Lan Feng*, Mohammadhossein Bahari*, Kaouther Messaoud Ben Amor, Éloi Zablocki, Matthieu Cord, and Alexandre Alahi
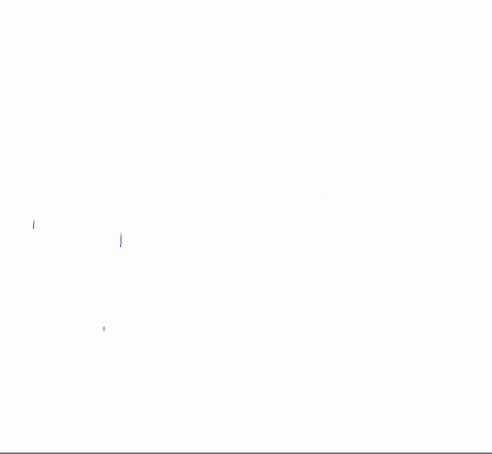
In this work, we:
- introduce UniTraj, a comprehensive open-source framework for vehicle trajectory prediction, integrating various datasets, models, and evaluations,
- investigate prediction models’ generalization across different datasets and cities and provide insight into the characteristics of datasets on which models acquire better generalization capacities,
- explore the data scaling impact on model performance employing the largest collection of datasets currently available, and establish a new state-of-the-art model on the nuScenes dataset,
- provide an in-depth comparative analysis of the datasets, shedding light on our experimental findings.
UniTraj framework
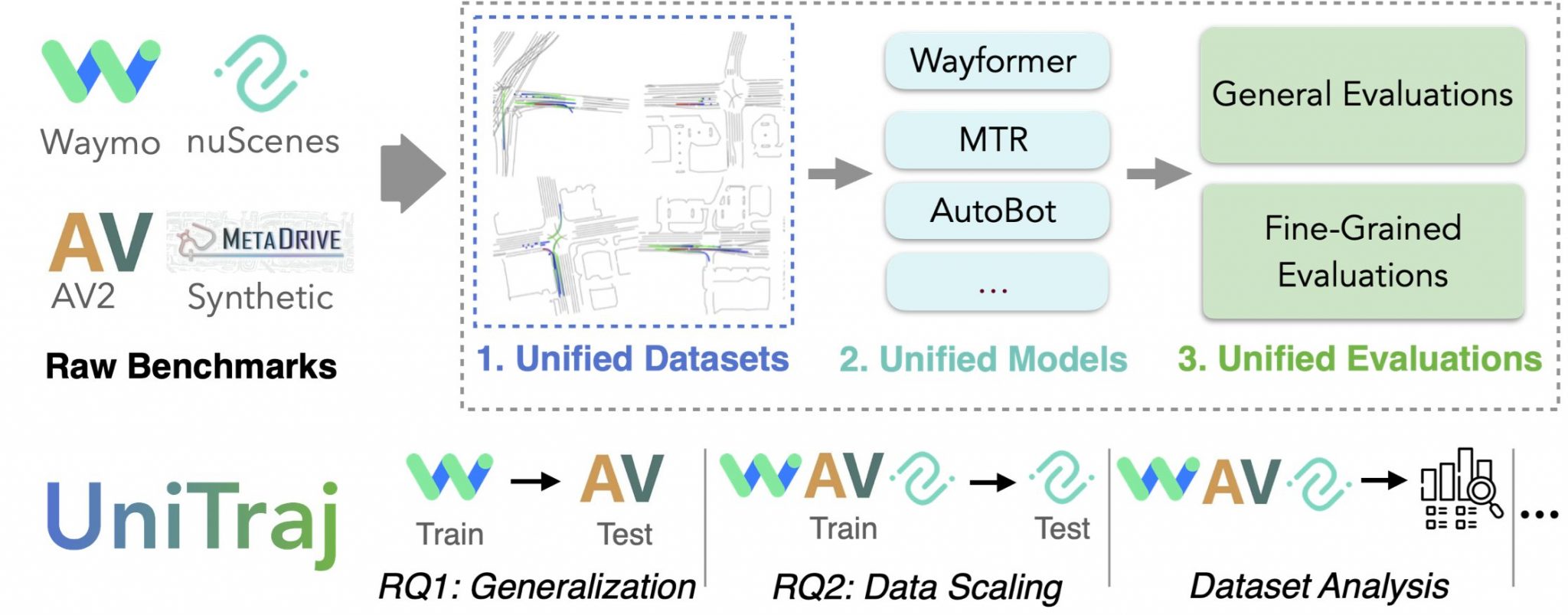
Data scaling results
Our model is top ranked in nuScenes leaderboard (01.04.2024)
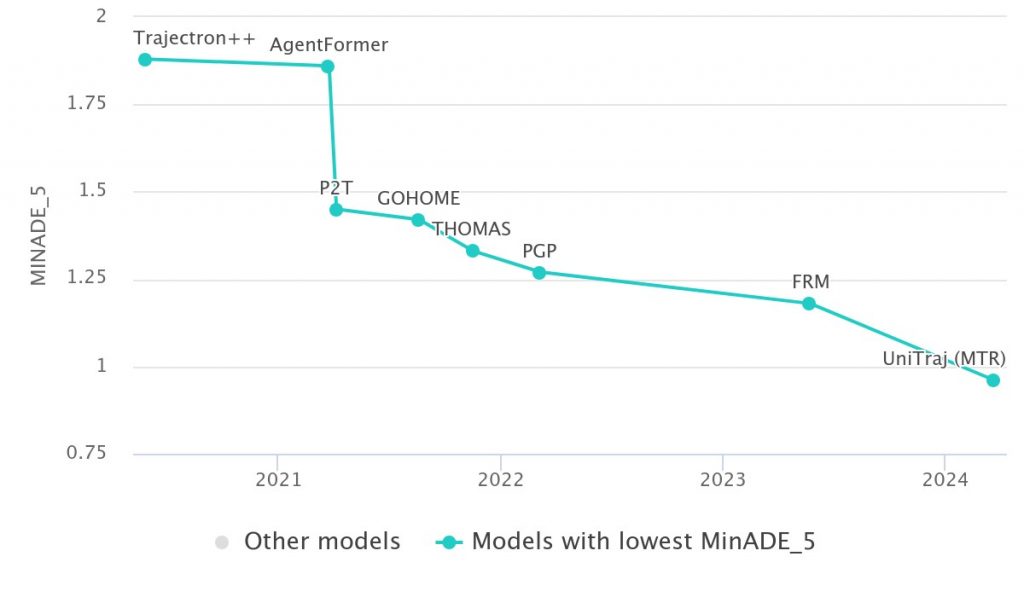
Abstract:
Vehicle trajectory prediction has increasingly relied on datadriven solutions, but their ability to scale to different data domains and the impact of larger dataset sizes on their generalization remain underexplored. While these questions can be studied by employing multiple datasets, it is challenging due to several discrepancies, e.g., in data formats, map resolution, and semantic annotation types. To address these challenges, we introduce UniTraj, a comprehensive framework that unifies various datasets, models, and evaluation criteria, presenting new opportunities for the vehicle trajectory prediction field. In particular, using UniTraj, we conduct extensive experiments and find that model performance significantly drops when transferred to other datasets. However, enlarging data size and diversity can substantially improve performance, leading to a new state-of-the-art result for the nuScenes dataset. We provide insights into dataset characteristics to explain these findings.