|
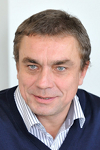 |
|
|
The Transport and Mobility Laboratory, directed by Michel Bierlaire, is active in operations research and optimization, with a particular emphasis on transportation systems. We are particularly interested in introducing mathematical models of behavior in optimization frameworks.
|
|
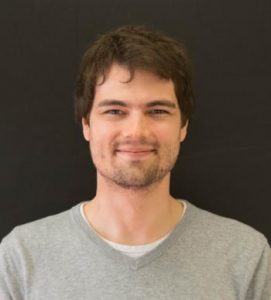 |
|
|
In the Chair of Continuous Optimization (OPTIM), we study the theory and applications of optimization. Our current focus is on geometry and non-convexity. Applications of interest connect with computational sciences, machine learning, statistics and robotics.
|
|
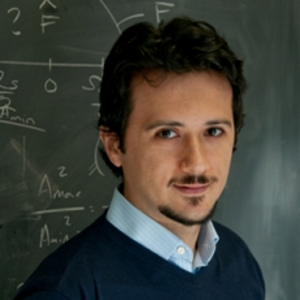 |
|
|
At LIONS, we develop theory and algorithms for machine learning, signal processing, and automatic control.
|
|
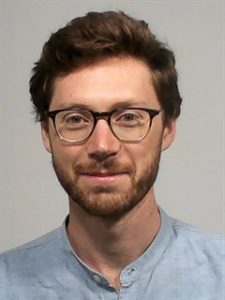 |
|
|
At DOLA, our goal is to understand and develop the algorithms used for machine learning problems, and in particular those with many “parameters”: training a neural network with back-propagation, searching saddle points for adversarial training, learning a non-parametric generative model, etc. We are often interested in the “infinite overparameterization limit” which enable the use of tools from mathematical analysis (such as the mean-field theory from mathematics physics) and leads to qualitative insights, quantitative guarantees and practical recommendations.
|
|
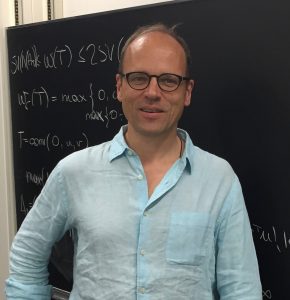 |
|
|
Discrete optimization problems are ubiquitous. Finding an optimal way to route information through a network or determining an energy-efficient way to display an image are just two examples from everyday life. Our research focuses on the design and analysis of algorithms for discrete optimization problems.
|
|
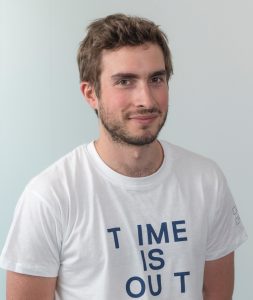 |
|
|
At TML (Theory of Machine Learning), we develop algorithmic and theoretical tools to better understand machine learning and to make it more robust and usable.
|
|
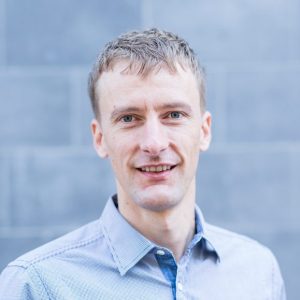 |
|
|
The MLO lab (Machine Learning and Optimization) studies optimization for deep learning, as well as distributed and collaborative machine learning systems.
|
|
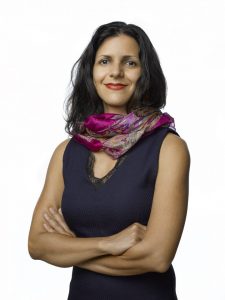 |
|
|
Our focus is on advancing fundamental understanding of multi-agent decision-making in uncertain and dynamic environments. Towards this vision, we develop game theory, distributed control, stochastic and data-driven safe control. Our theoretical work is motivated by applications ranging from transportation and power grid systems to rescue robotics.
|
|
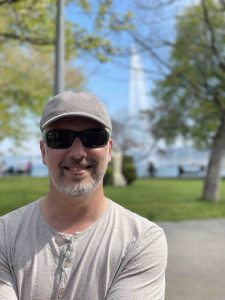 |
|
|
I am interested in high-dimensional problems, with motivations coming from machine learning, signal processing or combinatorics, and in particular to phase transitions in optimisation and sampling algorithms.
|
|
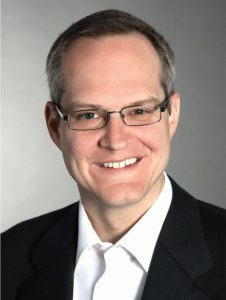 |
|
|
Daniel Kuhn is the director of the Risk Analytics and Optimization (RAO) lab. Research at RAO addresses the theoretical foundations and applications of optimization under uncertainty with a special focus on and data-driven optimization as well as stochastic, robust, and distributionally robust optimization. Application areas range from statistics and machine learning to business analytics, risk management and engineering.
|
|
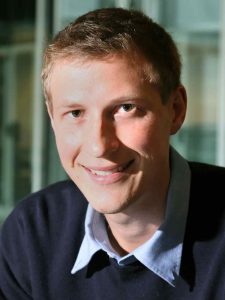 |
|
|
My main interest is in using polyhedral techniques for combinatorial optimization. In particular, I am interested in giving algorithms using these techniques and proving their optimality.
|
|
 |
|
|
I am interested in combinatorial optimization on random structures, and continuous optimization in random non-convex landscapes, with motivations coming from the theory of neural networks, inverse problems in signal processing or combinatorics.
|
Should your group be added to this list? Let us know.