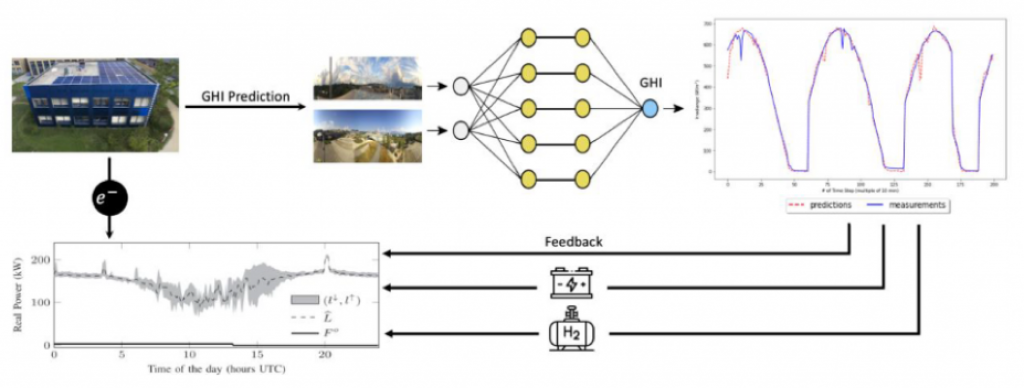
Contribute to optimize energy storage systems on EPFL campus coming from electricity generated by photovoltaic panels and improve tracking of the electricity dispatch plan by developing accurate solar irradiance predictions.
The EPFL smart grid demonstrator has been extensively used to develop, and experimentally validate, the concept of Active Distribution Network dispatchability. Network load imbalances lead to deviations in the dispatch plan, which in turn results in financial penalty charges. These charges are in the order of 500K to 2 Mill CHF per GWh for purchases 2% above or below the submitted dispatch.
The electricity dispatch plan relies, among other, on the availability of high-performance solar irradiance forecasting tools at day-ahead and intra-day time horizon. The need to forecast in different time horizons depends on the use case of PV forecasting such as better scheduling and dispatching of energy, storage or selling planning and reserve activation.
EPFL has the means to better predict its on-campus production of PV power to more accurately follow the dispatch plan set the day before.
We will use artificial intelligence in the form of a digital neural network which will be trained on a dataset of publicly available images collected from webcams on EPFL campus and surrounding areas, combined with data from traditional weather forecasting techniques. This novel approach combines long short-term memory with a convolutional neural network (LSTM-CNN) to predict intra-day solar irradiance values in the 2-4 hours range.
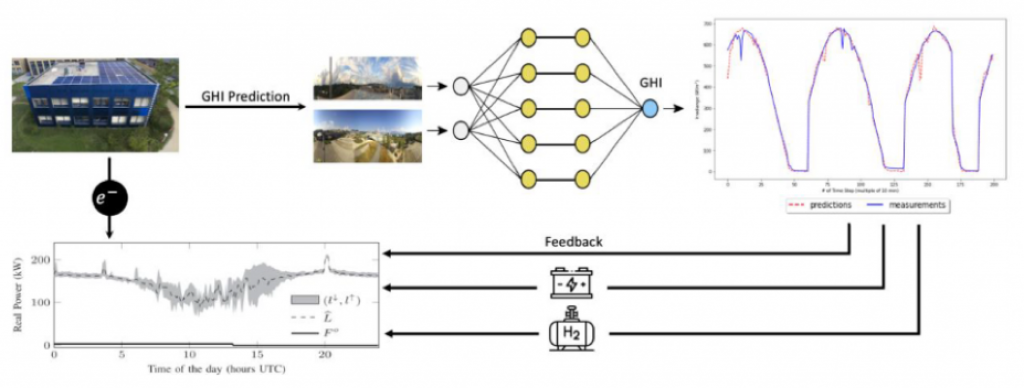
Forecasting the solar irradiance (GHI) will lead to the accurate prediction of the renewable energy production and will allow EPFL to appropriately select the size and kind of the energy storage systems.
A decision-making tool, based on an economic model that incorporates short- and long-term storage technological constraints and costs, current wholesale energy prices and an estimate of the penalty charges, to determine the strategy to be implemented to match the dispatch plan. The H2elios NeuroCam technology has the potential to optimize the use of energy sources at the national level.
Prof. Christophe MOSER, Laboratory of Photonics Devices, https://www.epfl.ch/labs/lapd/
Prof. Luisa LAMBERTINI, Chair of International Finance, https://www.epfl.ch/labs/cfi/
Prof. Mario PAOLONE, Distributed Electrical Systems Laboratory – Power Systems group, https://www.epfl.ch/labs/desl-pwrs/
Prof. Demetri PSALTIS, Optics Laboratory, https://www.epfl.ch/labs/lo/
Public webcams for forecasting sun irradiance:
The project requires to use webcams located on Place Cosandey and the roof of BC building. The 360 cameras record images which could contain personal data such as persons or cars visible on them. To ensure the privacy of such personal data, the images are blurred using OpenCV’s YOLO v3 model. An example of a blurred image is shown here. Live recordings of the cameras may be seen here and here. The blurred images are processed by a digital neural network to forecast solar irradiation. These images will not be published and will be deleted at the end of the thesis of the PhD students involved in the project.
Data management:
Data will be collected daily from December 2021 to end 2026, at a frequency of 10 minutes. By law, EPFL may collect and use personal data for research purposes (art. 36c of the Federal Law on the Federal Institutes of Technology of 4 October 1991), and we rely on this legal basis to legitimize our processing of personal data.
The storage plan for this project will be implemented using a Network Attached Storage (NAS) system at EPFL, a service provided internally by the Research Computing Platform. The data will be backed up on an S3 Bucket. Access rights will be controlled through the EPFL identity management system, ensuring only project members can access the stored data.
More detailed information and project-related news can be found at the following address:
https://www.epfl.ch/labs/lapd/research/s4s-project-h2elios-neurocams/