Currently, neural networks are implemented on electronic chips such as central processing units (CPUs) and graphics processing units (GPUs). However, computational algorithms and more specifically neural networks were also realized with optical systems even though they are far from being as popular as their electronic counterparts. In our laboratory, we demonstrated spatiotemporal nonlinearities inside multimode optical fibers can be used as a neuromorphic neural network and its performance can be comparable to digital neural networks.
As light propagates through a multimode fiber (MMF), the type used in fiber-to-the-home, the optical modes exchange power and create new optical frequencies via a complex spatiotemporal non-linear transformation that occurs at relatively low optical powers because of the light confinement and the long interaction length that is possible in a fiber. We will show recent results, obtained in collaboration with Prof. Psaltis group at EPFL, regarding programming the nonlinear interaction in MMFs for machine learning applications. Several different databases were used for recognition tasks. The classification accuracy that was obtained was comparable to deep, digitally implemented networks. The energy requirement for training and reading the optical system was orders of magnitude less than the digital counterpart.
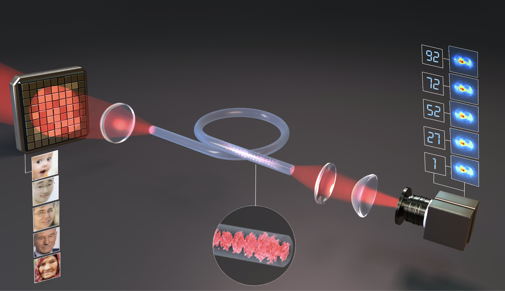