic and industrial communities. In comparison with conventional model-based control, data- driven control has the advantage that it can be applied in scenarios where data is readily available, but the system and uncertainty models are too complex to obtain or maintain, e.g., distributed systems (large-scale power systems) or energy efficient buildings, etc. Data-driven techniques are expected to be more adaptive and reactive to changing environment and uncertainties. Usually, a mathematical model of the plant is not available and thus a model needs to be identified from a set of experimental data and then the controller is designed using this identified model. Such a 2-step or indirect data-driven procedure has been proven to lead to potentially sub-optimal controllers. Moreover, the model that best fits the data is not necessarily also the most suitable for controller tuning.
Recently, behavioral modelling (BM) is proposed for the control design of the linear time-invariant systems. This result, known as the Willem’s fundamental lemma, shows that the subspace of input/output trajectories of a linear time- invariant (LTI) system can be obtained from the column span of a data Hankel matrix, thereby avoiding a parametric system representation. Multiple direct data-driven control methods have been proposed based on BM.
The goal of this project is to develop data-driven distributed control of linear time-invariant (LTI) identical dynamically coupled systems known as decomposable systems. These systems result from the interconnection of a large number of identical subsystems, for example, multi-agents, vehicle platoons, etc. If the state-space matrices of these systems satisfy a certain structural property, then it is possible to derive a data-driven procedure by leveraging tools from behavioral modelling for designing a distributed controller which has the same interconnection pattern as the plant. Figure 1 illustrates the collection of data to design the controller, whereas, Figure 2 demonstrates the implementation of a distributed controller for a swarm of mobile wheel robots.
The project has following milestones:
1. Literature survey spanning distributed systems, behavioral modelling, and semi-definite programs (2 – 3 weeks).
2. Derive the tractable stability conditions in terms of data-based linear matrix inequalities (LMIs) (3 – 4 weeks).
3. Develop simulations (for academic \& practical applications) on MATLAB using YALMIP (2 – 3 weeks).
4. Report writing (1 – 2 weeks).
5. (Bonus) Extension to other classes of distributed systems, e.g. LPV decomposable systems.
- Comment
- Assistant: Muhammad Zakwan ([email protected])
- Professor(s)
- Christophe Salzmann (Laboratoire d’automatique 3), Giancarlo Ferrari Trecate
- Administration
- Nicole Anne Bouendin
- Site
- https://www.epfl.ch/labs/la/pi/
Safe reinforcement learning from single agent to multi-agent
- ID: 13825
- Type(s): Projet de semestre MA EL, Projet de Master (PDM) EL
- Section(s): EL
- Status: Validé
- Professor: Maryam Kamgarpour, Tingting Ni
Reinforcement Learning (RL) involves studying sequential decision-making problems, where an agent aims to maximize an expected cumulative reward by interacting with an unknown environment. While RL has achieved impressive success in domains like video games and board games, safety concerns arise when applying RL to real-world problems, such as autonomous driving, robotics, power systems, and cyber-security. This project proposal aims to explore the field of safe RL, addressing the challenges of sample complexity, stability, and theoretical guarantees.
Outline: This project contains the following potential directions:
Designing Safe RL Algorithms: Explore and propose approaches for safe reinforcement learning that address the challenges of sample complexity, stability, and safety guarantees.
Theoretical Advancements: Advance the theoretical foundations of safe RL algorithms, focusing on a specific class of RL methods, such as policy-based methods, actor-critic methods, or other relevant approaches. Develop provable guarantees for the safety and stability of these algorithms.
Implementation and Evaluation: Implement the proposed safe RL algorithms in a realistic simulation environment. Evaluate their performance and compare them against existing methods using safety benchmarks.
Moving from single agent setting to multi-agent setting.
- Comment
- Requirement: We seek for motivated students with a strong mathematical, or computer science background. We do have some concrete ideas on how to tackle the above challenges, but we are always open for different suggestions. If you are interested, please send an email containing 1. one paragraph on your background and fit for the project, 2. your BS and MS transcripts to [email protected]. The students who have suitable track record will be contacted.This project will be supervised by Prof. Maryam Kamgarpour and Tingting Ni ([email protected]).
- Professor(s)
- Maryam Kamgarpour (Recherche en systèmes), Tingting Ni
- Administration
- Sandra de Best
- Site
- https://www.epfl.ch/labs/sycamore/safe-reinforcement-learning-from-single-agent-to-multiagent/
Slip-aware modeling/control for a mini racecar
- ID: 13826
- Type(s): Projet de semestre MA EL
- Section(s): EL
- Status: Refusé
- Professor: Colin Neil Jones, Johannes Christian Karl Waibel
Motivation:
The Automatic Control Lab develops Model Predictive Control schemes for a miniature race car system. Innovative methods will have a wide scope of applications in driving safety and/or autonomous driving.
Modeling the tire properties (longitudinal and side slip forces) turns out to be challenging for a 1/27-scale. This is due to difficulties collecting informative and consistent experimental data but also uncertainty about the physical effects that should be covered by the model structure.
Description:
The goal of this project is to establish a high-fidelity model of the mini race car by developing new modeling approaches, conducting experiments for data collection, and fitting the models to the data.
One way to improve the data quality will be to integrate an IMU and/or wheel speed sensors into the cars. Given the small size, this requires a careful component selection and mechanical design of attachments/wiring/etc.
A second approach can be to use Machine Learning to describe additional physical effects.
The obtained model will be used in simulation and ideally for model-based control that seizes the newly-gained tire information. Promising controllers will be tested on the real racetrack in return.
Skills needed
– Mechanical understanding, ideally knowledge about car dynamics
– Proficiency in C++ (for controlling the car and sensor interface)
– Proficiency in Matlab (for data analysis)
– Familiarity with Python/PyTorch (for Machine Learning)
– Significant experience in coding projects
– Familiarity with ROS/ROS2 (Robot Operating System) is a plus
– Familiarity with Eigen, Casadi is a plus (for modeling/parameter optimization)
– Optimal Control (e.g., MPC) is a plus
- Comment
- Please contact Johannes Waibel ([email protected]) if interested.
- Professor(s)
- Colin Neil Jones (Laboratoire d’automatique 3), Johannes Christian Karl Waibel
- Administration
- Nicole Anne Bouendin
Swarm control of mini-hovers
- ID: 13947
- Type(s): Projet de semestre MA EL, Projet de semestre BA EL
- Section(s): EL
- Status: Validé
- Professor: Alireza Karimi, Christophe Salzmann
In this project, the objective is to deploy a swarm of mini-hovers (Crazyflies) using ROS and a hierarchical control scheme. The drones position would be then measured using a motion capture system (OptiTrack) which would be used for swarm controls. The student would need to: 1. Design a controller synthesis procedure for low-level controls of each drone. 2. Integrate the Crazyflie into the ROS architecture. 3. Design a high-level controller for swarm dynamics.
- Comment
- Assistant: Gupta Vaibhav
- Professor(s)
- Alireza Karimi, Christophe Salzmann
- Administration
- Barbara Marie-Louise Frédérique Schenkel
- Site
- ddmac.epfl.ch, la.epfl.ch
Swarm control of mini-hovers
- ID: 13944
- Type(s):
- Section(s): MX
- Status: Validé
- Professor: Alireza Karimi, Vaibhav Gupta
In this project, the objective is to deploy a swarm of mini-hovers (Crazyflies) using ROS and a hierarchical control scheme. The drones position would be then measured using a motion capture system (OptiTrack) which would be used for swarm controls. The student would need to: 1. Design a controller synthesis procedure for low-level controls of each drone. 2. Integrate the Crazyflie into the ROS architecture. 3. Design a high-level controller for swarm dynamics.
- Professor(s)
- Alireza Karimi, Vaibhav Gupta
- Administration
- Barbara Marie-Louise Frédérique Schenkel
- Site
- ddmac.epfl.ch, la.epfl.ch
System identification and control design on a 2DOF hover
- ID: 13835
- Type(s): Projet de semestre MA GM
- Section(s): GM
- Status: Validé
- Professor: Alireza Karimi
The first goal of this project is to identify a model of the Quanser Aero 2 – 2 DOF Hover using conventional system identification methods. For this, different input signals and model structures will be considered. Matlab/Simulink environment will be used for the data collection and system identification.
The second goal of the project is to design a controller and implement it on the real system. After the identification process the nonlinearity of the system will be evaluated and a proper controller design strategy will be determined accordingly. If the effect of nonlinearities are observed to be small, an LTI controller with a model based or data-driven approach can be designed. Otherwise, a nonlinear controller using the Koopman operator approach can be synthesized. The designed controllers will be implemented in Simulink and tested on the real system.
- Comment
- Contact: [email protected]
- Professor(s)
- Alireza Karimi
- Administration
- Mert Eyuboglu
- Site
- https://www.epfl.ch/labs/ddmac/student-projects/
Time optimal control of a miniature hovercraft
- ID: 13805
- Type(s): Projet de semestre MA EL
- Section(s): EL
- Status: Validé
- Professor: Colin Neil Jones, Roland Schwan
Motivation:
In the previous semesters, we build a lightweight hovercraft platform capable of hovering on an air-hockey table. The long-term goal of the project would be the autonomous play against humans, or having multiple hovercrafts playing air hockey autonomously against each other.
Description:
In this project, we want to steer the hovercraft time-optimally to a given state (position, velocity) to intercept the puck and play it back optimally. For this, we aim to use advanced control algorithms like non-linear time optimal model predictive control to steer the hovercraft at its physical limits. For this, you will develop and deploy high-performance numerical optimization algorithms capable of running in real-time, building upon our existing ROS2 software stack.
Skills needed:
– Excellent knowledge of C/C++
– Knowledge about Optimal Control (MPC)
– Familiarity with ROS/ROS2 is a plus
If interested, please contact the responsible assistant directly ([email protected]) and attach your transcripts and CV.
- Professor(s)
- Colin Neil Jones (Laboratoire d’automatique 3), Roland Schwan
- Administration
- Nicole Anne Bouendin
Tuning Hyperparameters of a QP Solver
- ID: 13824
- Type(s): Projet de semestre MA EL
- Section(s): EL
- Status: Validé
- Professor: Colin Neil Jones, Roland Schwan
We recently developed and released a high-performance quadratic program (QP) solver based on a proximal interior-point method [1]. While performing well with default settings/hyperparameters, we are interested in finding more optimal and robust default hyperparameters and problem/domain specific hyperparameters to achieve better performance.
Bayesian optimization (BO) is a sample-efficient black-box optimization method, which has been successfully applied to tune the hyperparameters of machine learning models [2]. This project aims to apply the recently proposed BO (e.g., constrained BO [3]) methods to tune the hyperparameters of a QP solver.
Requirements:
– Proficient in Matlab or Python
– Knowledge in optimization or machine learning is preferred
– Knowledge in Bayesian optimization or quadratic programming is a plus
If interested, please contact the responsible assistants directly ([email protected], [email protected]) and attach your transcripts and CV.
References:
[1]: Schwan, R., Jiang, Y., Kuhn, D., & Jones, C.N. (2023). PIQP: A Proximal Interior-Point Quadratic Programming Solver. In IEEE Conference on Decision and Control.
[2]: Snoek, J., Larochelle, H., & Adams, R. P. (2012). Practical Bayesian optimization of machine learning algorithms. Advances in Neural Information Processing Systems, 25.
[3]: Xu, W., Jiang, Y., Svetozarevic, B., & Jones, C.N. (2023, July). Constrained efficient global optimization of expensive black-box functions. In International Conference on Machine Learning (pp. 38485-38498). PMLR.
- Comment
- Assistants: Roland Schwan ([email protected]), Wenjie Xu ([email protected])
- Professor(s)
- Colin Neil Jones (Laboratoire d’automatique 3), Roland Schwan
- Administration
- Nicole Anne Bouendin
Industrial projects (not yet on ISA)
To address climate change properly and accelerate energy transition from fossil fuels to renewable energy, new energy generation technologies are required. They have to be reliable, efficient, scalable and provide sufficient low-cost energy to satisfy current and future demands. Airborne Wind Energy (AWE) is an emerging technology that involves autonomous tethered aircraft to produce electricity from high altitude winds.
Skypull can offer a solution and proposes an innovative approach, based on a new revolutionary type of flight device, aimed at maximizing the lift, with minimum resistance increase and allowing autonomous operation: a “box” drone with proles multi-element aerodynamics, characterized by high aerodynamic performance, intrinsic structural strength, low weight and low production costs.
In order to keep the drone operational over prolonged periods of time it is critical to recharge the onboard electronics during operation. Skypull developed the technology of regenerative differential braking, which allows the controlled braking of the aircraft while simultaneously regenerating energy.
Goal
In order to better understand the process of differential braking our drone model needs to be extended to accommodate for the braking process. The models focus will be on the drivetrain (motor controller, motor and propeller), but should be integrated in the drone model.
Based on this model a set of control laws will be developed to enable the drone to brake, turn and regenerate energy in a stable and robust way.
Responsibility
* Literatue/Skypull technology review
* Installation of a test bench
* Implementation of the drive train model and integration into drone model
* Derivation of control strategy for differential braking
* Testing on Skypull hardware
Requirements
* Good understanding of Simulink / MATLAB
* Good fundamental knowledge in control system design and modelling
* Basic knowledge of aerodynamics and aircraft actuators
* Basic knowledge of motor controllers
* Ability to work independently, proactive personality and highly motivated
Professor:Colin Jones
Type of Project: Master in Industry
Industrial contacts
Andrea Pedrioli Simulation Engineer, [email protected]
Aldo Cattano Chief Technology Officer, [email protected]
Skypull SA, via alla Stampa 49, 6967 Lugano
Literature
* Loyd M. L., “Crosswind kite power”, Journal of Energy 4(3) 106-111 (1980).
* Kathryn E. Johnson , “Adaptive Torque Control of Variable Speed Wind Turbines”
The energy systems group at CSEMis developing innovative technologies at the intersection of power and energy, control, and data science. In the march towards a fully- decarbonised energy system, we are developing control solutions to make buildings and grids more energy-efficient and more flexible. The current state of the art is model- predictive control to optimize the operating conditions of distributed energy resources. However its practical deployment is hindered by the time and effort required to build models of these resources, and by the inability of most models to reflect the impact of occupants on the energy performance of buildings.
In this context, we are offering an internship on the development of artificial-intelligence algorithms. The objective of the project is to build on state-of-the-art algorithms in deep reinforcement learning to create robust and optimal control strategies. Such algorithms include deep Q-networks (DQN, first demonstrated by Deepmindin 2013 to play Atari games), deep deterministic policy gradient (DDPG, first introduced by Google Deepmindin 2015 for simple motion control tasks), and proximal policy approximation (PPO, introduced by Open AI in 2017 for humanoid running and steering).
The physical nature of the control problems in energy management raises some specific challenges for these algorithms, especially in terms of convergence. The project will therefore combine theoretical aspects, with an opportunity to improve some of the most advanced general-purpose artificial intelligence algorithms, and practical aspects involving the rapid prototyping of software architectures and the implementation of algorithms.
While the objective of the work is clearly industrial, its nature means there will be scope to present the results in scientific journals or at conferences. The student will be part of a team of twelve experienced engineers and researchers.
Professor:Colin Jones
Type of Project: Master in Industry
Contacts: Pierre-Jean Alet, Baptiste Schubnel
Autonomous driving technologies that aim for more safety, comfort, and environmentally friendly vehicles, have been growing rapidly in automotive industry recently. These systems go beyond vehicle-centric assistant applications by integrating traffic and environment information. One of the main challenges in autonomous driving development is to design and then validate the planning and control algorithms in a closed-loop fashion, where both vehicle dynamics characteristics and a wide variety of traffic scenarios are taken into account. The designs should also guarantee optimal performance toward precise tracking, and time/fuel optimality with respect to both vehicle and traffic constraints (i.e. avoid pedestrians and other cars).
One of the main innovation objectives of Siemens PLM is to accelerate the research and development activities of ADAS (advanced driver assistance systems) and autonomous driving technologies. We are continuously looking for outstanding students who are eager to do their Master thesis or internship on a challenging research project in a highly dynamic and international research environment. We have a variety of possible projects available that cover different aspects of planning and control algorithms, ranging from very theoretical to practical. The company also provides various tools to support the research activities, for example, Imagine.Lab Amesim for vehicle dynamics modelling, PreScan for sensor (camera, lidar, radar,…) and traffic environment modelling, and a miniature race car setup for embedded control implementation.
Examples of possible (but not limited to) topics: optimal control and learning control for an ADAS application (i.e. roundabout crossing, valet parking), fast embedded optimal control algorithm, optimal path/motion planning, simultaneous localization and mapping (SLAM) in virtual environment, vehicle dynamics, traffic planning and verification…
Background: control systems, robotics, computer science, or mathematics, familiar with programming. Experience with optimization, vehicle dynamics, ROS or autonomous vehicles is a plus.
Professor: Colin Jones
Type of project: Master
Contact: Colin Jones or Dr. Son Tong
![]() |
The work will be performed in collaboration with Siemens PLM Software in Leuven, Belgium. |
Houses are commonly equipped with PV panels, electric batteries, variable power heat pump and electric vehicles. This means single homes are becoming complex energy hubs which require an efficient energy management system (EMS) that can be configured in a simple way while satisfying complex needs such as improving self-consumption and reducing cost.
The energy systems group at CSEM is actively developing a next-generation energy management system that is based on Model Predictive Control and which can be easily reconfigured through an automated controller synthesis process. A first internship successfully contributed to this project with work focused on forecasting. In this context, we are offering an internship to improve the operation of this EMS by extending its functionalities, focusing on two aspects:
- The refinement of the predictive control algorithm with specification of complex objective such as peak shaving in the EMS algorithm, and the scale-up to multi-home systems.
- The buildup of a simulation environment that allows to testbench the controller. The simulation will rely on the versatile physical simulation environment Modelica, for which a collection of house models has already been developed. It will be used to assess the controller performance over long periods of time.
While the objective of the work is clearly industrial, there will be scope to present the results in scientific journals or at conferences. The student will be part of a team of twelve experienced engineers and researchers, and have the opportunity to use real data from test sites and face actual practical challenges of software deployment for energy applications.
Requirements:
- A strong interest for energy systems and energy management problematics.
- Good programming experience, preferably with exposure to Python; and an interest in good coding practices (object-oriented programming, unit testing, version tracking with Git).
- Familiarity with MPC or numerical optimization preferred, knowledge of Kalman filtering and/or machine learning a plus.
Type of project: Master in industry or internship, minimum 4 months.
Location: Neuchatel
Contact: Tomasz Gorecki, [email protected]
Professor: Colin Jones, [email protected]
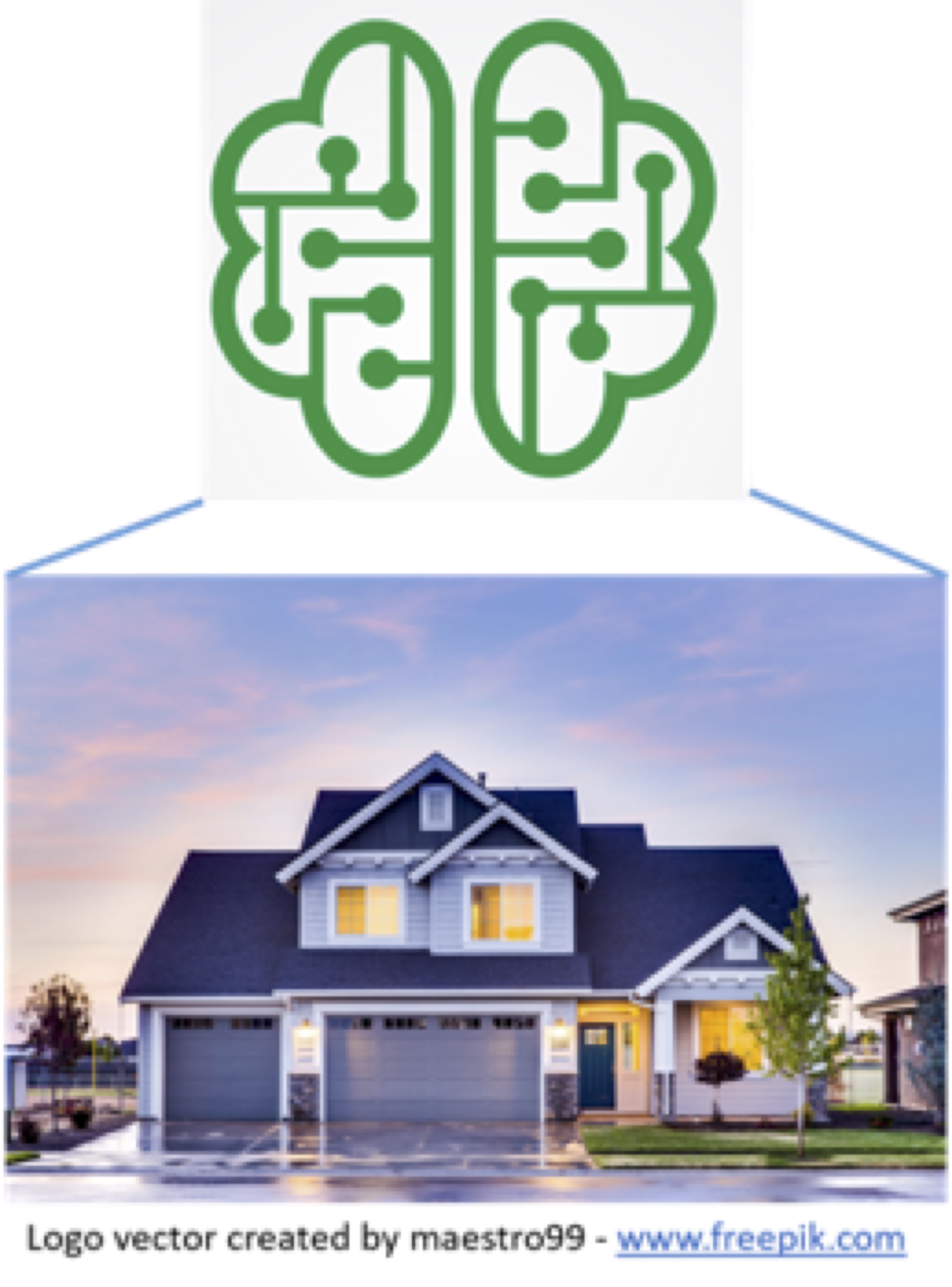
Past projects (not yet ready)
Projects 2018
Projects 2017
Projects 2016
Projects 2015
Projects 2014
Projects 2013
Projects 2012