K. M. Jablonka, C. Charalambous, E. Sanchez Fernandez, G. Wiechers, J. Monteiro, P. Moser, B. Smit, and S. Garcia, Machine learning for industrial processes: Forecasting amine emissions from a carbon capture plant Sci Adv 9 (1), eadc9576 (2023) doi: 10.1126/sciadv.adc9576
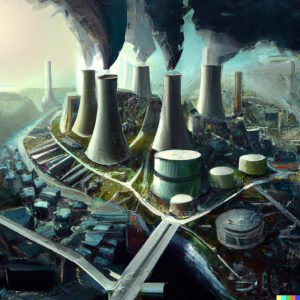
Abstract: One of the main environmental impacts of amine-based carbon capture processes is the emission of the solvent into the atmosphere. To understand how these emissions are affected by the intermittent operation of a power plant, we performed stress tests on a plant operating with a mixture of two amines, 2-amino-2-methyl-1-propanol and piperazine (CESAR1). To forecast the emissions and model the impact of interventions, we developed a machine learning model. Our model showed that some interventions have opposite effects on the emissions of the components of the solvent. Thus, mitigation strategies required for capture plants operating on a single component solvent (e.g., monoethanolamine) need to be reconsidered if operated using a mixture of amines. Amine emissions from a solvent-based carbon capture plant are an example of a process that is too complex to be described by conventional process models. We, therefore, expect that our approach can be more generally applied. Solvent emissions in a carbon capture plant are modeled and forecasted using machine learning.