Background
In our recent study, we demonstrated that light propagation inside multimode optical fibers can be utilized as nonlinear, high-dimensional data transform units and thanks to their rich physical dynamics, machine learning tasks could be performed with a much smaller dependence on digital electrical hardware (such as GPUs)1. This phenomenon holds promise to alleviate the consequences of overparameterization, which allowed neural networks to perform extraordinarily complicated tasks, but has the downsides such as high energy consumption and exponential increase in the need of digital computation resources.
For achieving competitive performance levels with the proposed optical computation approach, the reconfiguration of the system for the planned task is crucial. In another study, we have shown that with wavefront shaping and by optimizing a few tens of parameters, the reconfiguration of the system could improve the performance of the optical computer significantly2. Figure 1 illustrates 31% increase in test accuracy with reconfiguration, while using >1000 times less parameters than an equivalently performing digital neural network. However, the optimization follows a blackbox approach and limited in the number of programming parameters that can be controlled.
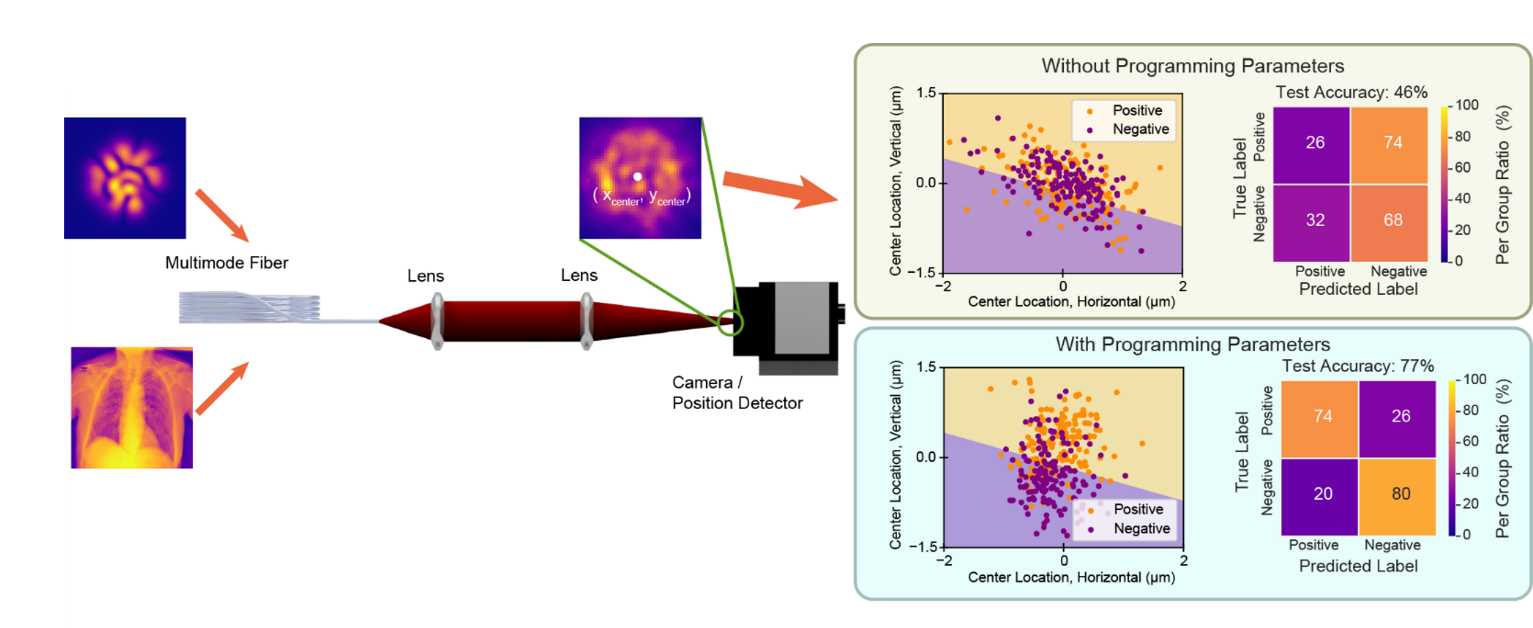
Project Description
The goal of this project is to implement a model-based optimization approach, which should allow reconfiguration of the computation with arbitrary functions and degrees of freedom. To achieve this goal, the project follows two main steps, firstly a new experimental setup with a high-speed spatial light modulator will be built. The placement and synchronization of the device will require optical alignment and instrumentation control. Secondly, the new system will be used for collecting experimental results, real-time training and fine-tuning of a digital neural network model of the experiment. This model then will enable us to find optimal parameters for reconfiguring the optical system. During this part, the main focus will be on building and training digital neural networks.
For detailed information please contact Ilker Oguz ([email protected]).
References
[1] Teğin, U., Yıldırım, M., Oğuz, İ., Moser, C. & Psaltis, D. Scalable optical learning operator. Nat. Comput. Sci. 2021 18 1, 542–549 (2021).
[2] Oguz, I. et al. Programming Nonlinear Propagation for Efficient Optical Learning Machines. Preprint at https://doi.org/10.48550/arXiv.2208.04951 (2022).