Detecting critical events in a trustable way with explainable artificial intelligence
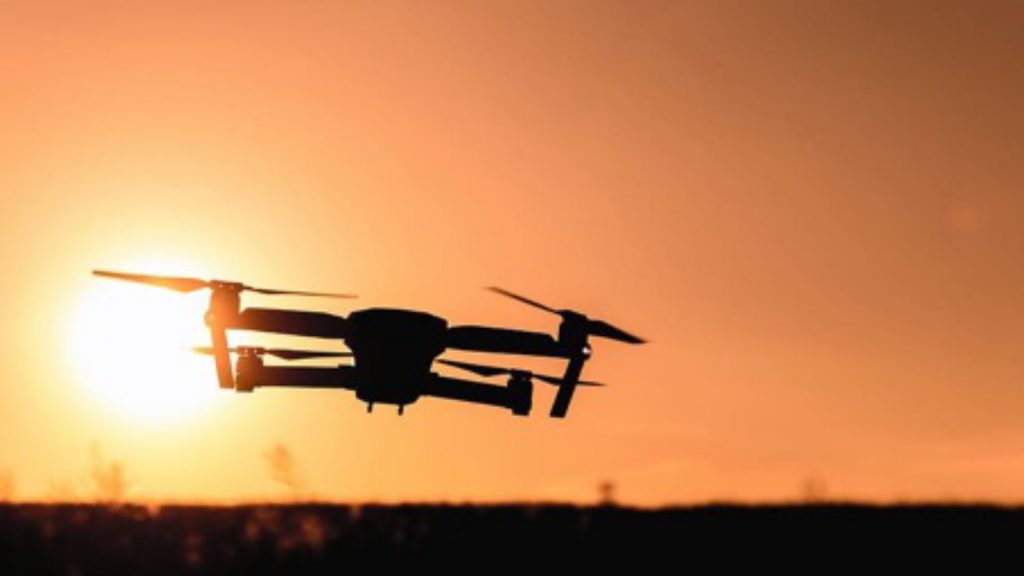
Summary
Critical and rare events have a major impact on society. Made more probable by aging infrastructure and environmental and climatic changes, we witness more and more unusual events, which are very difficult to predict by usual modeling tools. In particular, machine learning algorithms are being more and more used as predictive tools in many areas of environmental and civil engineering, but the ability of a learning algorithm to predict the unexpected is hampered by the lack of examples available to train such models. This is a major issue, since machine learning algorithms are, by definition, data hungry and need annotated examples to learn. Moreover, even if predicting correctly, these models are of little use for decision making, since they cannot explain the rationale behind predictions, nor providing robust uncertainty estimates. Driven by these major flaws of current learning models, the IMOS and ECEO laboratories of ENAC join forces to study the problem of detecting rare events related to railway networks, and tackle the detection of infrastructure conditions deterioration and of risks related to sudden land cover changes. The explAIn project makes a significant leap forward toward systems that can predict and explain, therefore setting the basis for a new generation of machine learning models for environmental sciences and civil engineering.
General information
- PIs: Devis Tuia (ECEO / IIE) and Olga Fink (IMOS / IIC)
- Team members: Florent Forest, Hugo Porta
- Start date: 01.09.2022
- Duration: 24 months