Keywords: machine learning, FLIM, microscopy, classification
Background
Head and Neck Cancers (HNC) cases approach a million diagnoses each year worldwide. Treatment of HNC involves surgical resection, which is deemed complete if the biopsy comes back from histopathology with clear (negative) margins. Positive surgical margins leave microscopic residues of cancer in the wound bed and require a second resection, as this minimal residual disease in turn gives rise to local and possible non-lac recurrences, and consecutive death of the patient. A positive surgical margin increases the risk of local recurrence by 90%, reducing the overall survival expectancy by 50% and increasing all-cause mortality at 5 years by 90% [1].
Tumor excision with negative margins, i.e., performing resections with clear pathological margins > 5 mm (R0) is the principal aim of oncology surgery. Up to 20% of patients will have a final diagnosis of positive margins despite negative intraoperative margin, due to errors in the sampling of frozen sections (the biopsy is sectioned in 2D sections to be stained and observed under the microscope), analytical error, or resection error due to the patient undergoing reconstruction surgery in between the two resections.
There is a need for a point-of-care method to map the exact tumor margins as a 3D model of the tumor before resection, and detection of residual malignant cells in the wound bed in real-time.
The tool we design is a needle-like endoscope (see Figure 1) based on a multimode fiber, which allows for deep imaging and diffraction-limited resolution using the transmission matrix method [2,3]. The contrast mechanism is based on endogenous fluorescence of the cells. Tumor cells have a different metabolism as healthy cells, which affects their fluorescent lifetime response [4].
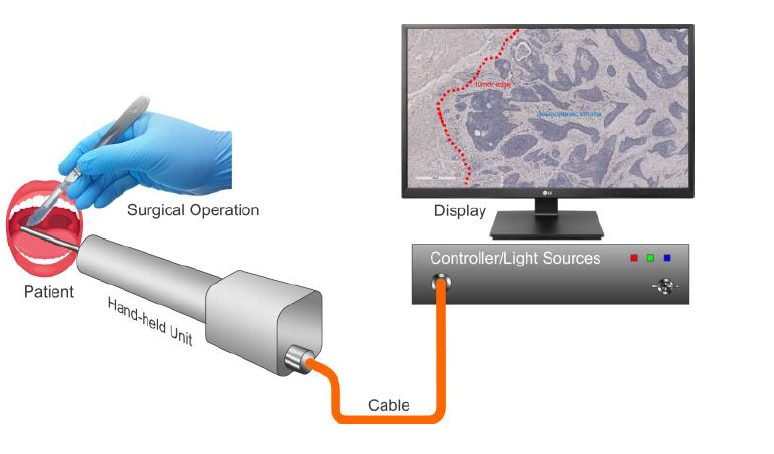
Video description of the project and description on the ISREC’s website
Project description
The role of the student in this project is to collect data using a commercial FLIM microscope and subsequent training of a machine learning algorithm to classify tumoral or healthy tissues [5]. The student will have access to the BIOP imaging facility and produce relevant data on biological samples, exploring important parameters such as the resolution and field of view and their effect on the classification abilities.
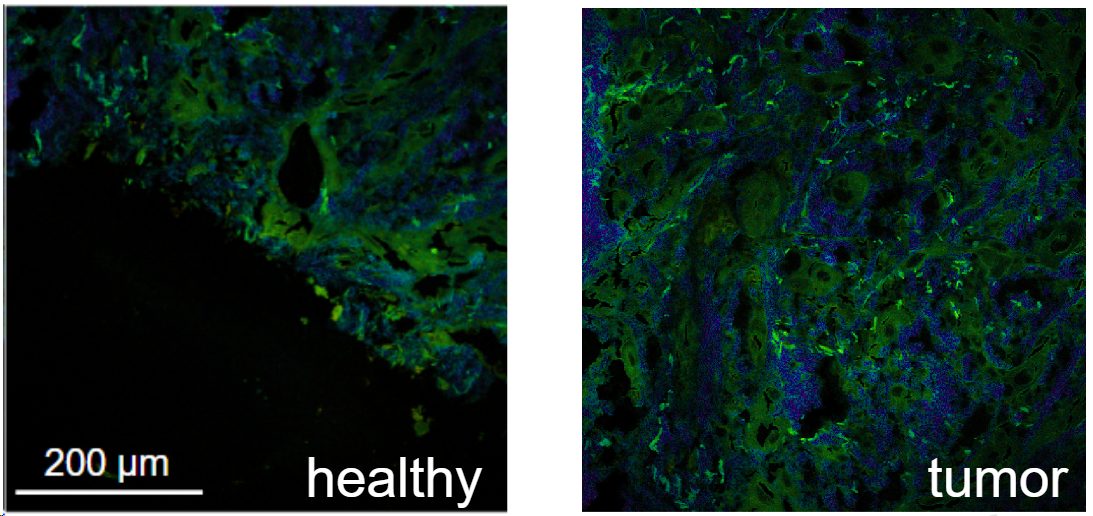
During the project, the student will have a chance to learn about FLIM and get familiar with fluorescence microscopy in general. They will gain experience with machine learning and image processing.
Key objectives are the following, and can be adapted for a semester or master project:
– Improving existing algorithm for segmentation on mouse tissue (U-Net)
– Extending classification to more categories than just cancer or normal, aiming for digital immunostaining
– Collection and classification of human tissues, comparison with mouse tissues
– Comparison of the classification performance on microscopic and endoscopic images
Student profile
The student should be interested in fluorescence microscopy, biology, and classification tasks. The student should be motivated and organized. They should have good communication skills in English as the report shall be written in that language. The student should have some knowledge of machine learning and neural networks. Previous experience with classification tasks is preferred.
Contact
If you are interested in this project, please contact me by email at: [email protected]
References
[1] Li, M. M., Puram, S. V., Silverman, D. A., Old, M. O., Rocco, J. W., & Kang, S. Y. (2019). Margin Analysis in Head and Neck Cancer: State of the Art and Future Directions. Annals of surgical oncology, 26(12), 4070–4080.
[2] Boniface, A., Dong, J. & Gigan, S. (2020). Non-invasive focusing and imaging in scattering media with a fluorescence-based transmission matrix. Nat Commun 11, 6154.
[3] Damien Loterie, Salma Farahi, Ioannis Papadopoulos, Alexandre Goy, Demetri Psaltis, & Christophe Moser. (2015). Digital confocal microscopy through a multimode fiber, Opt. Express 23, 23845-23858.
[4] Datta, R., Heaster, T. M., Sharick, J. T., Gillette, A. A., & Skala, M. C. (2020). Fluorescence lifetime imaging microscopy: fundamentals and advances in instrumentation, analysis, and applications. Journal of biomedical optics, 25(7), 1–43.
[5] Duran-Sierra, E., Cheng, S., Cuenca, R., Ahmed, B., Ji, J., Yakovlev, V.V., Martinez, M., Al-Khalil, M., Al-Enazi, H., Cheng, Y.-S.L., & et al. (2021). Machine-Learning Assisted Discrimination of Precancerous and Cancerous from Healthy Oral Tissue Based on Multispectral Autofluorescence Lifetime Imaging Endoscopy. Cancers, 13, 4751.