The LO has a long history of combining optics and neural networks. Several projects are currently ongoing, including the application of neural networks to “Imaging with multimode fibers” and “Optical computing”.
Imaging with mulitmode fibers using machine learning
Cylindrical glass waveguides called multimode optical fibers are widely used for the transmission of light through long distances. However, light becomes distorted in terms of spectral and spatial properties due to linear and nonlinear scatterings as it propagates inside the fiber. In LO we have demonstrated that with the help of neural networks these distortions can be learned and be corrected. Moreover, these distortions are controlled with neural networks to achieve the desired spectral and spatial properties after propagation through fiber.
Optical implementation of neural networks
Currently, neural networks are implemented on electronic chips such as central processing units (CPUs) and graphics processing units (GPUs). However, computational algorithms and more specifically neural networks were also realized with optical systems even though they are far from being as popular as their electronic counterparts. In our laboratory, we demonstrated spatiotemporal nonlinearities inside multimode optical fibers can be used as a neuromorphic neural network and its performance can be comparable to digital neural networks.
Publications :
Predicting optical transmission through complex scattering media from reflection patterns with deep neural networks
K Skarsoulis, E Kakkava, D Psaltis
Optics Communications, 126968 (2021)
Scalable Optical Learning Operator
U Teğin, M Yıldırım, I Oguz, C Moser, D Psaltis
arXiv preprint arXiv:2012.12404 (2020).
Inference in artificial intelligence with deep optics and photonics
G Wetzstein, A Ozcan, S Gigan, S Fan, D Englund, M Soljacic, C Denz, D A.B. Miller, D Psaltis
Nature 588, 39-47 (2020)
Optical neural networks : The 3D connection
NU Dinc, D Psaltis, D Brunner
Photoniques, 34-38 (2020)
Deep learning-based image classification through a multimode fiber in the presence of wavelength drift
E Kakkava, N Borhani, B Rahmani, U Teğin, C Moser, D Psaltis
Applied Sciences 10 (11), 3816 (2020)
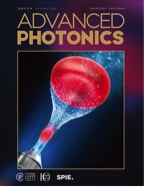
Three-dimensional tomograpy of red blood cells using deep learning
J Lim, AB Ayoub, D Psaltis
Advanced Photonics 2 (2), 026001 (2020)
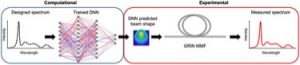
Controlling spatiotemporal nonlinearities in multimode fibers with deep neural networks
U Teğin, B Rahmani, E Kakkava, N Borhani, C Moser, D Psaltis
APL Photonics 5 (3), 030804 (2020)
Imaging through multimode fibers using deep learning: The effects of intensity versus holographic recording of the speckle pattern
E Kakkava, B Rahmani, N Borhani, U Teğin, D Loterie, G Konstantinou, C Moser, D Psaltis
Optical Fiber Technology 52, 101985 (2019)
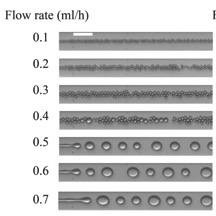
Learning from droplet flows in microfluidic channels using deep neural networks
P Hadikhani, N Borhani, SMH Hashemi, D Psaltis
Scientific reports 9 (1), 8114 (2019)
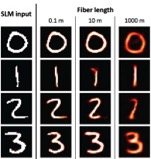
Learning to see through multimode fibers
N Borhani, E Kakkava, C Moser, D Psaltis
Optica, 5(8):960-966 (2018)
Learning tomography assessed using Mie theory
JW Lim, A Goy, MH Shoreh, M Unser, D Psaltis
Phys. Rev. Applied, 9:034027 (2018)
Learning Approach to Optical Tomography
Ulugbek S. Kamilov, Ioannis N. Papadopoulos, Morteza H. Shoreh, Alexandre Goy, Cedric Vonesch, Michael Unser and Demetri Psaltis
Optica Volume 2, Issue 6, pp. 517-522 (2015)