Machine Learning (ML) in engineering and material science is unlocking possibilities that were once unimaginable. This new disruptive toolkit allows analyzing vast amounts of data and predicting material behaviors with unprecedented speed and accuracy. At LSMS, the synergy of advanced computation and new data-driven tools opens a new realm of possibilities in terms of the problems we can tackle and how efficiently we can do so.
Stress Distribution in Heterogeneous Materials
Heterogeneous solid materials are composed of distinct constituents exhibiting mechanical properties that vary throughout their microstructure. These materials are extensively used:
- Wood which consists of cellulose fibers embedded in a matrix of lignin.
- Metals which are often composed of small crystals with different orientations.
- Fiber-reinforced polymers which consist of a matrix and reinforcing fibers such as glass and carbon.
Simulating the deformation of these materials helps in understanding and predicting their behavior under loading. However, for complex microstructures or constituent behaviors, these computations can be slow and expensive.
Artificial Neural Networks (ANNs), when trained on datasets generated by traditional simulation methods, can provide faster and cheaper access to information about deformation and stress distribution within heterogeneous solids. The following illustration shows a U-Net ML model that is trained to predict the stress distribution in a small volume of an elastic porous material. The model captures the fine details with sufficient accuracy in under a second on a basic laptop, even with this resolution of over 2 million elements.
For additional information, please contact Lucas Fourel: [email protected]
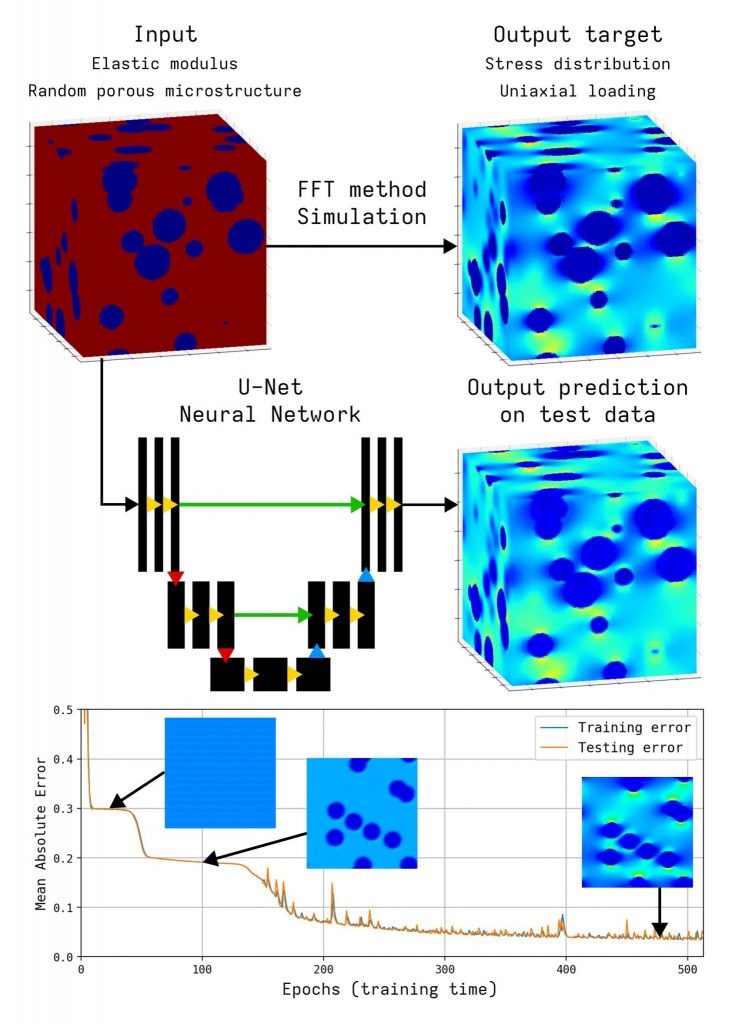
Data-Driven Computational Friction
Through the sponsorship of the Swiss National Science Foundation (via an Ambizione grant), Dr. Joaquin Garcia-Suarez is leading a project aimed at exploring new data-driven ways to model contacting surfaces in relative motion, in both engineering and geophysics. The goal is to replace traditional constitutive laws (known by their limited generalizability) through the adaptation of data-driven tools to the idiosyncrasies of the friction. In particular, we are interested in performing large-scale dynamic rupture simulations with relevance to seismology using a data-based description of the frictional response of the fault.
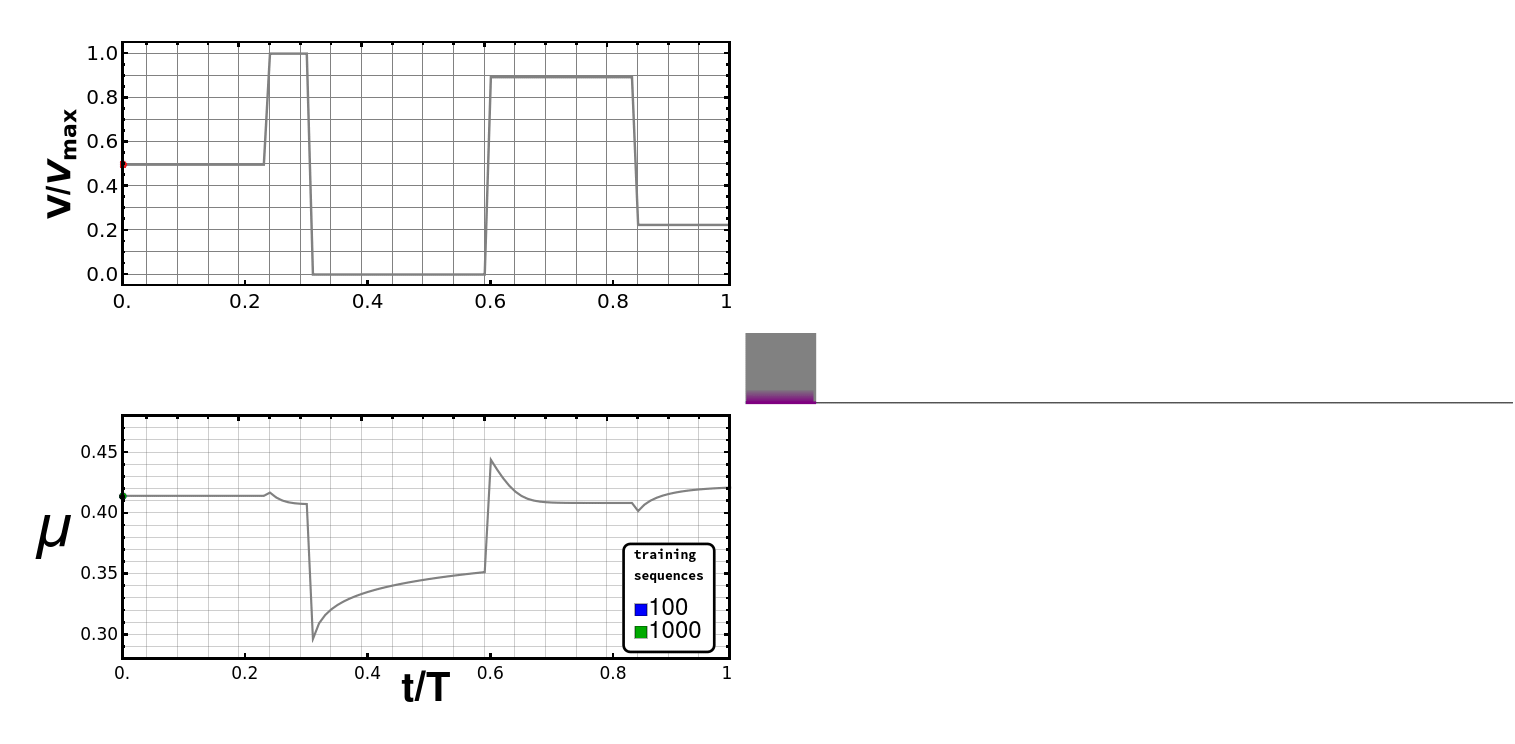