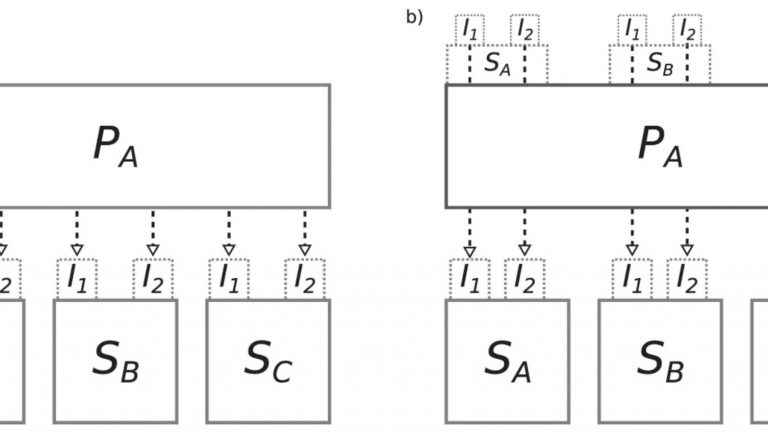
Common workflows for computing material properties using different quantum engines
S. P. Huber, E. Bosoni, M. Bercx, J. Bröder, A. Degomme, V. Dikan, K. Eimre, E. Flage-Larsen, A. Garcia, L. Genovese, D. Gresch, C. Johnston, G. Petretto, S. Poncé, G.-M. Rignanese, C. J. Sewell, B. Smit, V. Tseplyaev, M. Uhrin, D. Wortmann, A. V. Yakutovich, A. Zadoks, P. Zarabadi-Poor, B. Zhu, N. Marzari, and G. (…)
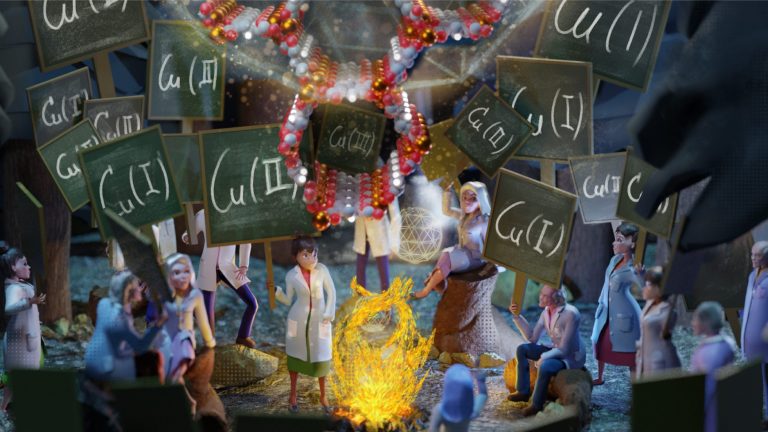
Publication in Nature Chemistry
Kevin, Daniele and Mohamad inspired by one of the life lines of “How to become a millionaire” for a model to predict oxidation states of MOFs. Interested: K. M. Jablonka, D. Ongari, S. M. Moosavi, and B. Smit, Using collective knowledge to assign oxidation states of metal cations in metal–organic frameworks Nat Chem (2021) http://dx.doi.org/10.1038/s41557-021-00717-y
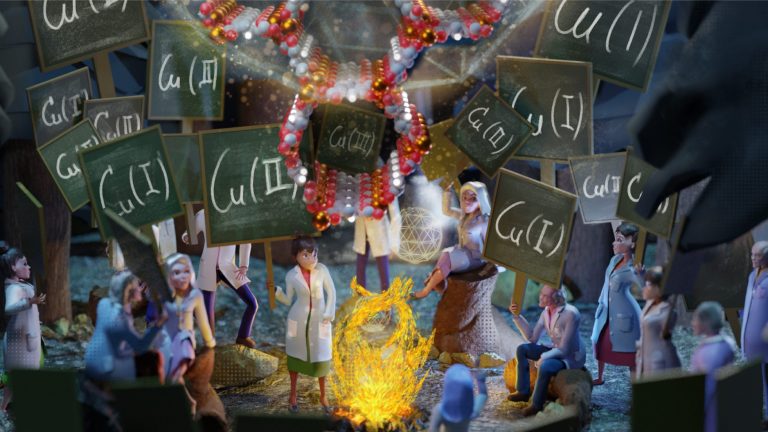
Using collective knowledge to assign oxidation states of metal cations in MOF
K. M. Jablonka, D. Ongari, S. M. Moosavi, and B. Smit, Using collective knowledge to assign oxidation states of metal cations in metal–organic frameworks Nat Chem (2021) doi: 10.1038/s41557-021-00717-y Abstract: Knowledge of the oxidation state of metal centres in compounds and materials helps in the understanding of their chemical bonding and properties. Chemists have developed (…)
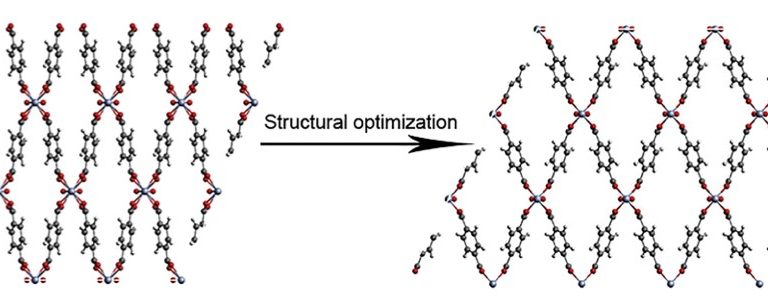
Screening MOFs for natural gas purificatiom
Z. Li, Y. Zhang, B. Liu, G. Chen, and B. Smit, Multilevel screening of computation-ready, experimental metal-organic frameworks for natural gas purification AIChE J., e17279 (2021) doi: 10.1002/aic.17279 Abstract In this study, traditional Monte Carlo simulation and density functional theory‐based structural optimization methods were combined to screen computation‐ready experimental metal‐organic framework (MOF) database for the (…)
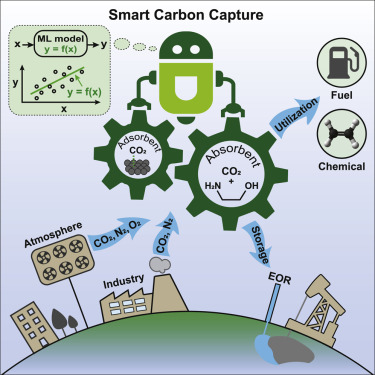
Smart Carbon Capture with Machine Learning
M. Rahimi, S. M. Moosavi, B. Smit, and T. A. Hatton, Toward smart carbon capture with machine learning Cell Reports Physical Science 2, 100396 (2021) doi: 10.1016/j.xcrp.2021.100396 Abstract: Machine learning (ML) is emerging as a powerful approach that has recently shown potential to affect various frontiers of carbon capture, a key interim technology to assist (…)
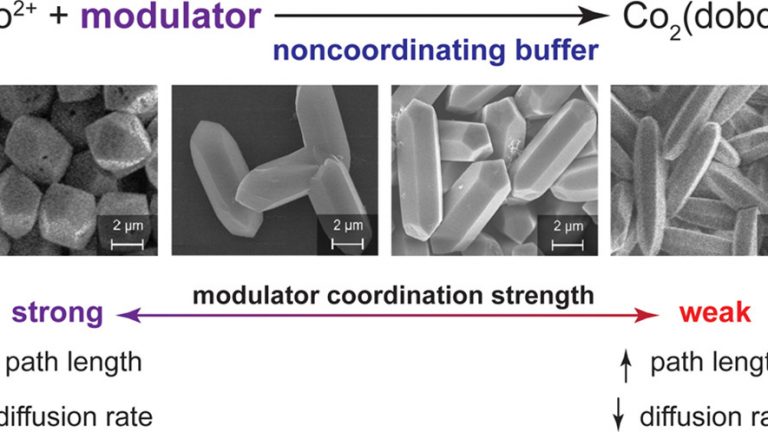
Controlling Crystal Morphology in MOFs
K. A. Colwell, M. N. Jackson, R. M. Torres-Gavosto, S. Jawahery, B. Vlaisavljevich, J. M. Falkowski, B. Smit, S. C. Weston, and J. R. Long, Buffered Coordination Modulation as a Means of Controlling Crystal Morphology and Molecular Diffusion in an Anisotropic Metal–Organic Framework J Am Chem Soc (2021) doi: 10.1021/jacs.1c00136 Abstract: Significant advances have been (…)
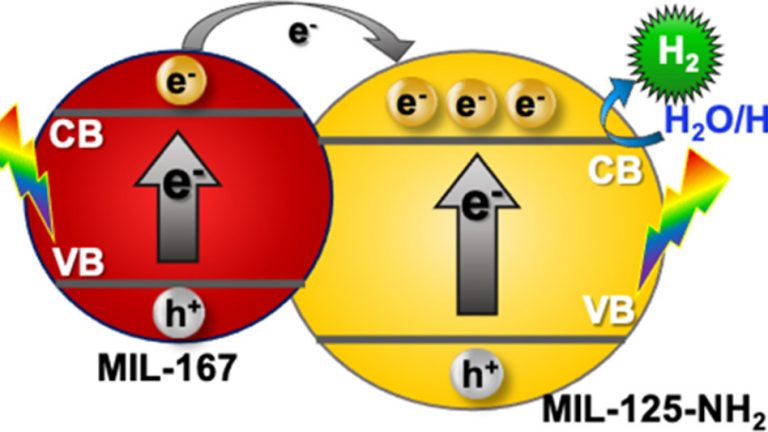
MOF/MOF Heterojunctions
S. Kampouri, F. M. Ebrahim, M. Fumanal, M. Nord, P. A. Schouwink, R. Elzein, R. Addou, G. S. Herman, B. Smit, C. P. Ireland, and K. C. Stylianou, Enhanced Visible-Light-Driven Hydrogen Production through MOF/MOF Heterojunctions ACS Appl. Mater. Interfaces (2021) DOI: 10.1021/acsami.0c23163 Abstract: A strategy for enhancing the photocatalytic performance of MOF-based systems (MOF: metal–organic (…)
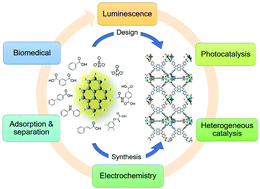
Pyrene-based MOFs
F. P. Kinik, A. Ortega-Guerrero, D. Ongari, C. P. Ireland, and B. Smit, Pyrene-based metal organic frameworks: from synthesis to applications Chem Soc Rev (2021) doi: 10.1039/D0CS00424C Abstract Pyrene is one of the most widely investigated aromatic hydrocarbons given to its unique optical and electronic properties. Hence, pyrene-based ligands have been attractive for the synthesis (…)
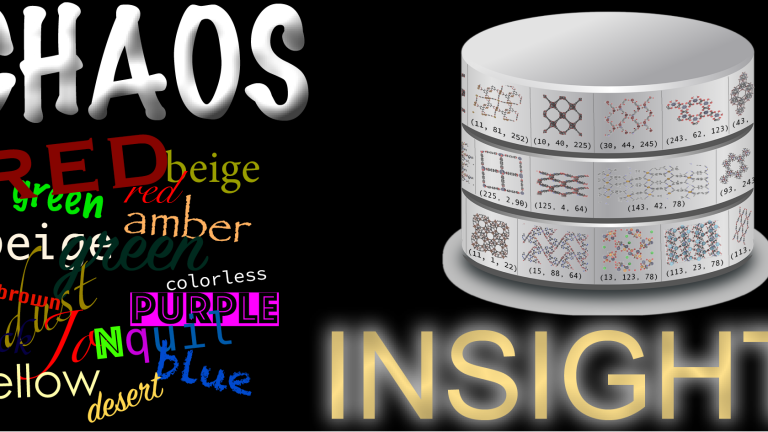
Colours of MOF
K. M. Jablonka, B. Smit, S. M. Moosavi, M. Asgari, C. Ireland, and L. Patiny, A data-driven perspective on the colours of metal-organic frameworks Chem Sci (2021) DOI: 10.1039/D0SC05337F Abstract: Colour is at the core of chemistry and has been fascinating humans since ancient times. It is also a key descriptor of optoelectronic properties of materials (…)
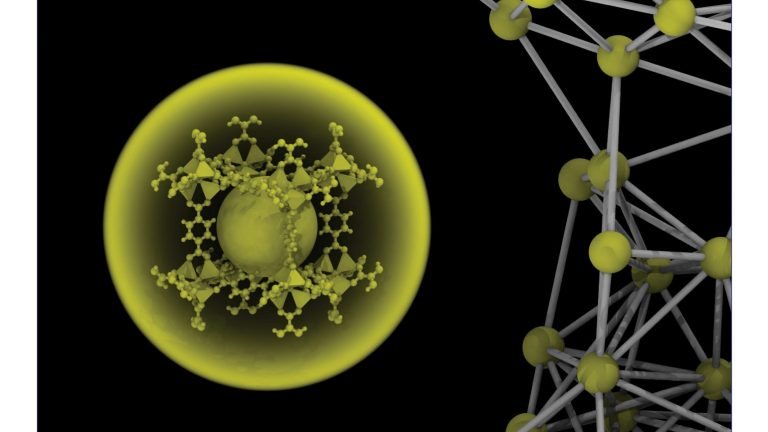
Cover of JACS
The perspective of Mohamad and Kevin on a cover of JACS. S. M. Moosavi, K. M. Jablonka, and B. Smit, The Role of Machine Learning in the Understanding and Design of Materials J Am Chem Soc (2020) http://dx.doi.org/10.1021/jacs.0c09105