Every working turbine produces a downstream wind flow velocity deficit (commonly known as a wake) that in turn can result in a decrease of the power output on any other downstream-placed turbine that encounters its trajectory. Especially during the last decade, it has been shown that the optimization of the turbine positioning (layout) in a wind farm can be efficiently used to minimize the wake effects and, therefore, maximize the overall wind power production of the overall wind farm (see review from Herbert-Acero et al. 2014 and references therein). More specifically, in last years it has been shown that non-deterministic methods (especially Genetic Algorithms, and to a lesser extent also others as Particle Swarm Optimization or Ant Colony Optimization) are able to reach a higher level of evolved optima compared to gradient-based techniques. Recently, a few works have applied wind farm layout optimization (WFLO) to a realistic framework, with a high enough wind flow climatology resolution and removing any grid that constraints the turbines positioning to a (few) hundreds of possible positions. At wiRE we have worked in this line (Kirchner-Bossi and Porté-Agel, 2018), by considering the Gaussian model (Bastankhah and Porté-Agel, 2014, Niayifar and Porté-Agel, 2016) for the first time as the analytical wake model for realistic WFLO. This wake model has been shown to be more accurate compared to any previously used model (e.g., Jensen or Frandsen models). In addition, the WFLO ad-hoc genetic algorithm-based methodology developed in Kirchner-Bossi and Porté-Agel (2018) provided a bigger improvement of the wind farm overall power output compared to any other so called ‘realistic WFLO’ works in the literature (in these cases performed under the Jensen model for a fair comparison).
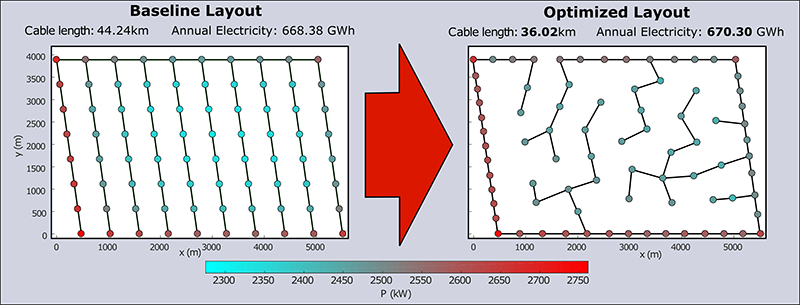
In addition to the positioning optimization itself, further WFLO research includes other elements for its analysis and optimization (e.g., Chowdhury et al., 2012). From these, the optimization of the wind farm shape, the power density, the minimum cable length or the type of turbine stand out, all this with the possibility to add other features as considering a variable height for the turbines, or adopting new cost functions as the Levelized Cost of Energy (LCOE), among others. In this way, at WiRE we have also investigated the wind farm layout optimization using mutli-rotor wind turbines, for different power densities scenarios (Kirchner-Bossi and Porté-Agel (2020).
Finally, the possibility to optimize two or more wind farm features (or cost functions) at a time allows providing as a result a wide range of optimal possibilities, obtained from the combination of the optimized functions involved. This type of optimization, called Multi-Objective Optimization, allows the decision makers (e.g., investors, governments, etc.) to select from the range of solutions the one which fits most to their needs or financial conditions. Some preliminary progress has been carried out at WiRE regarding these questions and methods. For example, a preliminary multi-objective WFLO study is currently being carried out that considers the maximization of the power output at the time that searches the minimization of the electricity cable length, two variables that are antagonistic. In addition, all this is done by granting an unconstrained area shape to the problem, an approach that has shown to remarkably increase the optimization performance compared to a fixed area wind farm optimization.
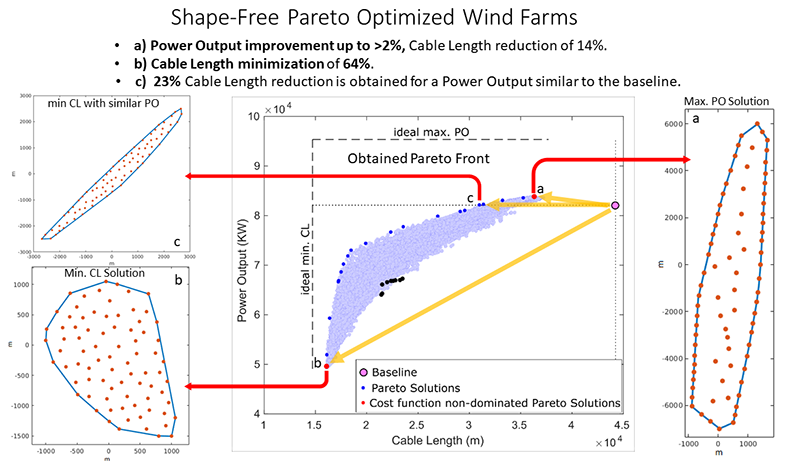
References
- Bastankhah, M., and Porté-Agel, F. (2014). A new analytical model for wind-turbine wakes. Renewable Energy, 70, 116–123.
- Chowdhury, S., Zhang, J., Messac, A., Castillo, L. (2012). Unrestricted wind farm layout optimization (UWFLO): Investigating key factors influencing the maximum power generation. Renew. Energy, 38, 16–30.
- Herbert-Acero, J.F., Probst, O., Réthoré, P.E., Larsen, G.C., Castillo-Villar, K.K. (2014) A review of methodological approaches for the design and optimization of wind farms. Energies, 7, 6930–7016.
- Kirchner-Bossi, N., and Porté-Agel, F. (2018). Realistic Wind Farm Layout Optimization through Genetic Algorithms Using a Gaussian Wake Model. Energies, 11(12), 3268.
- Kirchner-Bossi, N., & Porté-Agel, F. (2020, September). Multi-rotor Wind Farm Layout Optimization. In Journal of Physics: Conference Series (Vol. 1618, No. 3, p. 032014). IOP Publishing.
- Niayifar, A., & Porté-Agel, F. (2016). Analytical modeling of wind farms: A new approach for power prediction. Energies, 9(9), 741.