Short-Term Wind Power Forecast
Short-term (<1 week) wind power forecasting is one of the most relevant tasks in wind power industry (e.g., Soman et al., 2010). This responds to the fact that multiple applications are highly sensitive to the accuracy of the wind power production estimate within that prediction horizon. One of the most important is related to the need for any power producer to provide to the electricity market regulator an accurate prediction (against a potential fine) of the power that will be able to turn over the electricity network in the following 12h-48h. Other applications rely on the capacity to predict calms that optimize the arrangement of maintenance and operation to the turbines, or other more technical applications related to online/offline generator decision, operational security, load variation decision, etc.
Relying on the prediction strategy adopted, up to four types of short-term power forecast models can be enumerated (adopted from Chang, 2014), namely:
- Physical models, that consist on the numerical modelling of the atmospheric dynamics (NWP, LES, RANS, etc),
- Statistical models (MOS, ARMA, ARiMA, etc.), that extract the statistical inference inside the time series of the previous power data itself (spectral density, self-correlation, etc).
- Machine-Learning (ML) models (ANN, Fuzzy Logic, Support Vector Machines, etc), that are capable to manage a big amount of previous wind power information and combine it with time series from other variables and sources.
- Hybrid models, when the variables that the ML tool combines with the previous power output series consists of meteorological data from a physical model.
In recent years, hybrid models have gained popularity against statistical or physical models traditionally used (Okumus and Dinler, 2016), due to the improvement of the ML techniques and the computational capacity, especially regarding GPU’s. At the WiRE laboratory, we are developing numerical models (e.g., Tabas et al, 2019) towards more accurate wind power forecasting. Among the various techniques, we focus on multiscale coupling and machine learning. The former can better handle the multi-scale nature of the problem by simulating the physical processes at different scales in a coupled way, whereas the latter is capable of finding the complex nonlinear relationship between inputs and outputs. Moreover, the hybridization of the two techniques has the potential to yield more accurate results. The developed models are being tested at the JUVENT wind farm which is the largest wind farm in Switzerland with 16 wind turbines located in the Jura mountains (Switzerland). The site features the combined presence of three complexities: topography, heterogeneous vegetation including forest, and interactions between wind turbine wakes. Hence, it allows a comprehensive evaluation of the model performances.
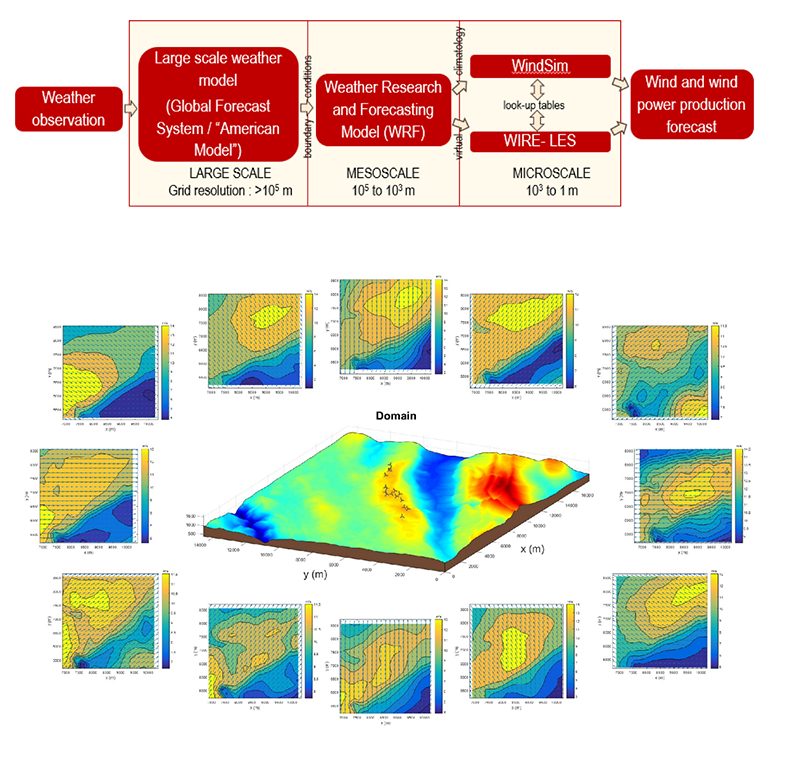
Our main research questions to this regard can be enumerated as follows:
- To develop a wind power nonlinear multi-scale model combined with Machine Learning that results more efficient than linear models in a site in complex terrain.
- To study whether considering models with predictor-expanded search spaces can result in an efficient strategy compared to the traditionally used approach limited to a few wind-related predictors in a specific location.
- To develop a wind power forecast model that represents successfully the effect of icing on the turbines blades, thus being able to overcome a caveat that is currently very extended in the wind power industry.
References:
- Chang, W. Y. (2014). A literature review of wind forecasting methods. Journal of Power and Energy Engineering, 2(4).
- Okumus, I., and Dinler, A. (2016). Current status of wind energy forecasting and a hybrid method for hourly predictions. Energy Conversion and Management, 123, 362-371.
- Soman, S. S., Zareipour, H., Malik, O., and Mandal, P. (2010). A review of wind power and wind speed forecasting methods with different time horizons. In North American Power Symposium 2010 (pp. 1-8). IEEE.
- Tabas, D., Fang, J., and Porté-Agel, F. (2019) Wind Energy Prediction in Highly Complex Terrain by Computational Fluid Dynamics. Energies7: 1311.