Available hemodynamical datasets have been exploited to build regression functions that map easily-obtained noninvasive measurements (e.g., peripheral pressure) to scarce central indices (e.g., central pressure) using machine learning techniques. This mapping is achieved by training a mathematical model using a data sample in order to make predictions or decisions without being explicitly programmed. In practice, the training dataset consist of an input layer (noninvasive measurements) and the corresponding output layer (central indices), which constitute the target quantity that we want to estimate. In our laboratory, we envisage the development of machine learning biomedical tools for predicting central hemodynamical indices and parameters of the contractility of the heart, determining disease-related indices and classifying disease data.
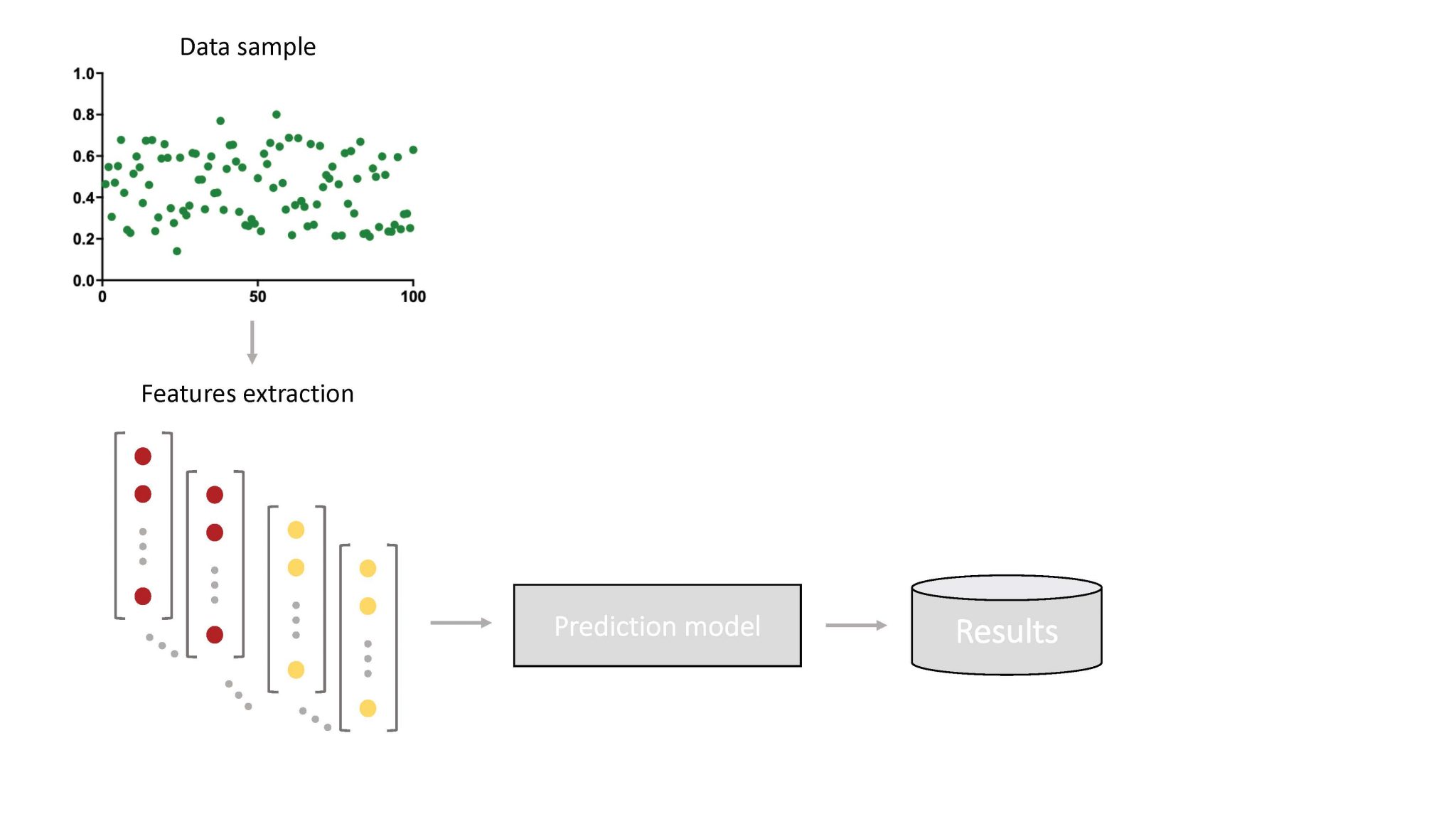